Acoustic-Based Machine Anomaly Detection Using Beamforming and Sequential Transform Learning
IEEE Sensors Letters(2023)
摘要
Acoustic-based analysis has been widely used for the maintenance and operation of industrial machines. However, interferences and background noise highly contaminate the observed acoustic signal. Here, we present a novel two-stage multichannel source separation technique for improved separation and robust anomaly detection. Beamforming is applied in the first stage to provide separation at a coarser level. Sequential transform learning is employed in the second stage to learn the dynamics of the time-varying source signal for more refined source separation. The separated machine sounds are analyzed for anomaly using a simple template matching approach. Results obtained using the MIMII dataset indicate that the proposed two-stage method provides an average improvement of 1.98 dB in signal-to-noise ratio and 19.75
$\%$
in accuracy when compared to the best-performing state-of-the-art methods for source separation and anomaly detection, respectively.
更多查看译文
关键词
Sensor signal processing,signal applications,anomaly detection (AD),beamforming,multichannel,sequential transform learning,source separation (SS)
AI 理解论文
溯源树
样例
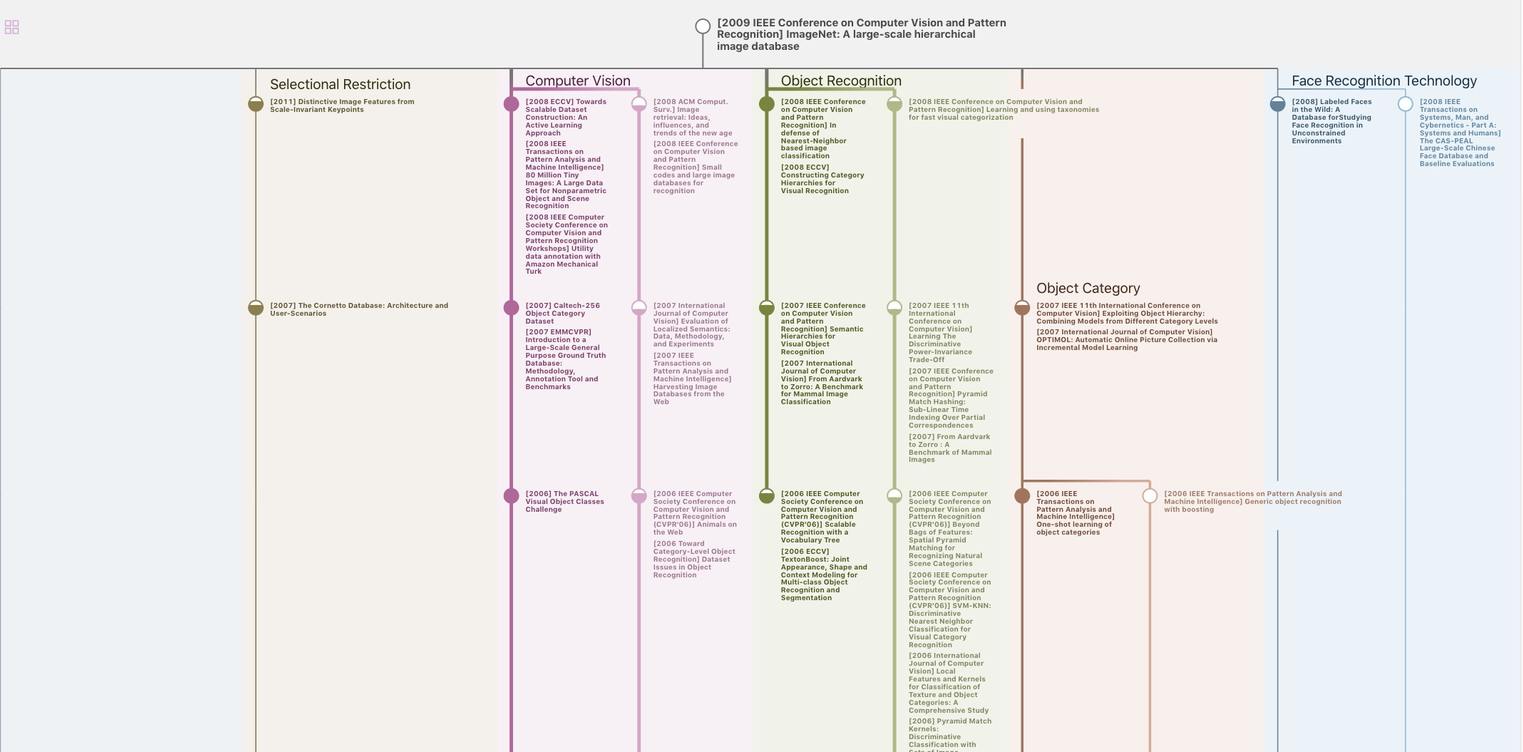
生成溯源树,研究论文发展脉络
Chat Paper
正在生成论文摘要