ImmuneMirror: a Machine Learning-based Integrative Pipeline and Web Server for Neoantigen Prediction
biorxiv(2023)
摘要
Neoantigens are derived from tumors but are absent in normal tissues. Emerging evidence suggests that neoantigens can stimulate tumor-specific T-cell-mediated antitumor immune responses, and neoantigens are potential immunotherapy targets. We developed ImmuneMirror as a stand-alone open-source pipeline ( ) and a web server ( ) incorporating a balanced random forest model for neoantigen prediction and prioritization; the model was trained and tested using known immunogenic neopeptides collected from 19 published studies. The area under the curve (AUC) of our model was 0.87. We utilized ImmuneMirror in gastrointestinal tract cancers and discovered a subgroup of microsatellite instability-high (MSI-H) colorectal cancer (CRC) patients with a low neoantigen load but a high tumor mutation burden (TMB>10 mutations per Mbp). Although the efficacy of PD-1 blockade has been demonstrated in advanced MSI-H patients, almost half of such patients do not respond well. Our study may identify MSI-H patients who do not benefit from this treatment. Additionally, the neopeptide YMCNSSCMGV-TP53G245V, derived from a hotspot mutation restricted by HLA-A02, was identified as an actionable target in esophageal squamous cell carcinoma (ESCC). This is the largest study to comprehensively evaluate neoantigen prediction models using experimentally validated neopeptides. Our results demonstrate the reliability and effectiveness of ImmuneMirror for neoantigen prediction.
### Competing Interest Statement
The authors have declared no competing interest.
更多查看译文
关键词
neoantigen prediction,machine learning,multiomics,gastrointestinal tract cancer,immunotherapy,computational platform
AI 理解论文
溯源树
样例
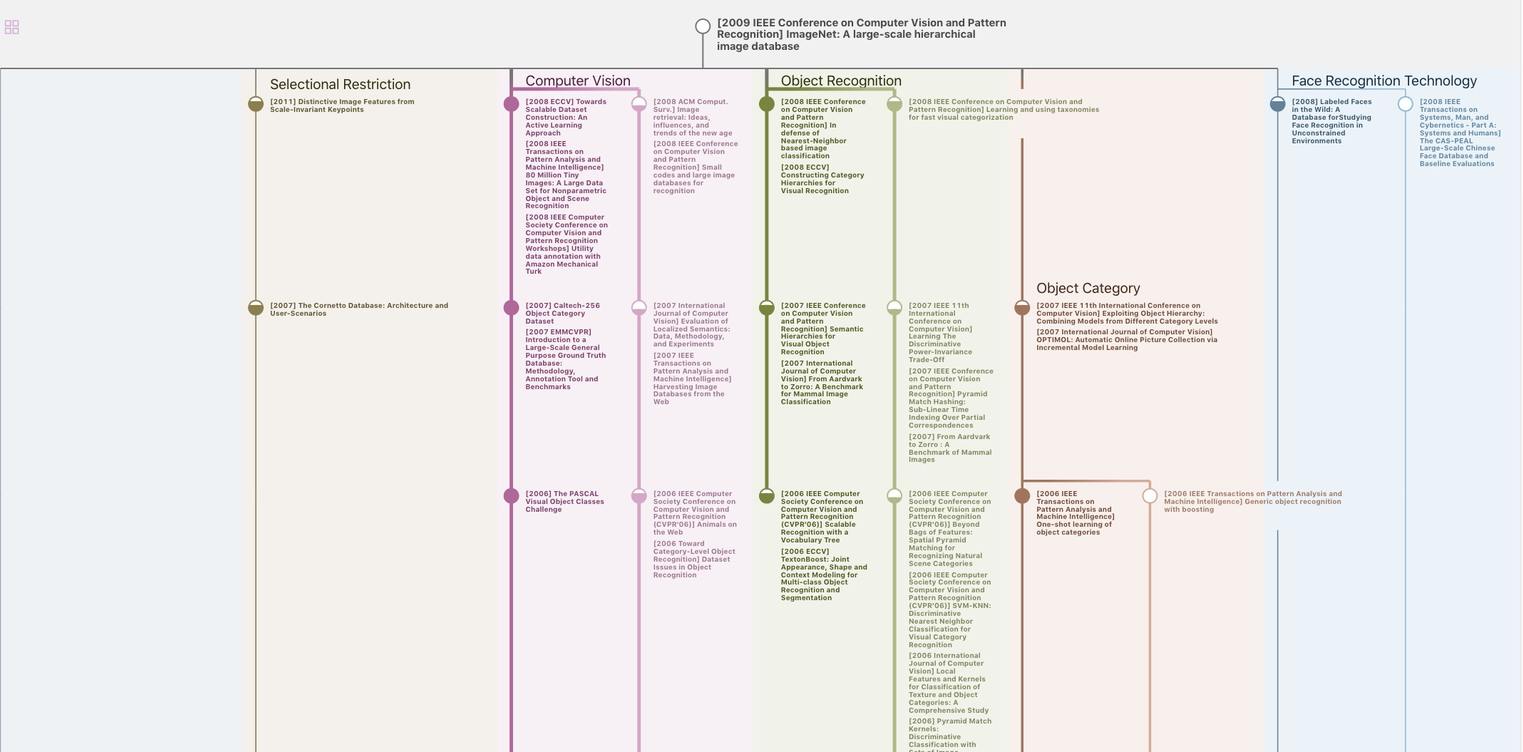
生成溯源树,研究论文发展脉络
Chat Paper
正在生成论文摘要