Genotypic-phenotypic landscape computation based on first principle and deep learning
Briefings in Bioinformatics(2024)
摘要
The relationship between genotype and fitness is fundamental to evolution, but quantitatively mapping genotypes to fitness has remained challenging. We propose the Phenotypic-Embedding theorem (P-E theorem) that bridges genotype-phenotype through an encoder-decoder deep learning framework. Inspired by this, we proposed a more general first principle for correlating genotype-phenotype, and the Phenotypic-Embedding theorem provides a computable basis for the application of first principle. As an application example of the P-E theorem, we developed the Co-attention based Transformer model to bridge Genotype and Fitness (CoT2G-F) model, a Transformer-based pre-train foundation model with downstream supervised fine-tuning (SFT) that can accurately simulate the neutral evolution of viruses and predict immune escape mutations. Accordingly, following the calculation path of the P-E theorem, we accurately obtained the basic reproduction number ( R 0 ) of SARS-CoV-2 from first principles, quantitatively linked immune escape to viral fitness, and plotted the genotype-fitness landscape. The theoretical system we established provides a general and interpretable method to construct genotype-phenotype landscapes, providing a new paradigm for studying theoretical and computational biology.
### Competing Interest Statement
The authors have declared no competing interest.
更多查看译文
AI 理解论文
溯源树
样例
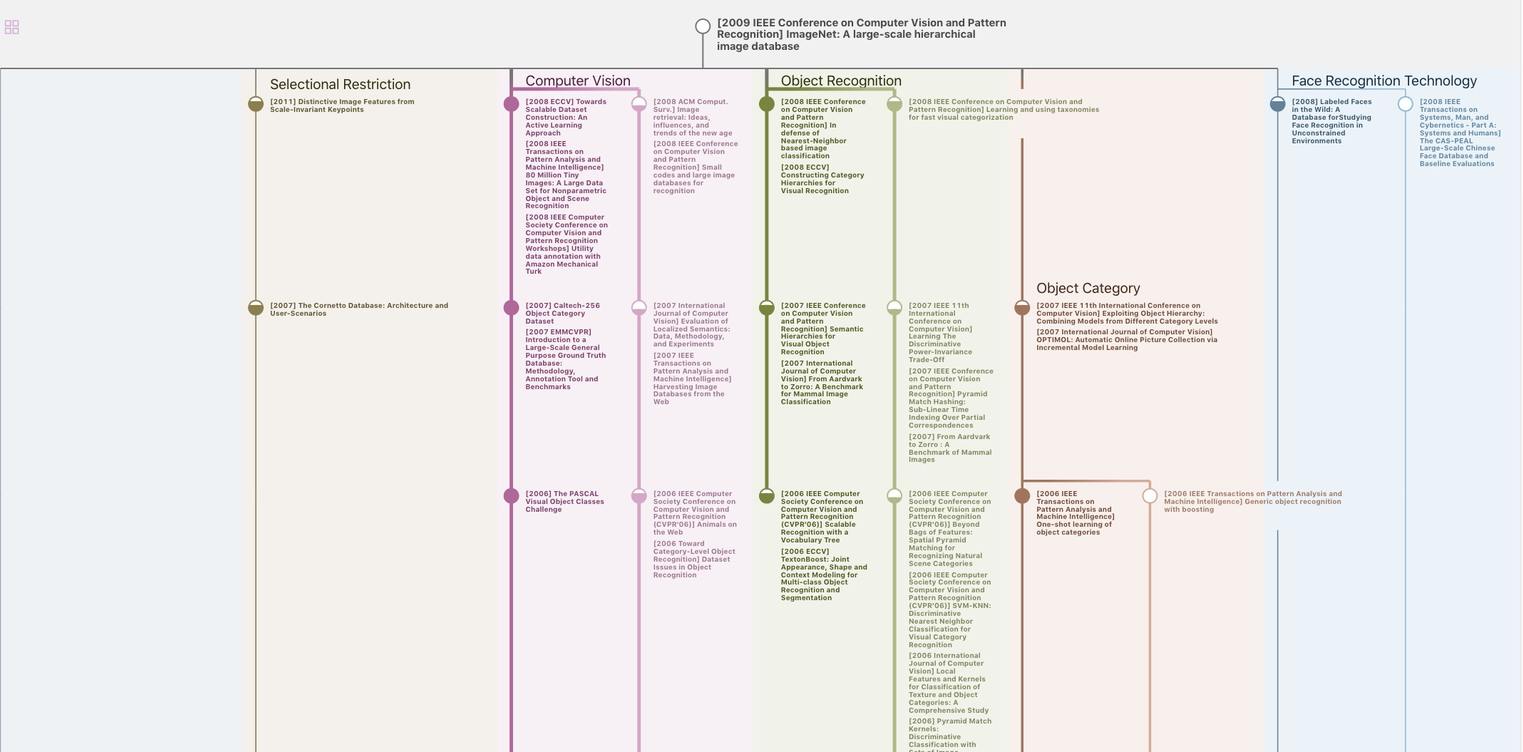
生成溯源树,研究论文发展脉络
Chat Paper
正在生成论文摘要