Uncertainty-Aware Variational Inference for Target Tracking
IEEE Transactions on Aerospace and Electronic Systems(2023)
摘要
In the low Earth orbit, target tracking with ground based assets in the context of situational awareness is particularly difficult. Because of the nonlinear state propagation between the moments of measurement arrivals, the inevitably accumulated errors will make the target state prediction and the measurement likelihood inaccurate and uncertain. In this article, optimizable models with learned parameters are constructed to model the state and measurement prediction uncertainties. A closed-loop variational iterative framework is proposed to jointly achieve parameter inference and state estimation, which comprises an uncertainty-aware variational filter (UnAVF). The theoretical expression of the evidence lower bound and the maximization of the variational lower bound are derived without the need for the true states, which reflect the awareness and reduction of uncertainties. The evidence lower bound can also evaluate the estimation performance of other Gaussian density filters, not only the UnAVF. Moreover, two rules, estimation consistency and lower bound consistency, are proposed to conduct the initialization of hyperparameters. Finally, the superior performance of UnAVF is demonstrated over an orbit state estimation problem.
更多查看译文
关键词
Dynamic system,Kalman filter,nonlinear filter,variational inference (VI)
AI 理解论文
溯源树
样例
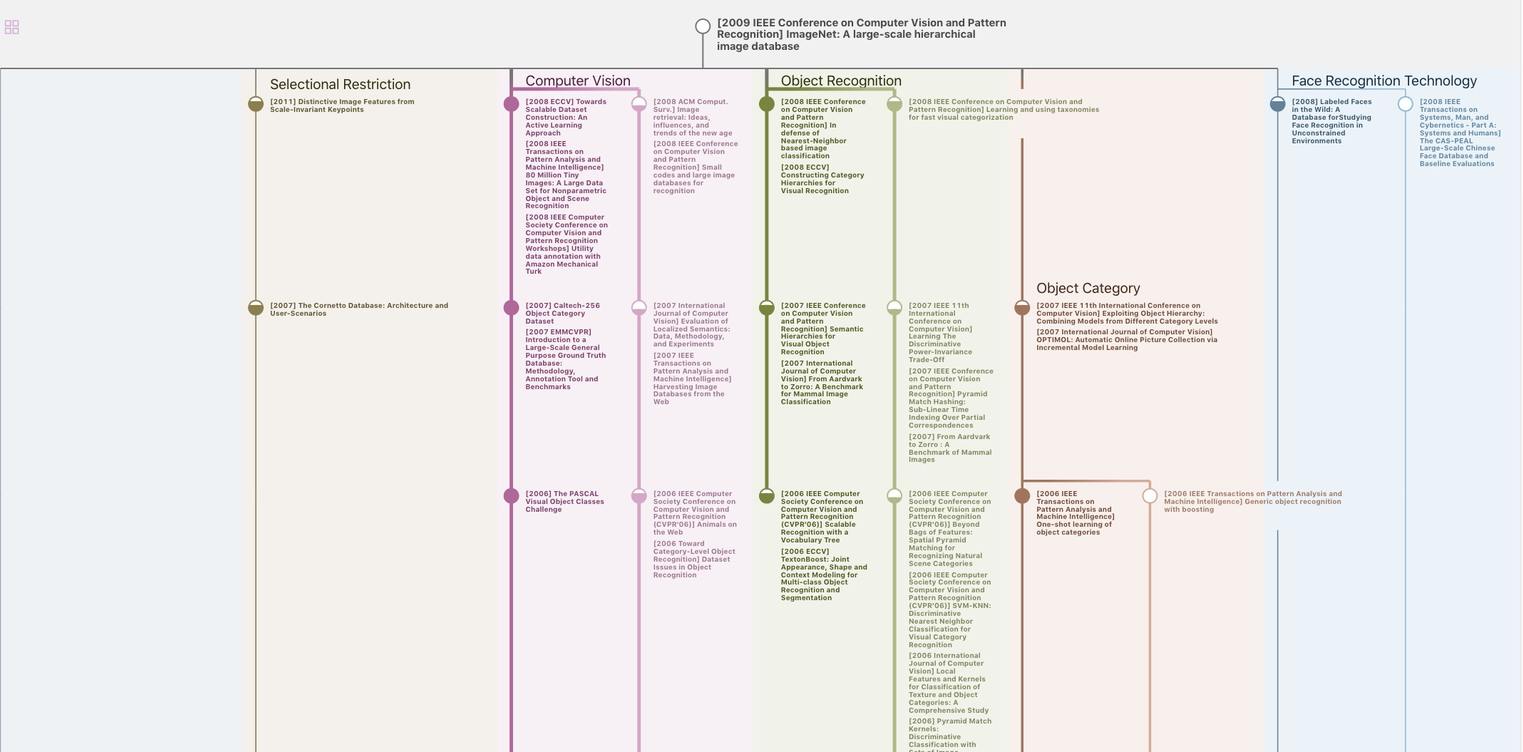
生成溯源树,研究论文发展脉络
Chat Paper
正在生成论文摘要