Deep intra-image contrastive learning for weakly supervised one-step person search
PATTERN RECOGNITION(2024)
摘要
Weakly supervised person search aims to perform joint pedestrian detection and re-identification (re-id) with only bounding-box annotations. Recently, the idea of contrastive learning is initially applied to weakly supervised person search, where two common contrast strategies are memory-based contrast and intra-image contrast. We argue that current intra-image contrast is shallow, which suffers from spatial-level and occlusionlevel variance. In this paper, we present a novel deep intra-image contrastive learning using a Siamese network. Two key modules are spatial-invariant contrast (SIC) and occlusion-invariant contrast (OIC). SIC performs many-to-one contrasts between two branches of Siamese network and dense prediction contrasts in one branch of Siamese network. With these many-to-one and dense contrasts, SIC tends to learn discriminative scaleinvariant and location-invariant features to solve spatial-level variance. OIC enhances feature consistency with the masking strategy to learn occlusion-invariant features. Extensive experiments are performed on two person search datasets. Our method achieves a state-of-the-art performance among weakly supervised one -step person search approaches.
更多查看译文
关键词
Person search,Weakly supervised,Contrastive learning,Spatial-invariant,Occlusion-invariant
AI 理解论文
溯源树
样例
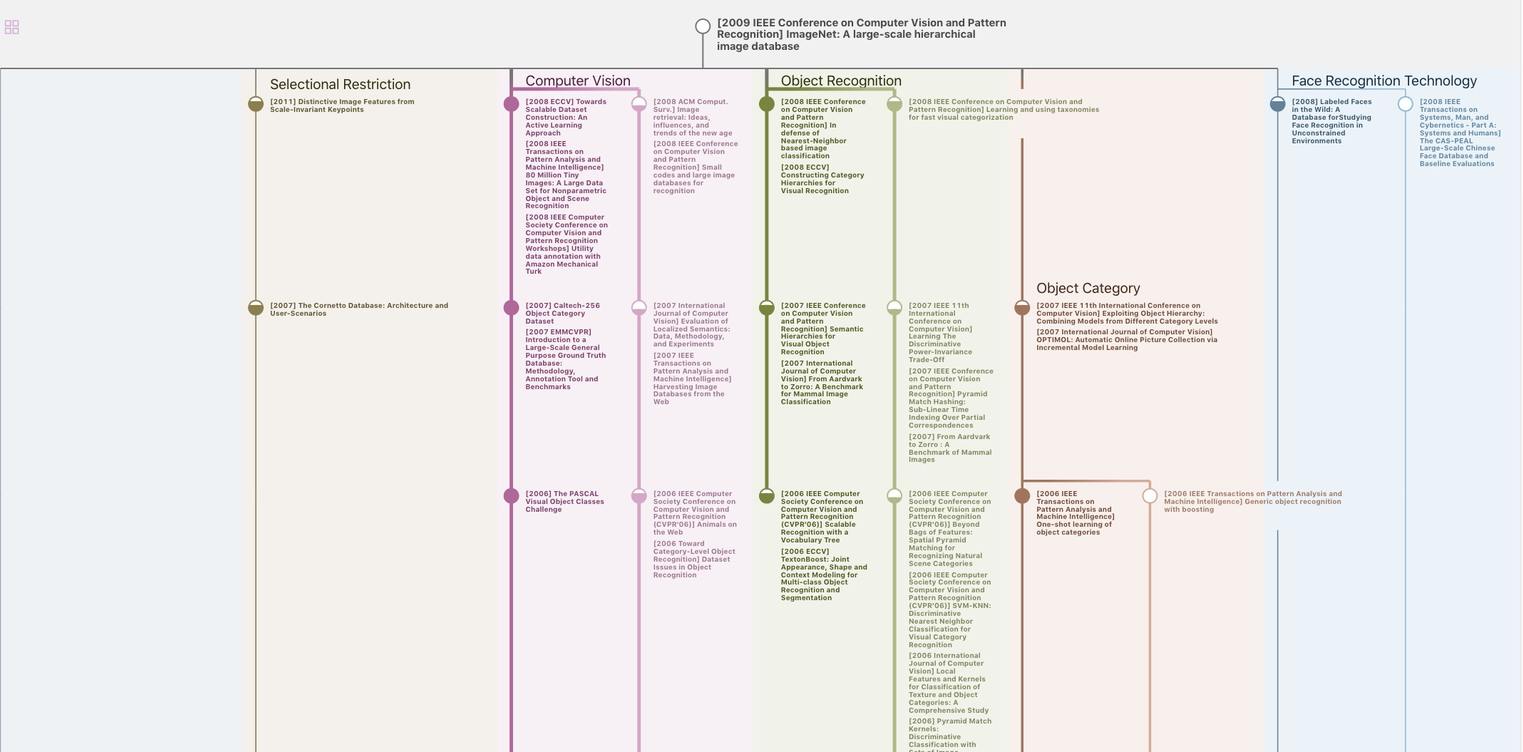
生成溯源树,研究论文发展脉络
Chat Paper
正在生成论文摘要