A Speech-Noise-Equilibrium Loss Function for Deep Learning-Based Speech Enhancement
2022 13th International Symposium on Chinese Spoken Language Processing (ISCSLP)(2022)
摘要
The deep learning (DL)-based speech enhancement (SE) has demonstrated its advantage over the classical methods. In most DL-based SEs, however, the systems are optimized based on the minimum mean squared error (MSE), which could result in poor performance in severe noise conditions, e.g., very low signal-to-noise ratios. This paper presents a speech-noise-equilibrium loss function, i.e., a weighted combination of the speech distortion and the noise residue, for network training. Furthermore, based on the observation of the non-Gaussian distribution of the prediction error, a mean absolute error (MAE) criterion is adopted for speech distortion, and hybrid training, i.e., MSE followed by MAE, is proposed for network optimization. Experiment results demonstrate that long-short term memory networks (LSTM)-based SE systems with the proposed loss function achieve better performance than the baselines, particularly, improving both speech quality and intelligibility at low signal-to-noise ratios.
更多查看译文
关键词
speech enhancement,deep learning,speech-noise-equilibrium,loss function
AI 理解论文
溯源树
样例
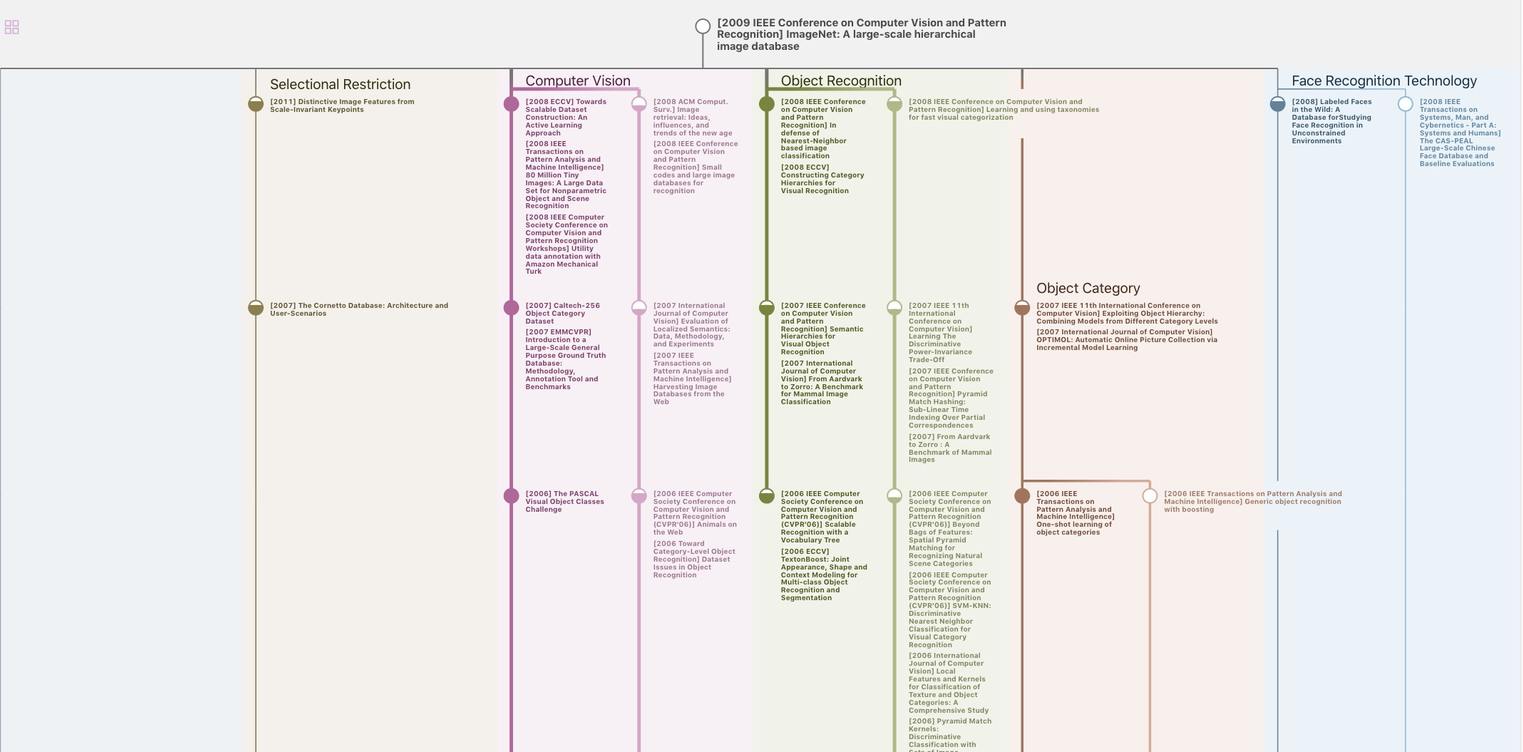
生成溯源树,研究论文发展脉络
Chat Paper
正在生成论文摘要