Shuffle is What You Need
2022 13th International Symposium on Chinese Spoken Language Processing (ISCSLP)(2022)
摘要
Self-supervised learning gains extensive attention in speaker recognition, partly due to the difficulty of collecting data with large-scale speaker labels. Contrastive learning is among the most popular approaches in this setting, where similar pairs (positive) are sampled from the same utterance while dissimilar pairs (negative) are sampled from different utterances. Despite the promising results reported in the literature, we argue that the random sampling approach may lead to unideal content residual in speaker embeddings, due to the learning of content dependency in positive pairs. In this paper, we investigate a novel frame shuffle approach, which constructs positive pairs by shuffling the frames of the anchor segment. Our experimental results on the VCTK dataset showed that the new approach can obtain comparable or better performance compared to random sampling. Moreover, the frame shuffle approach fully corrupts the linguistic content in the training data, which enforces the learned model being language independent. We tested the hypothesis in both multi-lingual and cross-lingual scenarios and observed remarkable performance improvement over the random sampling baseline.
更多查看译文
关键词
speaker recognition,self-supervised training,contrastive learning
AI 理解论文
溯源树
样例
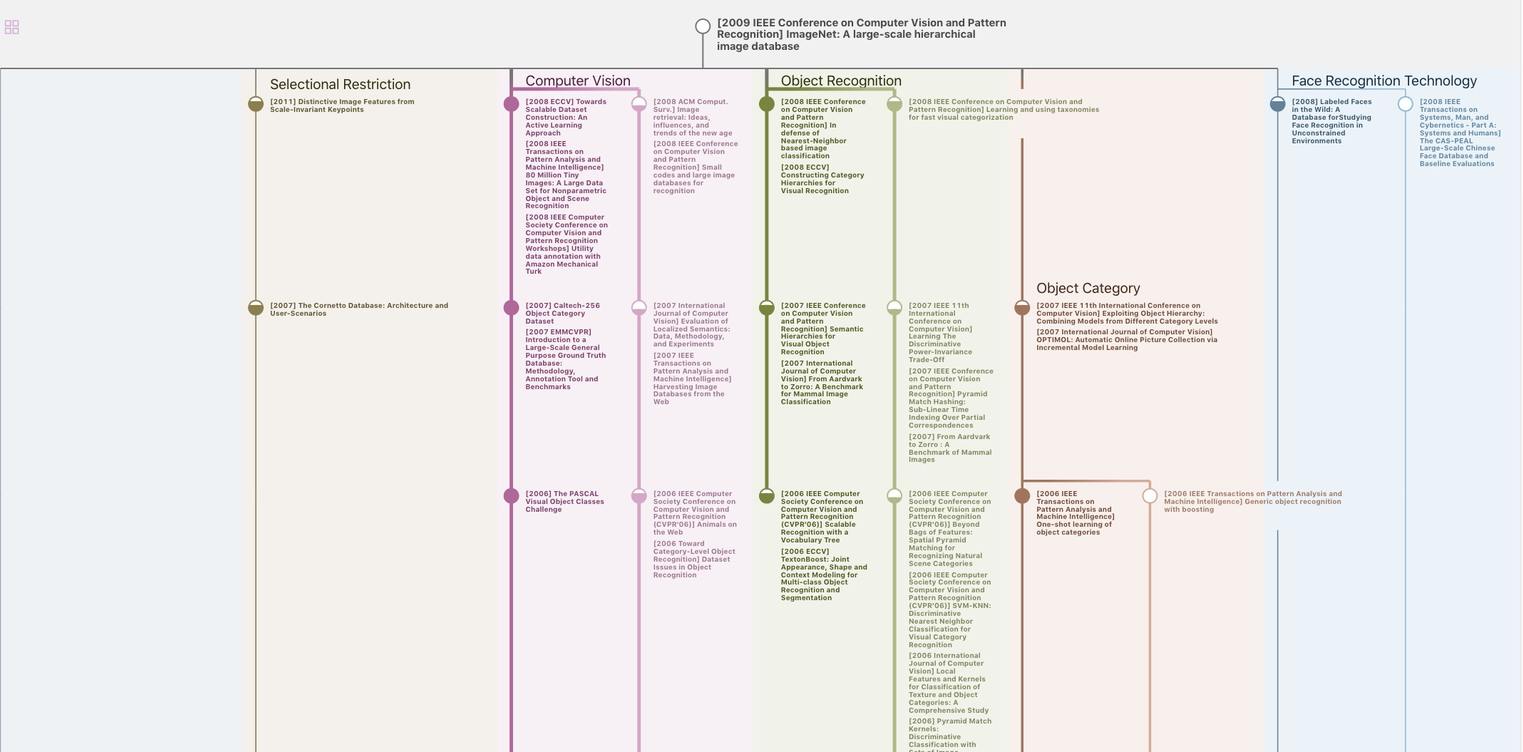
生成溯源树,研究论文发展脉络
Chat Paper
正在生成论文摘要