Image Classification Based on Deep Graph Convolutional Networks
2022 IEEE 9th International Conference on Data Science and Advanced Analytics (DSAA)(2022)
摘要
Due to their powerful modeling and reasoning capabilities, graph neural networks have not only achieved adequate performance in unstructured data but, in recent years, their research interests in Euclidean data such as images have also been on the rise. In this context, the most common task is image classification, whose method, based on graph neural networks, is roughly divided into two stages. The first is the graph construction stage, where the images are converted into graph structure data (composed of a node-edge-node form). The second is the graph classification stage, where the processed graph data is loaded into the graph classification network for graph classification. Subsequently, the images classification results are obtained. However, nearly two problems are faced in this setting. One is that the graph construction stage takes a long time due to the considerable computation that is required in order to convert images into graph structured data, the other problem is related to the graph classification used in the graph classification stage. The number of layers in the network tends to be limited, usually 4 layers or less. Hence, the graph's classification accuracy is affected to some extent. Subsequently, this study proposes a deep graph neural image classification model based on gSLIC, combining the attention mechanism to conduct related experiments, in order to prove that while the speed of graph construction is greatly improved, the image classification accuracy exceeds that of most existing models based on graph neural networks for image classification.
更多查看译文
关键词
image classification,graph convolutional networks,superpixel,simple linear iterative clustering
AI 理解论文
溯源树
样例
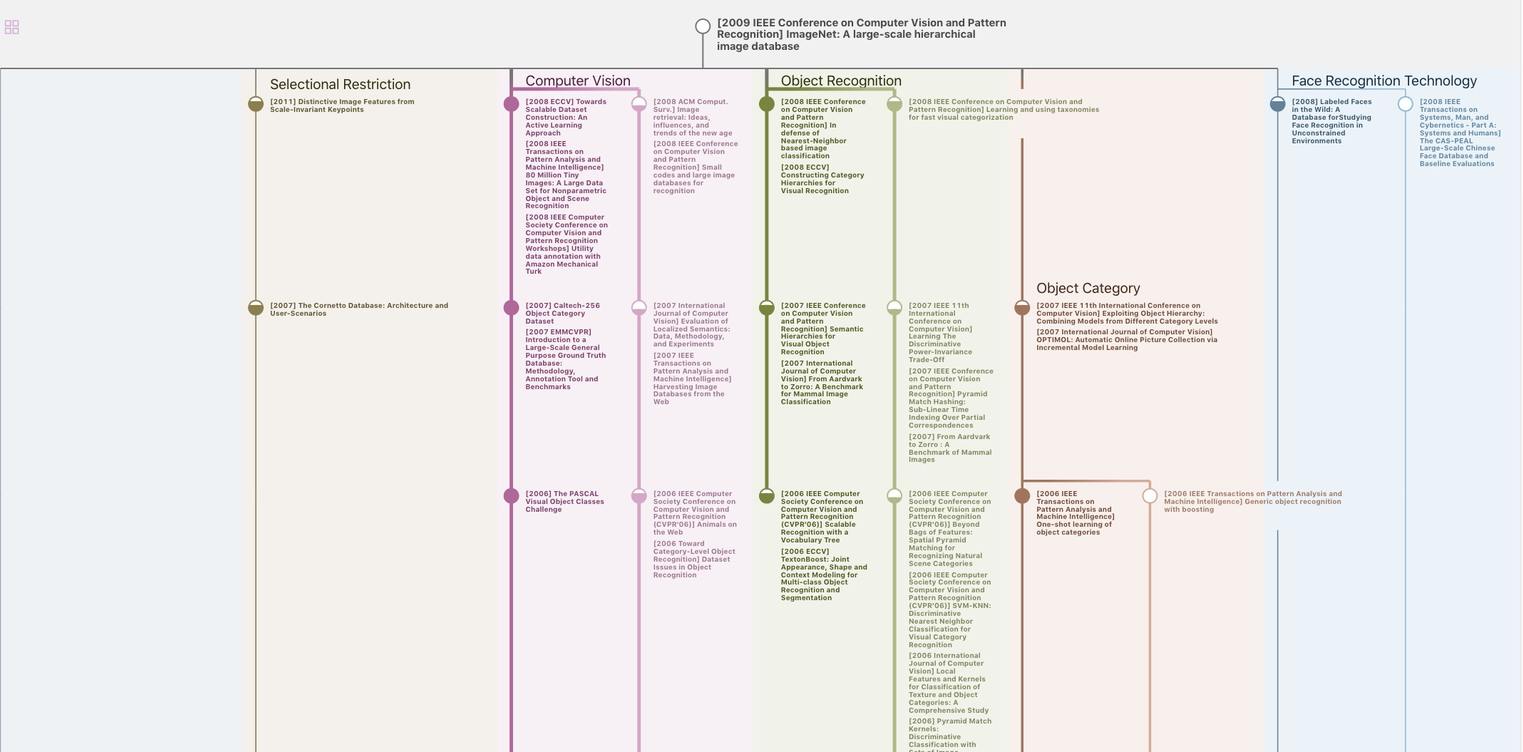
生成溯源树,研究论文发展脉络
Chat Paper
正在生成论文摘要