Multivariate Time Series Analysis: An Interpretable CNN-based Model
2022 IEEE 9th International Conference on Data Science and Advanced Analytics (DSAA)(2022)
Abstract
Deep neural networks, especially the Convolutional Neural Network (CNN) models, have shown promising results in multivariate time series data analysis. However, the predictions of these data-driven black-box models are tough to interpret from a human perspective, making it questionable to trust and rely on the predictions made by these models, specifically for time series data with the append-only feature. This paper proposes a new approach to interpret the CNN outputs by extracting and clustering the activated time series sequences learned from a trained network. These sequences show the representative features for each output label and form interpretable representations from the original time series data. Our approach is the first framework to identify each signal's role and dependencies, consider all possible combinations of signals in the multivariate time-series input, and visualize the data representative features. Our experiments on the Baydogan's archive indicate remarkable improvements in the interpretability of the network predictions and relation identification of each input signal to the output label and the channels of the network layers. Furthermore, the conducted experiments confirm that the extracted patterns are representative of the multivariate input and changing them results in a drastic reduction in the prediction accuracy.
MoreTranslated text
Key words
interpretability,neural networks,time series,classification
AI Read Science
Must-Reading Tree
Example
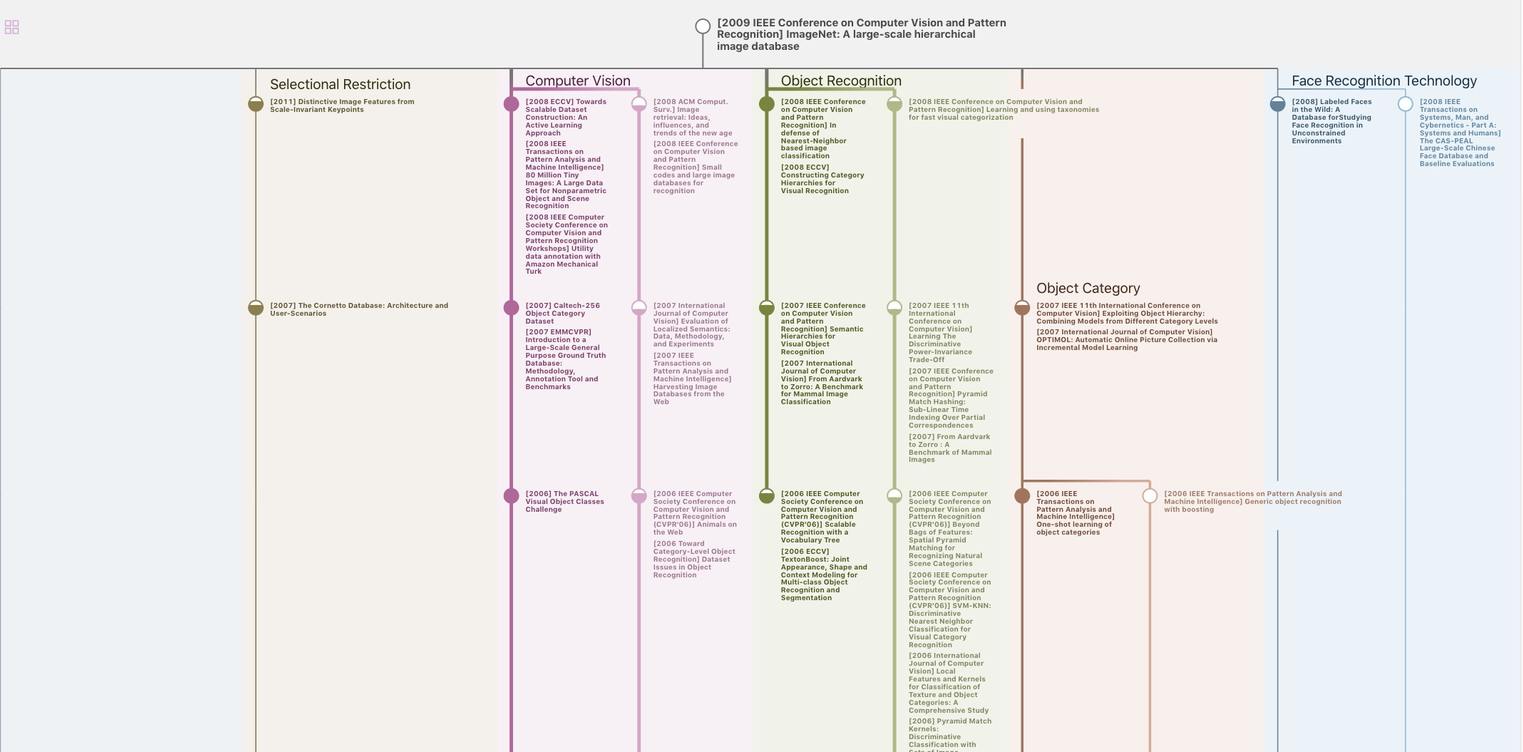
Generate MRT to find the research sequence of this paper
Chat Paper
Summary is being generated by the instructions you defined