Multi-aspect Matrix Factorization based Visualization of Convolutional Neural Networks
2022 IEEE 9th International Conference on Data Science and Advanced Analytics (DSAA)(2022)
摘要
What does the space learned by a Convolutional neural network look like? Can we automatically extract high-level concepts that concisely summarize this space in a human-understandable manner? Can we, then, use those concepts for neural network interpretability? In this work, we define a concept to be a co-cluster of data instances (e.g., images), raw features (e.g., pixels), and neuron activations per hidden layer. Such a co-clusters links human-understandable characteristics like data instances and raw features with the architectural elements like neurons of the neural network. In order to extract such multi dimensional concepts, we propose a framework based on regularized and constrained coupled matrix factorization, where the goal of regularization is to force the latent factors to correspond to the sought-after concepts. Our proposed framework is unsupervised since it only requires unlabeled data instances and their activations as an input. Through extensive qualitative and quantitative experimentation on a number of datasets and architectures we show that our proposed framework is able to extract coherent and human-understandable concepts. Finally, we demonstrate the flexibility and versatility of our proposed framework in its ability to be leveraged as an additional tool which complements the existing state-of-the-art neural network interpretability methods.
更多查看译文
AI 理解论文
溯源树
样例
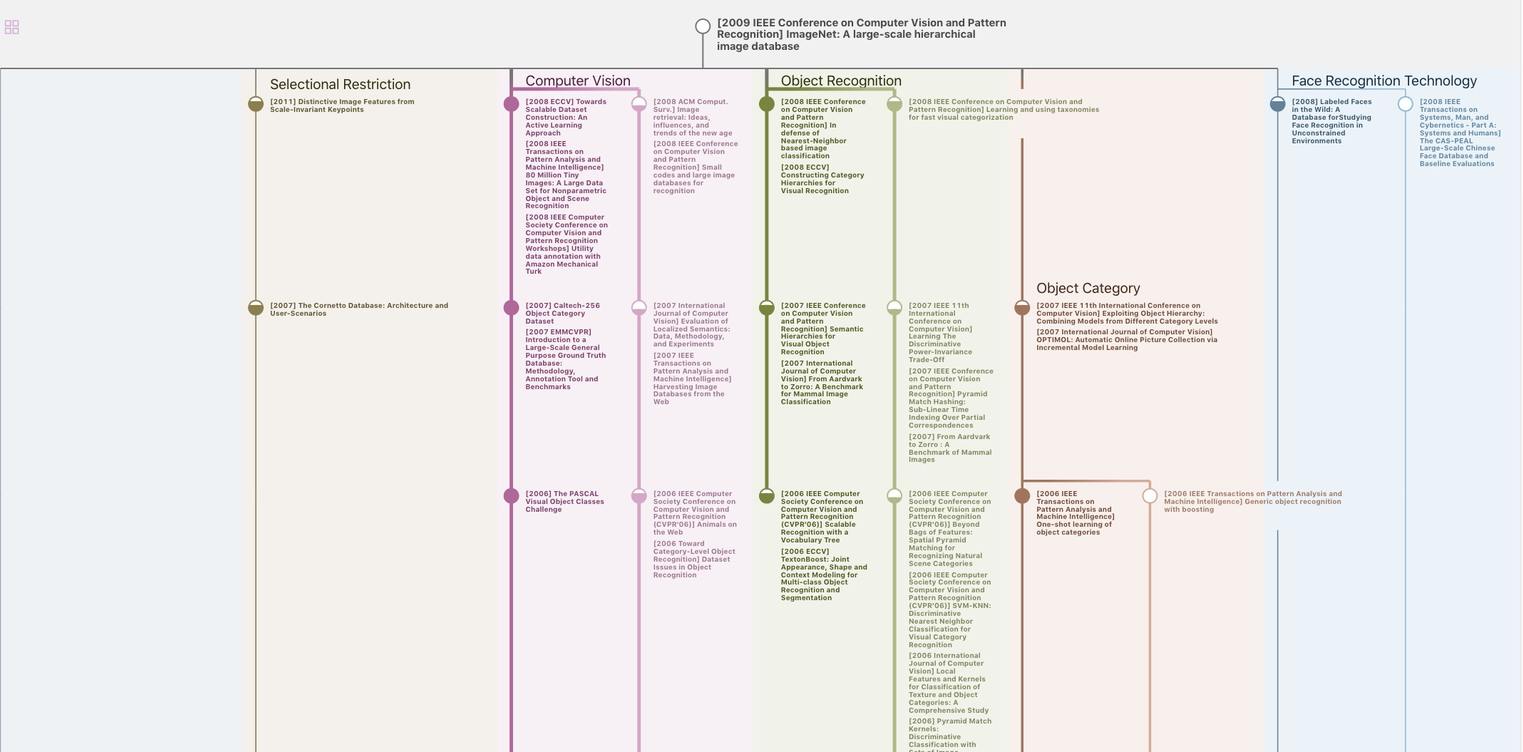
生成溯源树,研究论文发展脉络
Chat Paper
正在生成论文摘要