SV-Learn: Learning Matrix Singular Values with Neural Networks
2022 IEEE International Conference on Data Mining Workshops (ICDMW)(2022)
摘要
The singular value decomposition (SVD) factors a matrix into three separate matrices: two (semi-)unitary matrices whose columns are left/right singular vectors and one diagonal matrix whose diagonal entries are singular values. Typically, performing SVD on big matrices is taxing due to its compu-tational complexity in the cubic order of its dimensions. With the advances and rapid growth of deep learning techniques in a broad spectrum of applications, a fundamental question arises: can deep neural networks learn the singular values of a matrix? To answer this question, we propose a novel algorithm, namely SV-Iearn, to predict the singular values of a given input matrix by leveraging the advances of neural networks. Numerical results demonstrate that our proposed method outperforms the competing alternatives in terms of achieving lower normalized mean square error on singular value prediction when using real-world datasets. Further, the predicted singular values combined with singular vectors of an input data allow us to reconstruct the input matrices with promising performance.
更多查看译文
关键词
learning matrix singular values,networks,sv-learn
AI 理解论文
溯源树
样例
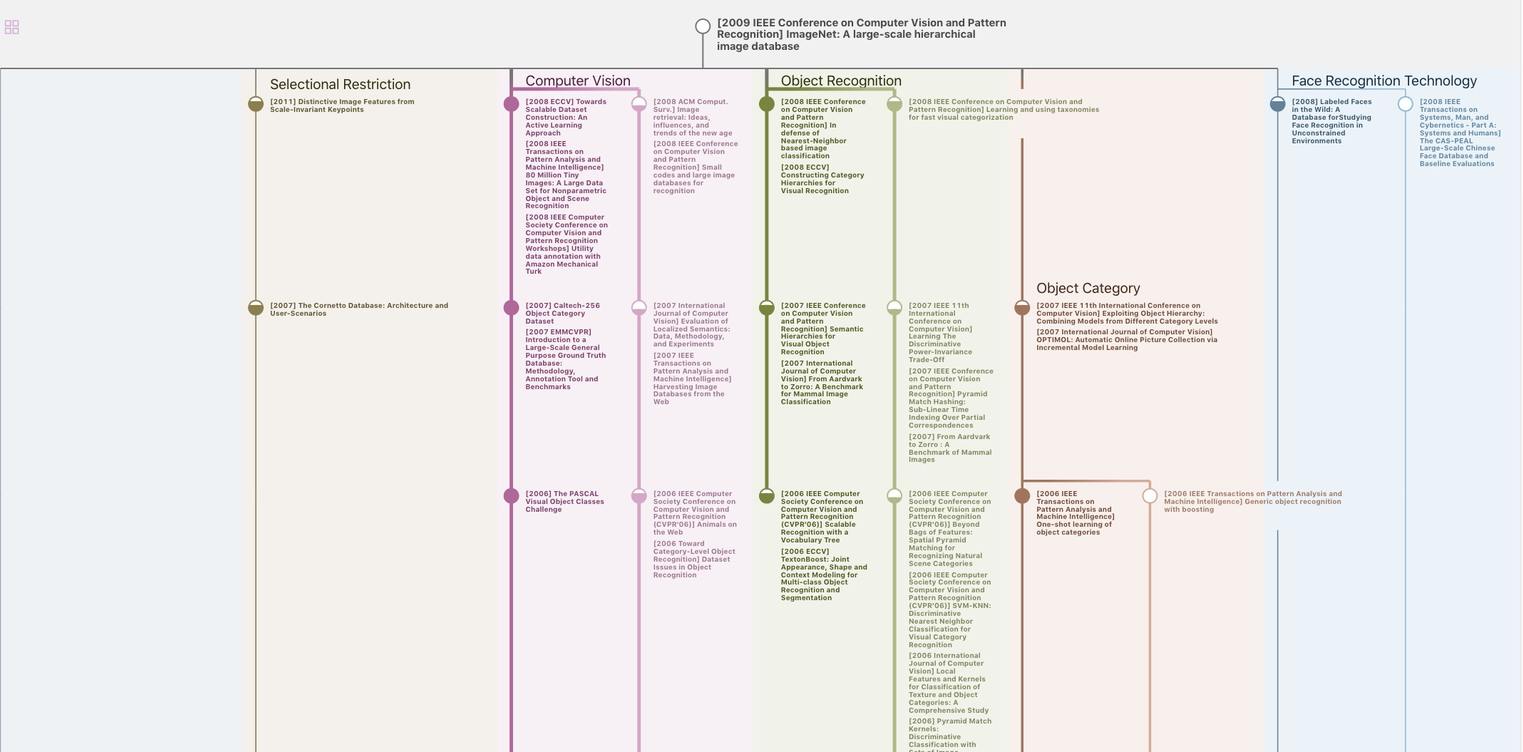
生成溯源树,研究论文发展脉络
Chat Paper
正在生成论文摘要