A Novel Deep Odometry Network for Vehicle Positioning Based on Smartphone
IEEE Transactions on Instrumentation and Measurement(2023)
摘要
Smartphone with multiple sensors integration has been widely used for navigation. The inertial measurement unit (IMU) embedded in smartphones has been widely used for pedestrian navigation for counting steps. However, it is a challenge to measure the accurate velocity of the vehicle from the smartphone-embedded IMU data with a high noise level. Thus, current vehicle navigation with a smartphone relies substantially on the Global Navigation Satellite System (GNSS), which provides unreliable positions in urban dense areas due to the blockage and the reflection of GNSS signals. In this study, we propose a smartphone-based positioning method to improve vehicle positioning performance continuously in GNSS-degraded areas through the improvement of IMU velocity estimation. A convolutional neural network–gated recurrent unit (CNN-GRU) combined deep learning odometry network, termed DeepOdo, is proposed to estimate the velocity of the vehicle with the IMU and barometer data as the input, rather than the traditional integral of the IMU measurements. Raw sensor data is utilized to boost the robustness. Labels of the DeepOdo are obtained from the integrated GNSS/IMU/barometer solutions in the smartphone which significantly simplifies the dataset collection. In GNSS-denied areas, IMU, barometer, and DeepOdo are integrated to provide accurate navigation solutions for the vehicle. Results of the proposed method show 73.14% and 98.33% improvements in horizontal and vertical directions, respectively, compared with the non-holonomic constraints (NHCs) aided IMU. Finally, the DeepOdo network is deployed in Android smartphones to demonstrate that the proposed solution can work properly on the mobile platform.
更多查看译文
关键词
Barometer,deep learning odometry,inertial measurement unit (IMU),smartphone,vehicle positioning
AI 理解论文
溯源树
样例
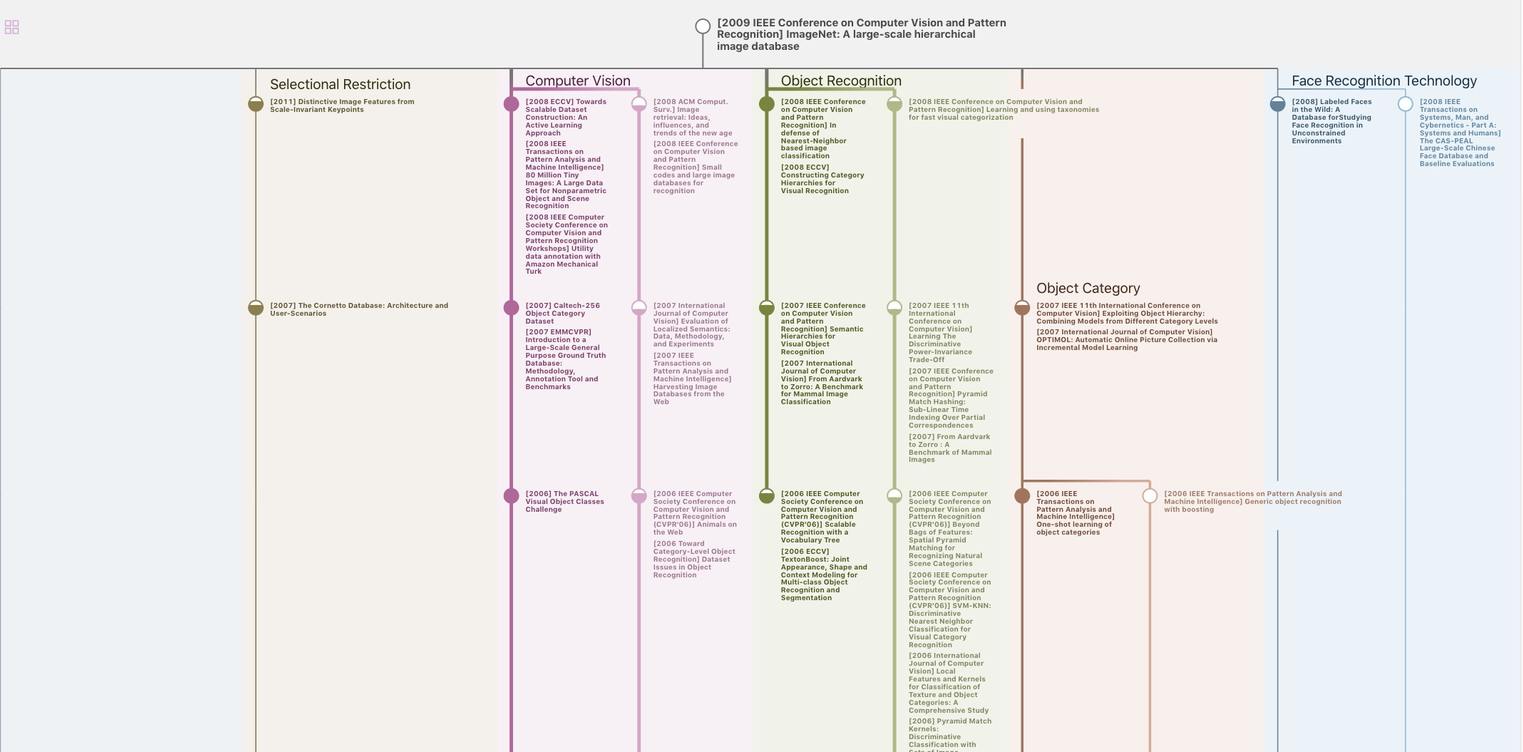
生成溯源树,研究论文发展脉络
Chat Paper
正在生成论文摘要