Quantitative level determination of fixed restorations on panoramic radiographs using deep learning
INTERNATIONAL JOURNAL OF COMPUTERIZED DENTISTRY(2023)
摘要
Aim: Although many studies in various fields employ deep learning models, only a few such studies exist in dental imaging. The present article aims to evaluate the effectiveness of convo-lutional neural network (CNN) algorithms for the detection and diagnosis of the quantitative level of dental restorations using panoramic radiographs by preparing a novel dataset. Materials and methods: 20,973 panoramic radiographs were used, all labeled into five distinct categories by three dental experts. AlexNet, VGG-16, and variants of ResNet models were trained with the dataset and evaluated for the classifi-cation task. Additionally, 10-fold cross-validation (ie, 9 folds were separated for training and 1 fold for validation) and data augmentation were carried out for all experiments. Results: The most successful result was shown by ResNet-101, with an accuracy of 92.7%. Its macro-average AUC was also the highest, at 0.989. Other accuracy results obtained for the dataset were 75.5% for AlexNet, 85.0% for VGG-16, 92.1% for ResNet-18, 91.7% for ResNet-50, and 92.1% for InceptionResNet-v2. Conclusions: An accuracy of 92.7% is a very promising result for a computer-aided diagnostic system. This result proved that the system could assist dentists in providing supportive preliminary information from the moment a patient's first panoramic radiograph is taken. Furthermore, as the introduced dataset is powerful enough, it can be re - labeled for different problems and used in different studies.
更多查看译文
关键词
artificial intelligence,computer-aided diagnosis,convolutional neural networks,deep learning,dental ation,panoramic radiograph
AI 理解论文
溯源树
样例
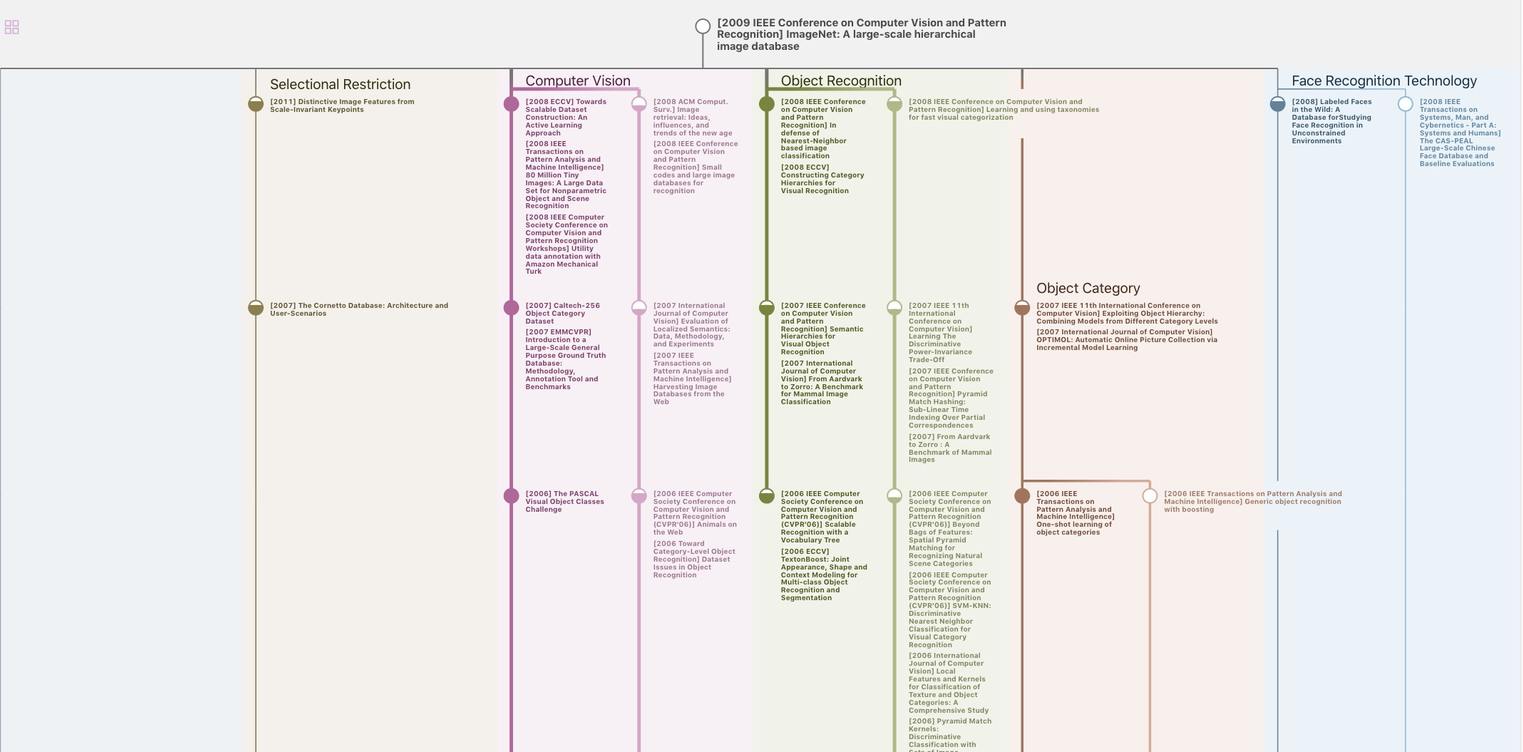
生成溯源树,研究论文发展脉络
Chat Paper
正在生成论文摘要