A Prototype-Oriented Clustering for Domain Shift with Source Privacy
CoRR(2023)
摘要
Unsupervised clustering under domain shift (UCDS) studies how to transfer the knowledge from abundant unlabeled data from multiple source domains to learn the representation of the unlabeled data in a target domain. In this paper, we introduce Prototype-oriented Clustering with Distillation (PCD) to not only improve the performance and applicability of existing methods for UCDS, but also address the concerns on protecting the privacy of both the data and model of the source domains. PCD first constructs a source clustering model by aligning the distributions of prototypes and data. It then distills the knowledge to the target model through cluster labels provided by the source model while simultaneously clustering the target data. Finally, it refines the target model on the target domain data without guidance from the source model. Experiments across multiple benchmarks show the effectiveness and generalizability of our source-private clustering method.
更多查看译文
关键词
source privacy,clustering,domain shift,prototype-oriented
AI 理解论文
溯源树
样例
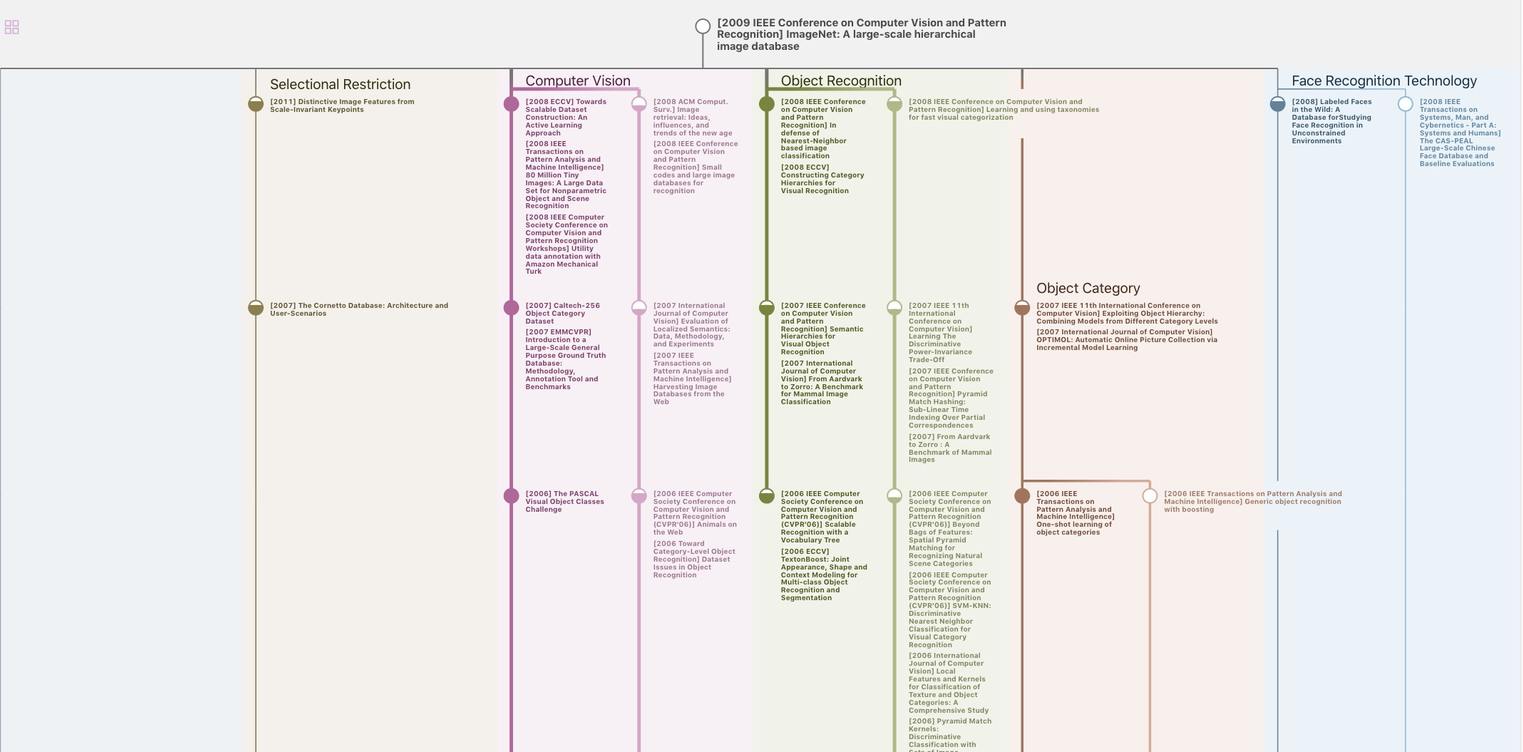
生成溯源树,研究论文发展脉络
Chat Paper
正在生成论文摘要