Optimal Stochastic Non-smooth Non-convex Optimization through Online-to-Non-convex Conversion
arxiv(2023)
摘要
We present new algorithms for optimizing non-smooth, non-convex stochastic objectives based on a novel analysis technique. This improves the current best-known complexity for finding a $(\delta,\epsilon)$-stationary point from $O(\epsilon^{-4}\delta^{-1})$ stochastic gradient queries to $O(\epsilon^{-3}\delta^{-1})$, which we also show to be optimal. Our primary technique is a reduction from non-smooth non-convex optimization to online learning, after which our results follow from standard regret bounds in online learning. For deterministic and second-order smooth objectives, applying more advanced optimistic online learning techniques enables a new complexity of $O(\epsilon^{-1.5}\delta^{-0.5})$. Our techniques also recover all optimal or best-known results for finding $\epsilon$ stationary points of smooth or second-order smooth objectives in both stochastic and deterministic settings.
更多查看译文
关键词
optimal stochastic,optimization,non-smooth,online-to-non-convex
AI 理解论文
溯源树
样例
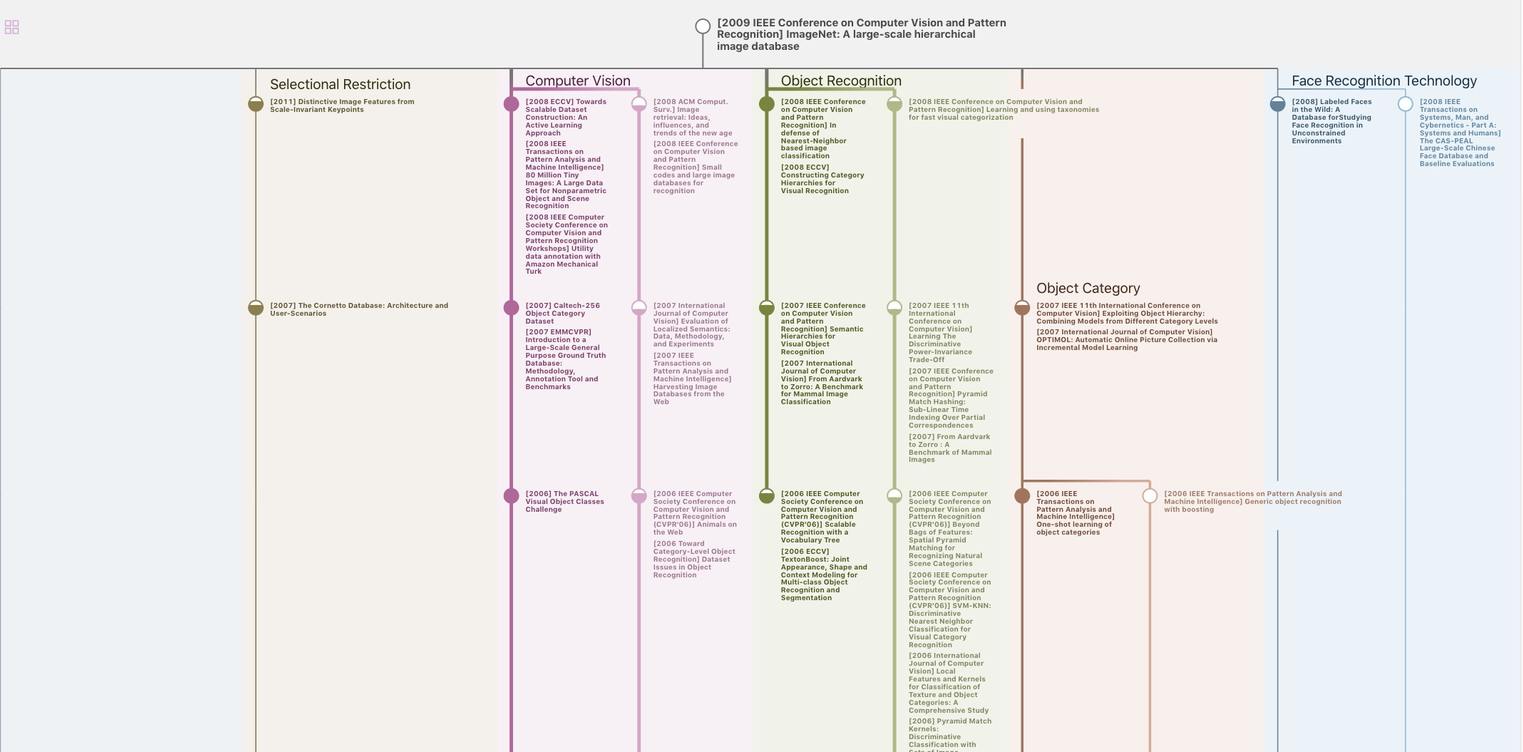
生成溯源树,研究论文发展脉络
Chat Paper
正在生成论文摘要