The Application of Reinforcement Learning to the FlipIt Security Game
2022 Cyber Research Conference - Ireland (Cyber-RCI)(2022)
摘要
Advanced Persistent Threat is a new, sophisticated type of targeted attack which is continuous, long-living, and stealthy. It has proven to be difficult to detect and defend against in the cloud-based environment by traditional methods, calling for more advanced security technologies. FlipIt is a two-player security game where an attacker and defender compete to control a sensitive resource in advanced scenarios such as APTs. Its robustness against APT attacks is outstanding. We model the FlipIt game as a Markov Decision Process and apply reinforcement learning to the framework. The goal is to find an optimal adaptive strategy for a player to compete against any unknown opponent in a FlipIt game with incomplete information. This means the best result for a player is to maximize the ownership of the resource with minimum cost. We perform experiments on single-agent and multi-agent scenarios, respectively. We further extend the model to involve noisy information and consider the openness of the game. Our experimental analysis proves that in a two-player FlipIt game, an adaptive player can automatically learn and find an optimal strategy using only the last move information of the opponent, who moves with a non-adaptive strategy (i.e. a periodic strategy with random noise). The parameters related to the random noise we considered affect the average benefit for each player. In addition, we consider the openness of the game in which new participants are introduced individually at random time steps with a certain probability. In this case, the model is generalized from two-player to n-player, and the convergence of the optimal strategy learned by each player is confirmed. Moreover, we demonstrate that varying the probability of adding an additional player does not affect the convergence but changes the average benefits for players.
更多查看译文
关键词
Security Games,Advanced Persistent Threats,FlipIt,Reinforcement Learning,Adaptive Strategy,Random Noise,Game Openness
AI 理解论文
溯源树
样例
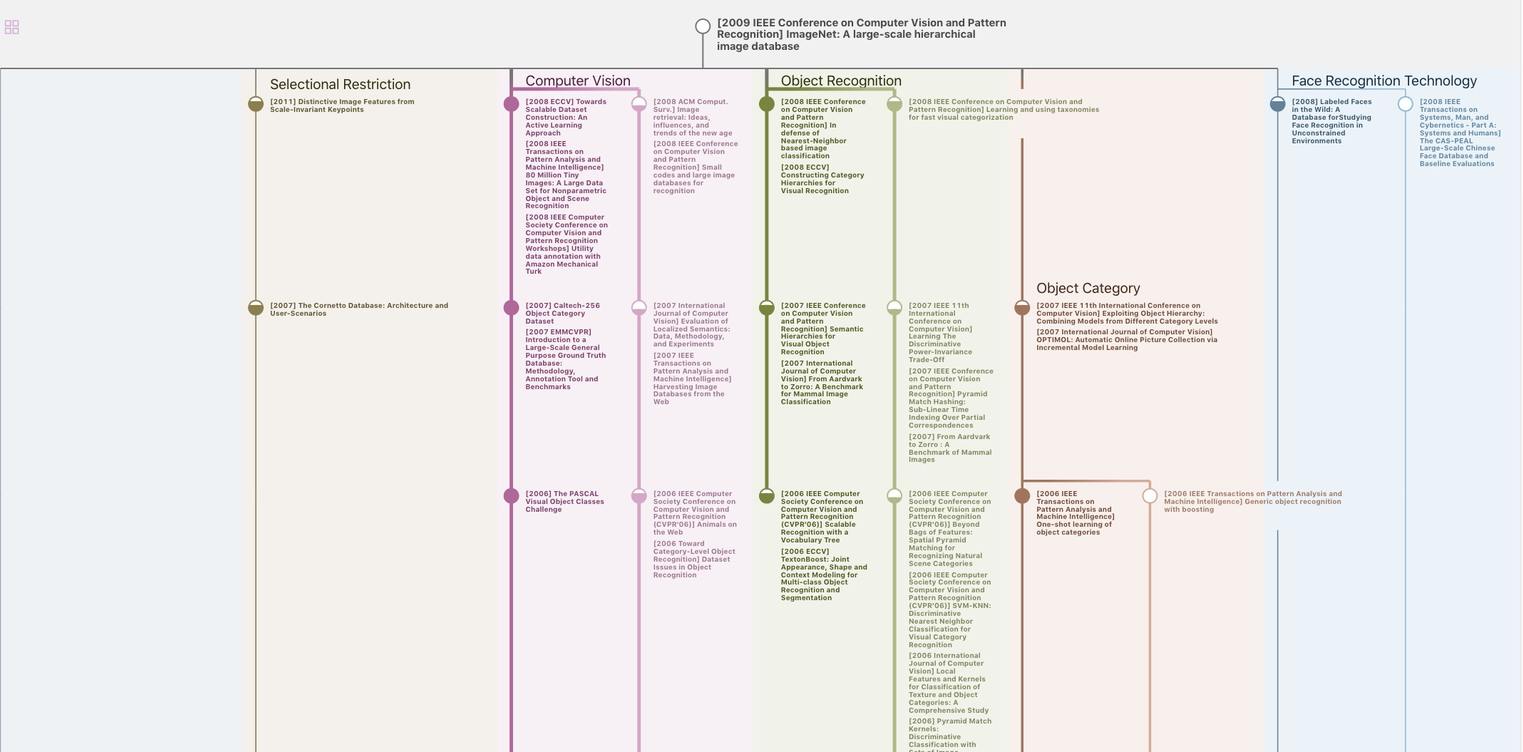
生成溯源树,研究论文发展脉络
Chat Paper
正在生成论文摘要