Seasonal forecasting skill for the High Mountain Asia region in the GoddardEarth Observing System
Earth System Dynamics(2023)
Abstract
Seasonal variability of the global hydrologic cycle directly impacts human activities, including hazard assessment and mitigation, agricultural decisions, and water resources management. This is particularly true across the High Mountain Asia (HMA) region, where availability of water resources can change depending on local seasonality of the hydrologic cycle. Forecasting the atmospheric states and surface conditions, including hydrometeorologically relevant variables, at subseasonal-to-seasonal (S2S) lead times of weeks to months is an area of active research and development. NASA's Goddard Earth Observing System (GEOS) S2S prediction system has been developed with this research goal in mind. Here, we benchmark the forecast skill of GEOS-S2S (version 2) hydrometeorological forecasts at 1-3-month lead times in the HMA region, including a portion of the Indian subcontinent, during the retrospective forecast period, 1981-2016. To assess forecast skill, we evaluate 2 m air temperature, total precipitation, fractional snow cover, snow water equivalent, surface soil moisture, and terrestrial water storage forecasts against the Modern-Era Retrospective analysis for Research and Applications, Version 2 (MERRA-2) and independent reanalysis data, satellite observations, and data fusion products. Anomaly correlation is highest when the forecasts are evaluated against MERRA-2 and particularly in variables with long memory in the climate system, likely due to the similar initial conditions and model architecture used in GEOS-S2S and MERRA-2. When compared to MERRA-2, results for the 1-month forecast skill range from an anomaly correlation of R-anom=0.18 for precipitation to R-anom=0.62 for soil moisture. Anomaly correlations are consistently lower when forecasts are evaluated against independent observations; results for the 1-month forecast skill range from R-anom=0.13 for snow water equivalent to R-anom=0.24 for fractional snow cover. We find that, generally, hydrometeorological forecast skill is dependent on the forecast lead time, the memory of the variable within the physical system, and the validation dataset used. Overall, these results benchmark the GEOS-S2S system's ability to forecast HMA hydrometeorology.
MoreTranslated text
Key words
seasonal forecasting skill,high mountain asia region,goddard earth
AI Read Science
Must-Reading Tree
Example
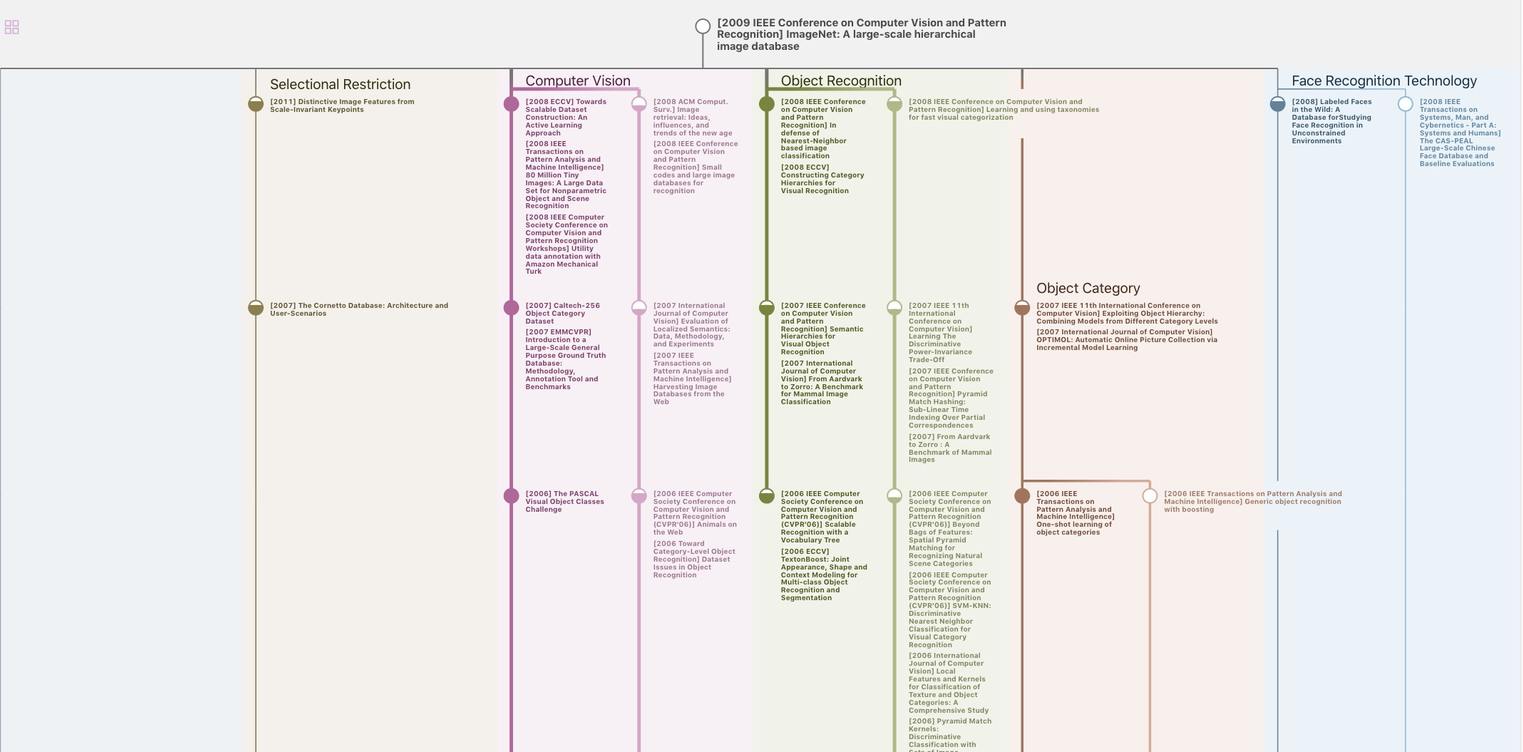
Generate MRT to find the research sequence of this paper
Chat Paper
Summary is being generated by the instructions you defined