Linear Partial Monitoring for Sequential Decision Making Algorithms, Regret Bounds and Applications
JOURNAL OF MACHINE LEARNING RESEARCH(2023)
摘要
Partial monitoring is an expressive framework for sequential decision-making with an abundance of applications, including graph-structured and dueling bandits, dynamic pricing and transductive feedback models. We survey and extend recent results on the linear formulation of partial monitoring that naturally generalizes the standard linear bandit setting. The main result is that a single algorithm, information-directed sampling (IDS), is (nearly) worst-case rate optimal in all finite-action games. We present a simple and unified analysis of stochastic partial monitoring, and further extend the model to the contextual and kernelized setting.
更多查看译文
关键词
Sequential Decision-Making,Linear Partial Monitoring,Information-Directed Sampling,Linear Bandits
AI 理解论文
溯源树
样例
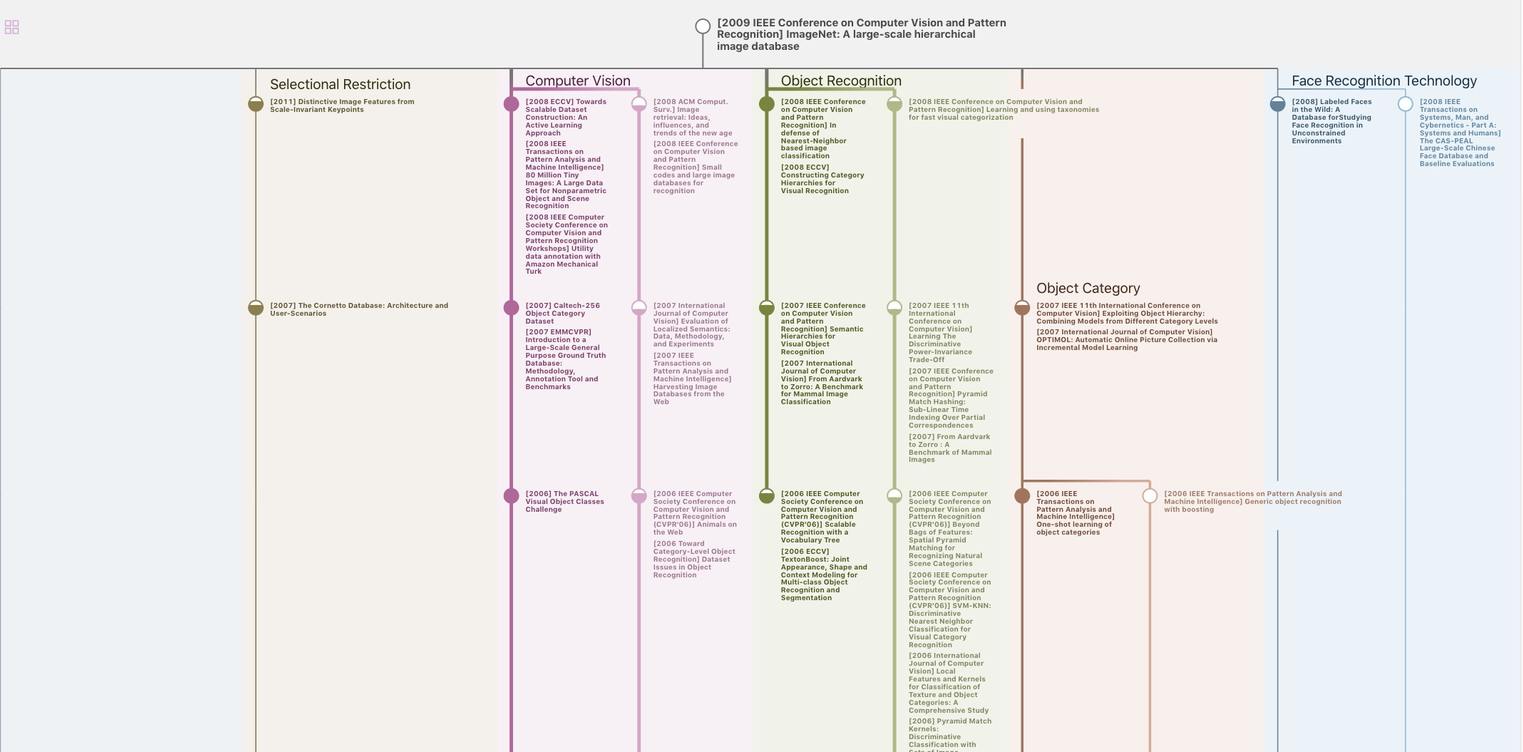
生成溯源树,研究论文发展脉络
Chat Paper
正在生成论文摘要