To be forgotten or to be fair: unveiling fairness implications of machine unlearning methods
arxiv(2024)
摘要
The right to be forgotten (RTBF) allows individuals to request the removal of personal information from online platforms. Researchers have proposed machine unlearning algorithms as a solution for erasing specific data from trained models to support RTBF. However, these methods modify how data are fed into the model and how training is done, which may subsequently compromise AI ethics from the fairness perspective. To help AI practitioners make responsible decisions when adopting these unlearning methods, we present the first study on machine unlearning methods to reveal their fairness implications. We designed and conducted experiments on two typical machine unlearning methods (SISA and AmnesiacML) along with a retraining method (ORTR) as baseline using three fairness datasets under three different deletion strategies. Results show that non-uniform data deletion with the variant of SISA leads to better fairness compared to ORTR and AmnesiacML, while initial training and uniform data deletion do not necessarily affect the fairness of all three methods. This research can help practitioners make informed decisions when implementing RTBF solutions that consider potential trade-offs on fairness.
更多查看译文
关键词
Fairness,Machine Unlearning,Artificial Intelligence,Privacy,Right to be Forgotten
AI 理解论文
溯源树
样例
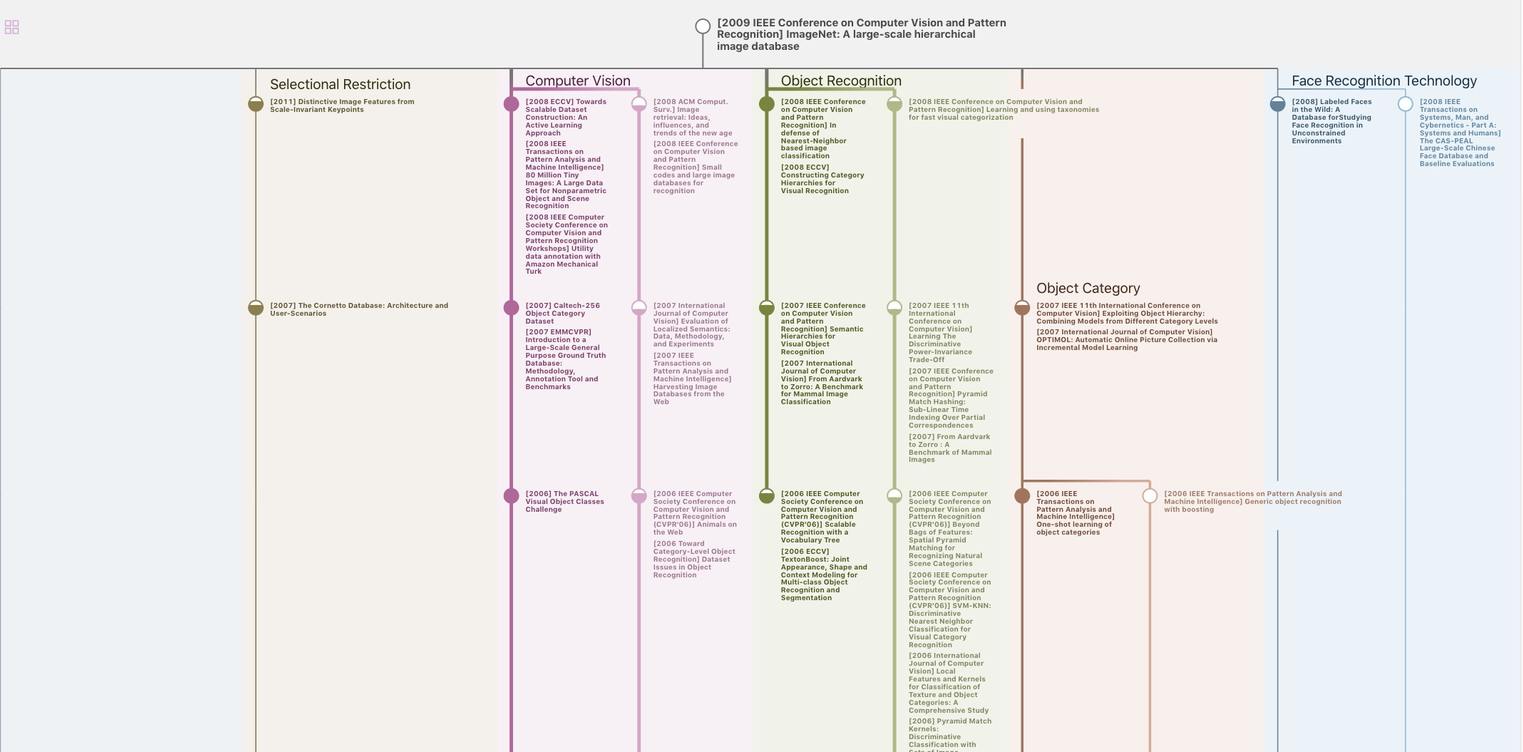
生成溯源树,研究论文发展脉络
Chat Paper
正在生成论文摘要