Multi-organ segmentation: a progressive exploration of learning paradigms under scarce annotation
PHYSICS IN MEDICINE AND BIOLOGY(2024)
摘要
Precise delineation of multiple organs or abnormal regions in the human body from medical images plays an essential role in computer-aided diagnosis, surgical simulation, image-guided interventions, and especially in radiotherapy treatment planning. Thus, it is of great significance to explore automatic segmentation approaches, among which deep learning-based approaches have evolved rapidly and witnessed remarkable progress in multi-organ segmentation. However, obtaining an appropriately sized and fine-grained annotated dataset of multiple organs is extremely hard and expensive. Such scarce annotation limits the development of high-performance multi-organ segmentation models but promotes many annotation-efficient learning paradigms. Among these, studies on transfer learning leveraging external datasets, semi-supervised learning including unannotated datasets and partially-supervised learning integrating partially-labeled datasets have led the dominant way to break such dilemmas in multi-organ segmentation. We first review the fully supervised method, then present a comprehensive and systematic elaboration of the 3 abovementioned learning paradigms in the context of multi-organ segmentation from both technical and methodological perspectives, and finally summarize their challenges and future trends.
更多查看译文
关键词
Multi-organ segmentation,annotation-efficient,transfer learning,semi-supervised learning,partial-supervised learning
AI 理解论文
溯源树
样例
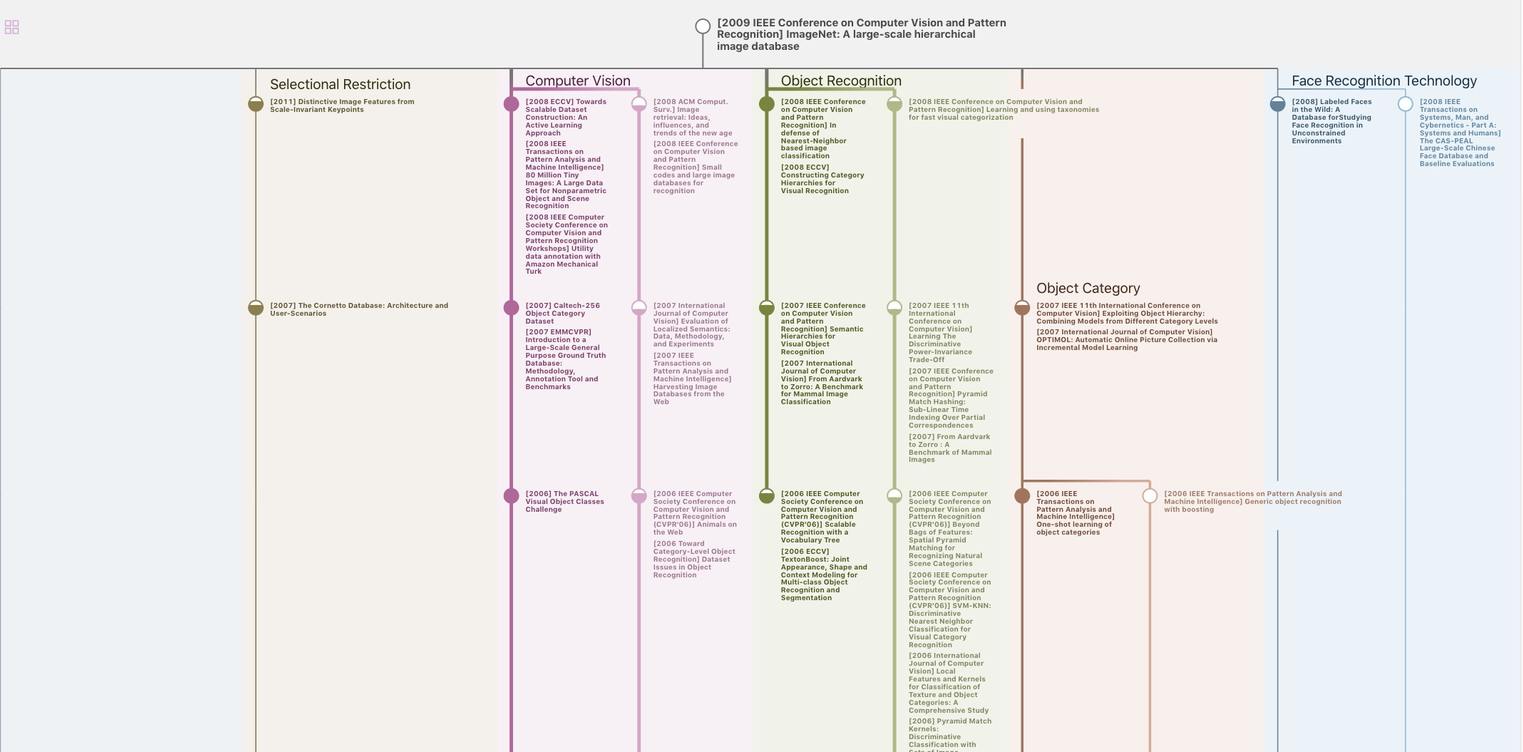
生成溯源树,研究论文发展脉络
Chat Paper
正在生成论文摘要