It's about Time: Rethinking Evaluation on Rumor Detection Benchmarks using Chronological Splits
arxiv(2023)
摘要
New events emerge over time influencing the topics of rumors in social media. Current rumor detection benchmarks use random splits as training, development and test sets which typically results in topical overlaps. Consequently, models trained on random splits may not perform well on rumor classification on previously unseen topics due to the temporal concept drift. In this paper, we provide a re-evaluation of classification models on four popular rumor detection benchmarks considering chronological instead of random splits. Our experimental results show that the use of random splits can significantly overestimate predictive performance across all datasets and models. Therefore, we suggest that rumor detection models should always be evaluated using chronological splits for minimizing topical overlaps.
更多查看译文
关键词
rumor detection benchmarks
AI 理解论文
溯源树
样例
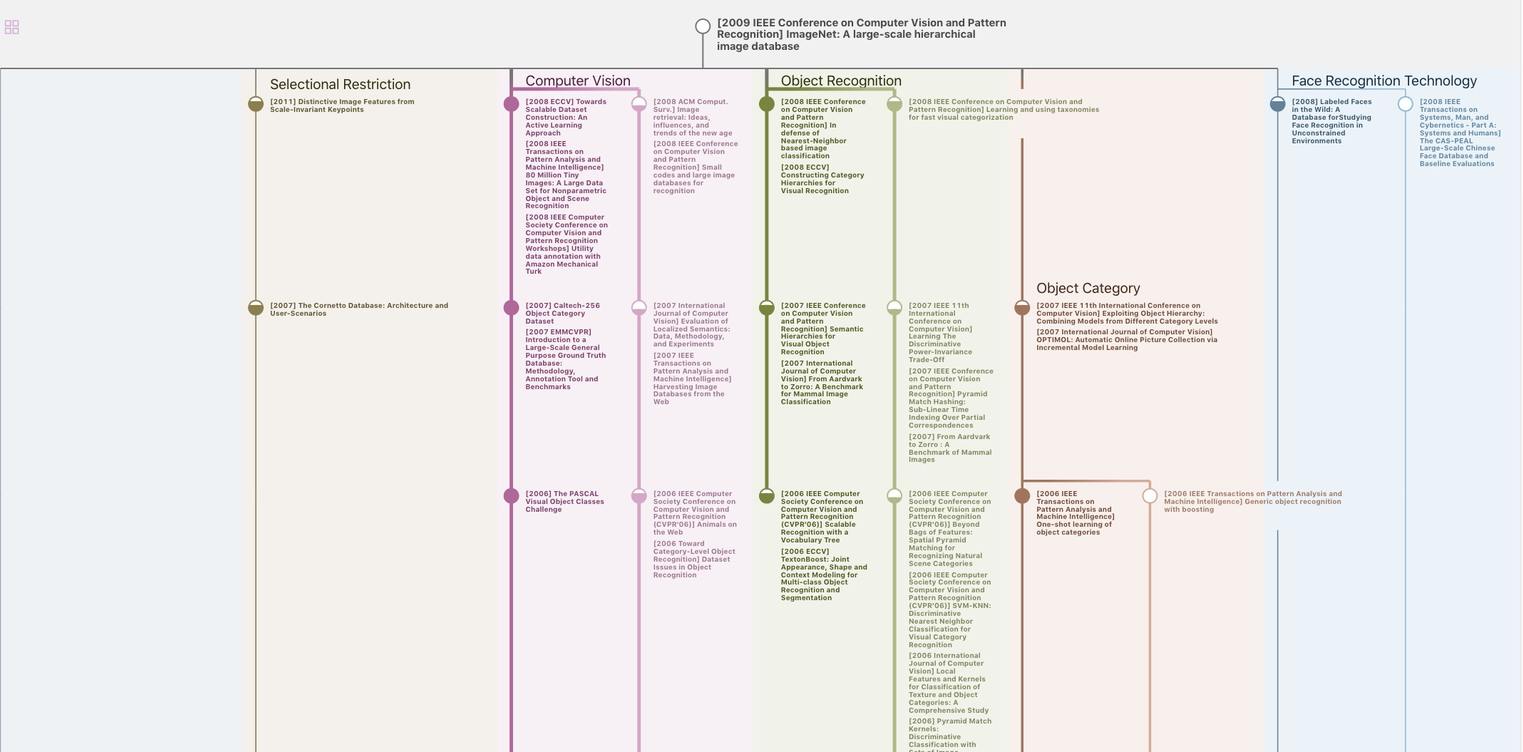
生成溯源树,研究论文发展脉络
Chat Paper
正在生成论文摘要