HMM-Boost: Improved Time Series State Prediction Via Supervised Hidden Markov Models: Case Studies in Epileptic Seizure and Complex Care Management
2022 IEEE International Conference on Knowledge Graph (ICKG)(2022)
摘要
We give a method for time series state prediction with a lazy teacher who only partially labels states, in particular only those states of an extreme nature. Hence, the labeling is not only lazy, but biased. Our method has two stages: (i) Impute new state labels for unlabeled states using a relabeling Hidden Markov Model, and in so doing treat the labeling bias. (ii) Use a supervised framework with the relabeled data. Our method is general, agnostic to the application and the supervised framework being used. We show compelling results in synthetic data and two real applications: epilepsy and complex care management. Our HMM-relabeling approach allows us to tackle time series with extremely sparse labels.
更多查看译文
关键词
HMM,Semi Supervised Learning,Time Series
AI 理解论文
溯源树
样例
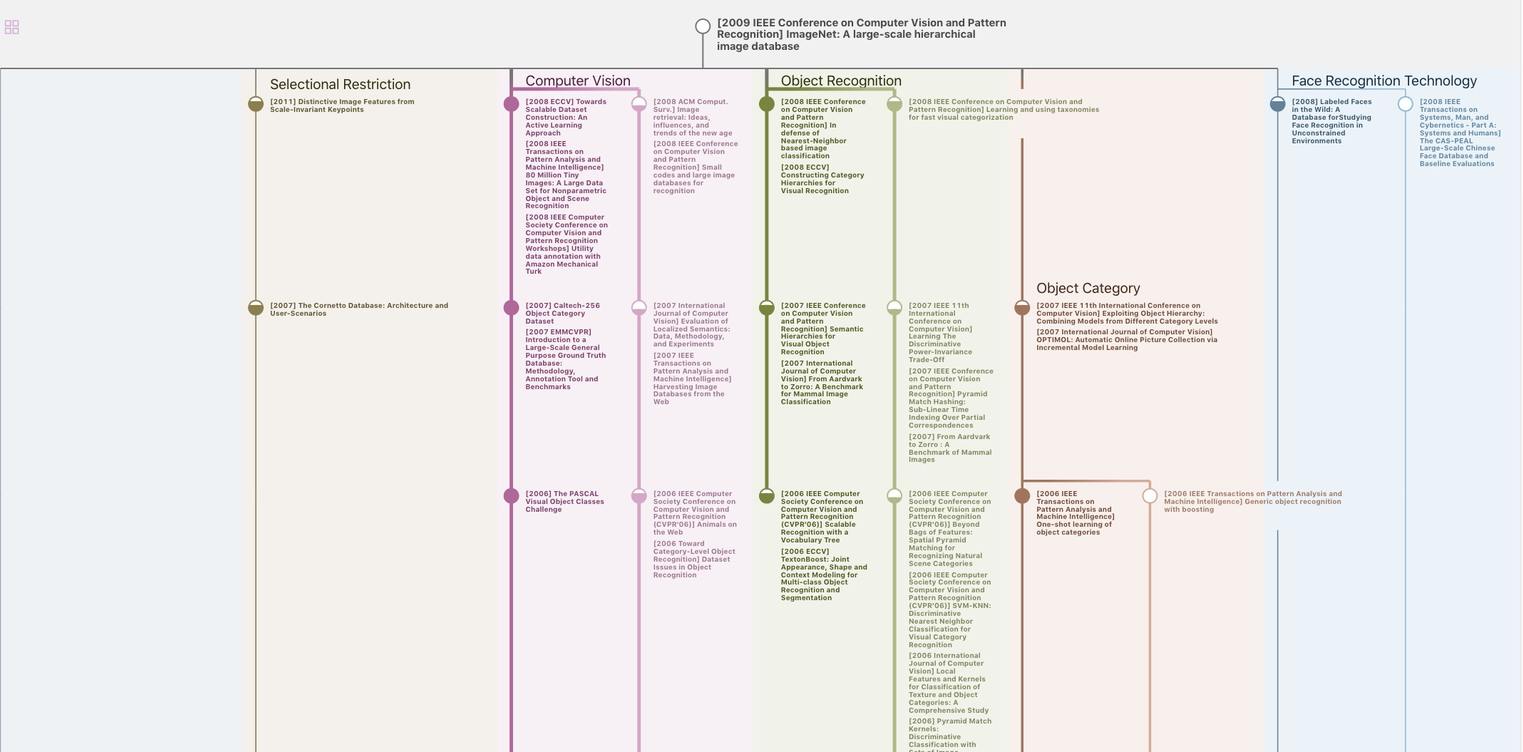
生成溯源树,研究论文发展脉络
Chat Paper
正在生成论文摘要