Multi-Sentence Matching via Exploiting List-level Semantics Expansion
2022 IEEE International Conference on Knowledge Graph (ICKG)(2022)
摘要
Sentence semantic matching plays a fundamental role in natural language understanding and is widely used in various tasks. Previous methods are mainly based on the One-to-One single pair matching process, while in many real application scenarios, such as user query matching in FAQ, we need to match multiple sentences with the specified query sentence. The reality is that the multi-sentence matching problem is largely unexplored. Although to some extent, we can use multiple One-to-One pair matching on the multi-sentence matching problem, the exploitation of list-level candidate semantics is still limited, which leads to inferior performance. To that end, in this paper, we present a focused study on the novel multi-sentence matching problem. To begin with, we propose a Multi-Sentence Matching Network (MSMN) with a meticulously designed Fusion Module and Contrastive Matching Module to deeply exploit the list-level candidate semantics expansion for the multi-sentence matching task. Then, we construct two multi-sentence matching datasets, i.e., mQuora and mReceipt, to bridge the gap of benchmark data in this area. Finally, we conduct extensive experiments on two datasets. The experimental results clearly demonstrate the effectiveness of our method.
更多查看译文
关键词
Multi-Sentence Matching,Contrastive Learning,Semantic Representation
AI 理解论文
溯源树
样例
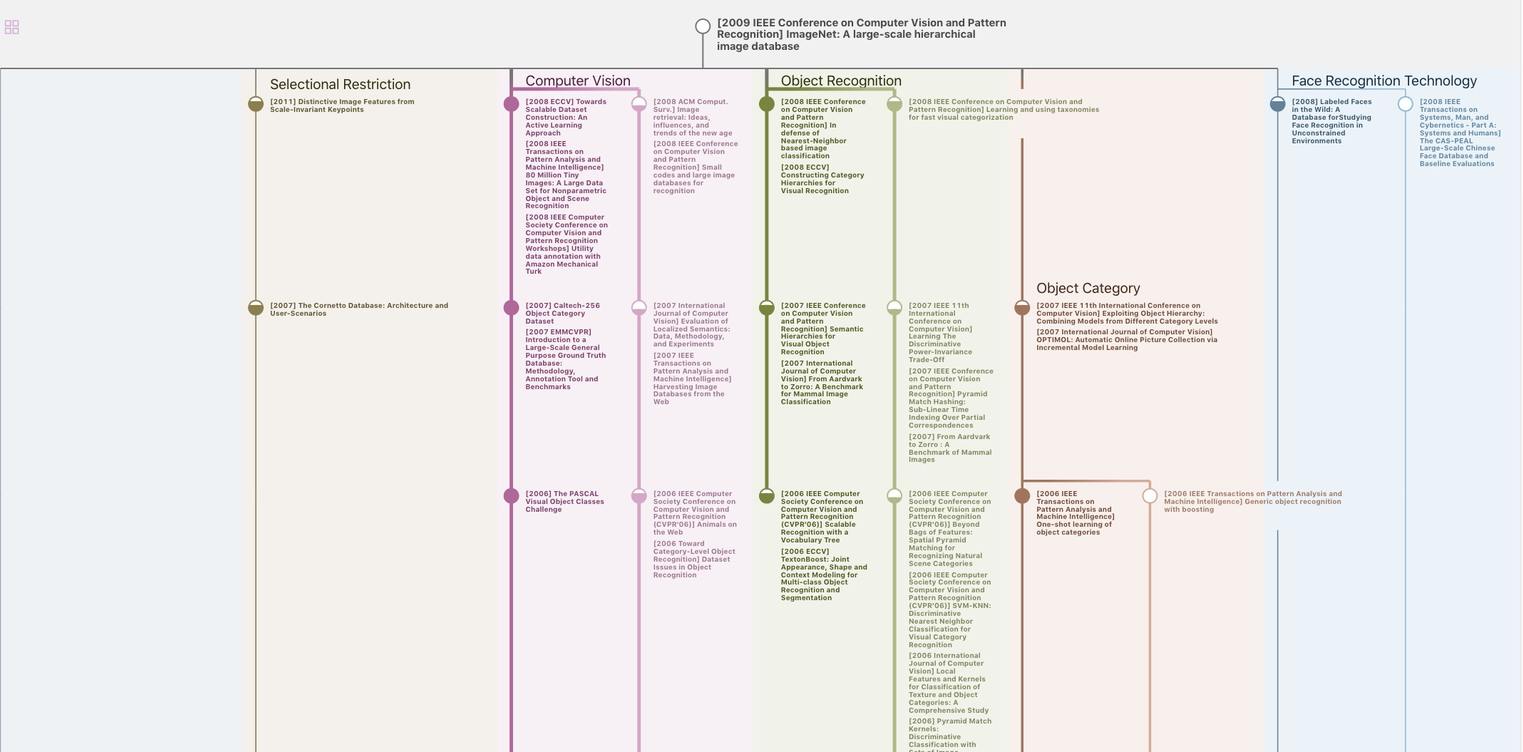
生成溯源树,研究论文发展脉络
Chat Paper
正在生成论文摘要