Deep Feature and Domain Knowledge Fusion Network for Mapping Surface Water Bodies by Fusing Google Earth RGB and Sentinel-2 Images
IEEE Geoscience and Remote Sensing Letters(2023)
摘要
Mapping surface water bodies from fine spatial resolution optical remote sensing imagery is essential for the understanding of the global hydrologic cycle. Although satellite data are useful for mapping, the limited spectral information captured by some satellite systems can be suboptimal for the task. For example, the very high-resolution images of Google Earth (GE) only contain RGB bands, which often means many water bodies and land objects are confused. Sentinel-2 (S2) imagery has a spectral resolution more suitable for mapping water bodies, but its medium spatial resolution limits the ability for detailed mapping of water-land boundaries. This letter proposes a deep feature and domain knowledge fusion network (DFDKFNet) for mapping surface water bodies by fusing GE and S2 images while incorporating domain knowledge. DFDKFNet uses the remote sensing indices of normalized difference water index (NDWI) and normalized difference vegetation index (NDVI) derived from the S2 image as the representative domain knowledge to better extract water bodies from terrestrial features. A similar pixel-based approach is used to downscale the NDWI and NDVI maps to match the spatial resolution between the GE and S2 images. The DFDKFNet uses the GE and downscaled NDWI and NDVI images to extract the deep semantic features of water bodies, which are fused with the domain knowledge extracted from the NDWI and NDVI images. DFDKFNet was compared with several state-of-the-art algorithms, and the results show that DFDKFNet can enhance water body mapping accuracy.
更多查看译文
关键词
Deep learning (DL),domain knowledge,water body
AI 理解论文
溯源树
样例
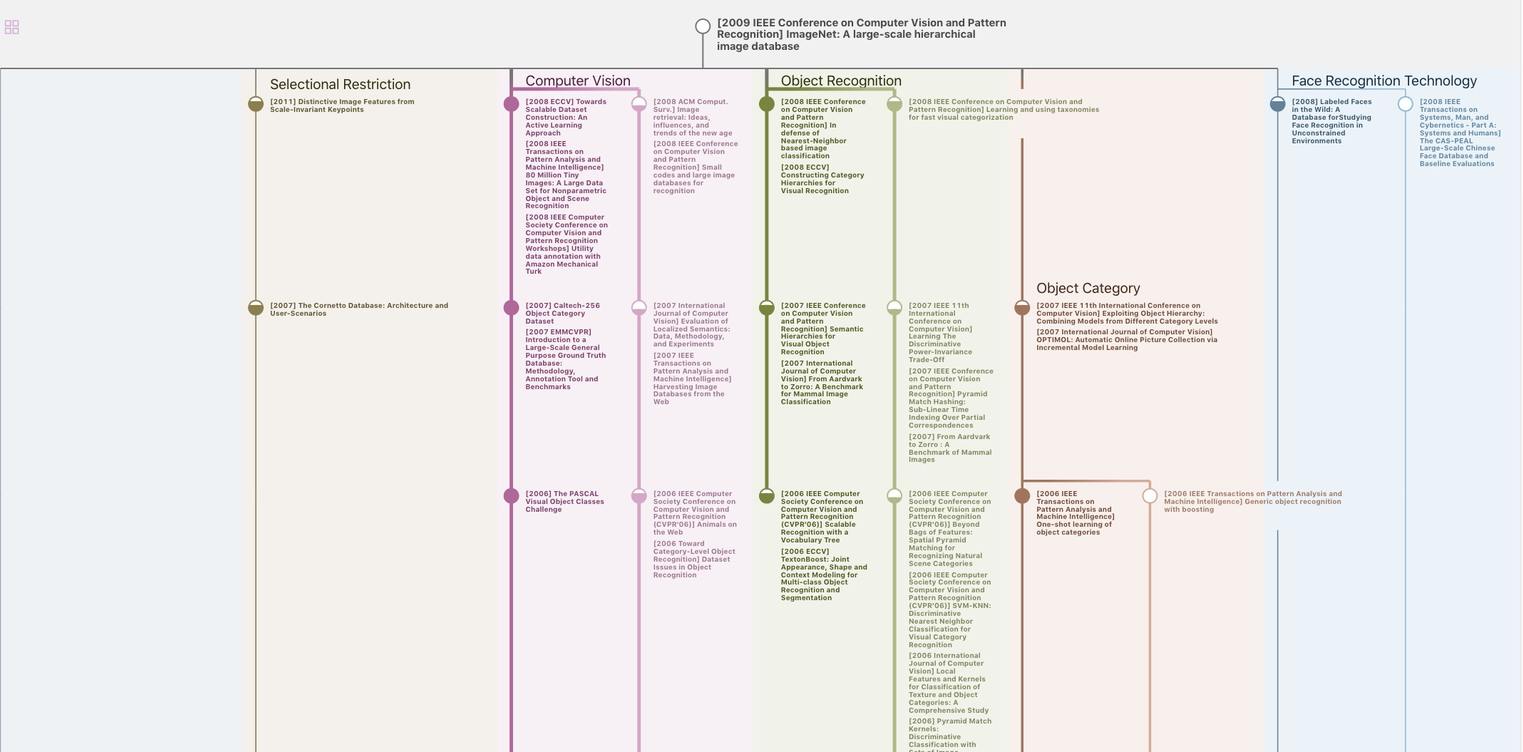
生成溯源树,研究论文发展脉络
Chat Paper
正在生成论文摘要