Adaptive Parameterization of Deep Learning Models for Federated Learning
arxiv(2023)
摘要
Federated Learning offers a way to train deep neural networks in a distributed fashion. While this addresses limitations related to distributed data, it incurs a communication overhead as the model parameters or gradients need to be exchanged regularly during training. This can be an issue with large scale distribution of learning asks and negate the benefit of the respective resource distribution. In this paper, we we propose to utilise parallel Adapters for Federated Learning. Using various datasets, we show that Adapters can be applied with different Federated Learning techniques. We highlight that our approach can achieve similar inference performance compared to training the full model while reducing the communication overhead drastically. We further explore the applicability of Adapters in cross-silo and cross-device settings, as well as different non-IID data distributions.
更多查看译文
关键词
federated learning,deep learning models,deep learning
AI 理解论文
溯源树
样例
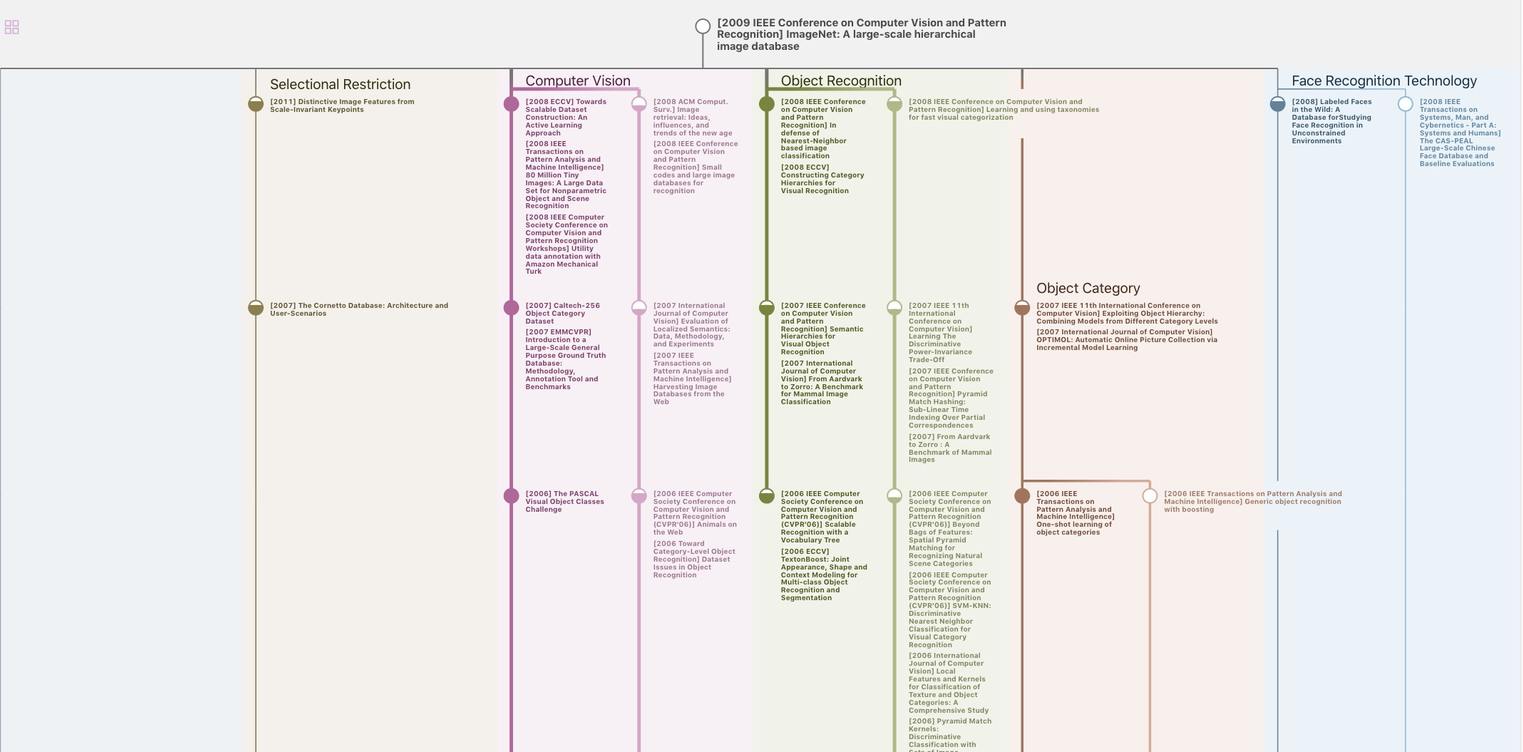
生成溯源树,研究论文发展脉络
Chat Paper
正在生成论文摘要