Penalized Quasi-likelihood Estimation and Model Selection in Time Series Models with Parameters on the Boundary
arxiv(2023)
摘要
We extend the theory from Fan and Li (2001) on penalized likelihood-based estimation and model-selection to statistical and econometric models which allow for non-negativity constraints on some or all of the parameters, as well as time-series dependence. It differs from classic non-penalized likelihood estimation, where limiting distributions of likelihood-based estimators and test-statistics are non-standard, and depend on the unknown number of parameters on the boundary of the parameter space. Specifically, we establish that the joint model selection and estimation, results in standard asymptotic Gaussian distributed estimators. The results are applied to the rich class of autoregressive conditional heteroskedastic (ARCH) models for the modelling of time-varying volatility. We find from simulations that the penalized estimation and model-selection works surprisingly well even for a large number of parameters. A simple empirical illustration for stock-market returns data confirms the ability of the penalized estimation to select ARCH models which fit nicely the autocorrelation function, as well as confirms the stylized fact of long-memory in financial time series data.
更多查看译文
关键词
model selection,models,estimation,time series
AI 理解论文
溯源树
样例
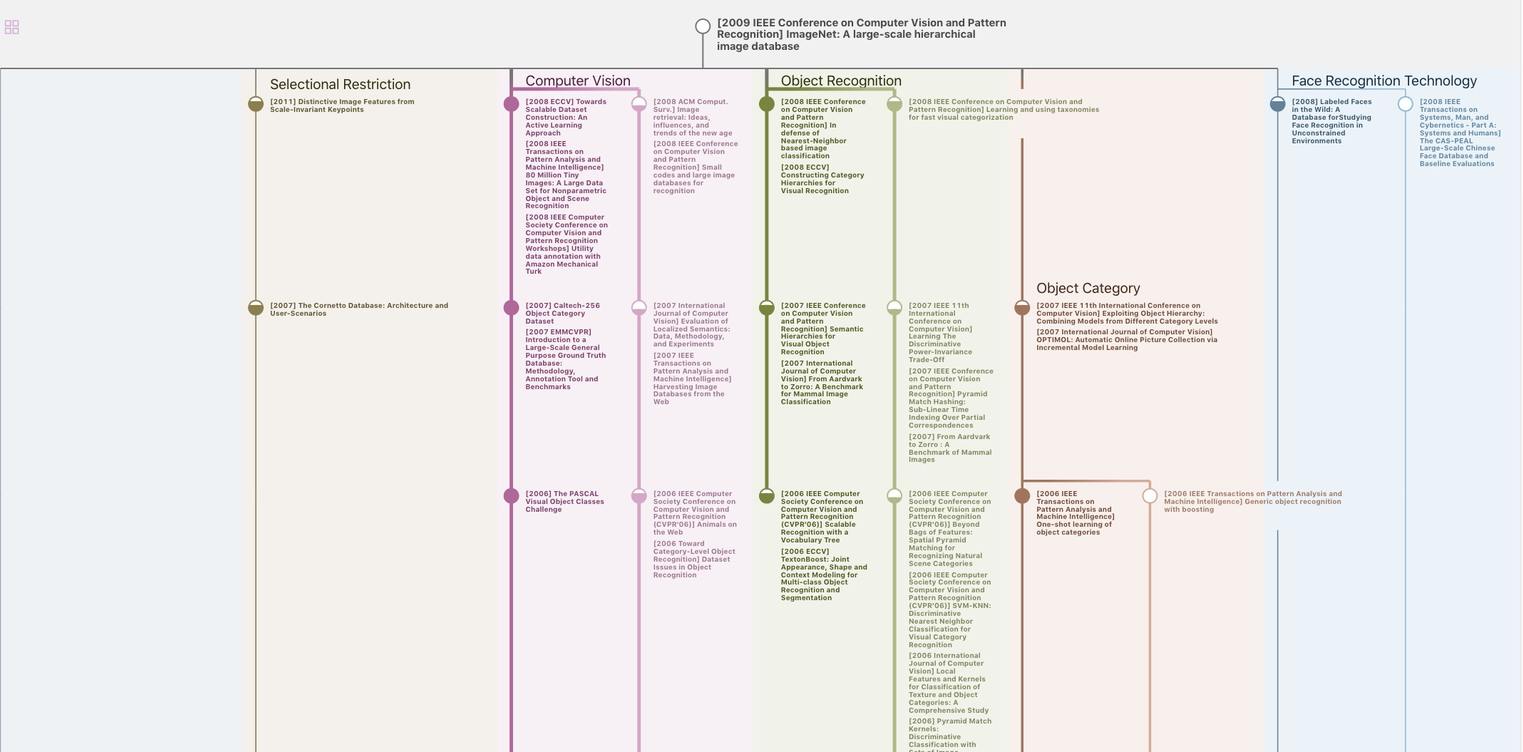
生成溯源树,研究论文发展脉络
Chat Paper
正在生成论文摘要