ProbPNN: Enhancing Deep Probabilistic Forecasting with Statistical Information
arxiv(2023)
摘要
Probabilistic forecasts are essential for various downstream applications such as business development, traffic planning, and electrical grid balancing. Many of these probabilistic forecasts are performed on time series data that contain calendar-driven periodicities. However, existing probabilistic forecasting methods do not explicitly take these periodicities into account. Therefore, in the present paper, we introduce a deep learning-based method that considers these calendar-driven periodicities explicitly. The present paper, thus, has a twofold contribution: First, we apply statistical methods that use calendar-driven prior knowledge to create rolling statistics and combine them with neural networks to provide better probabilistic forecasts. Second, we benchmark ProbPNN with state-of-the-art benchmarks by comparing the achieved normalised continuous ranked probability score (nCRPS) and normalised Pinball Loss (nPL) on two data sets containing in total more than 1000 time series. The results of the benchmarks show that using statistical forecasting components improves the probabilistic forecast performance and that ProbPNN outperforms other deep learning forecasting methods whilst requiring less computation costs.
更多查看译文
关键词
deep probabilistic forecasting,information
AI 理解论文
溯源树
样例
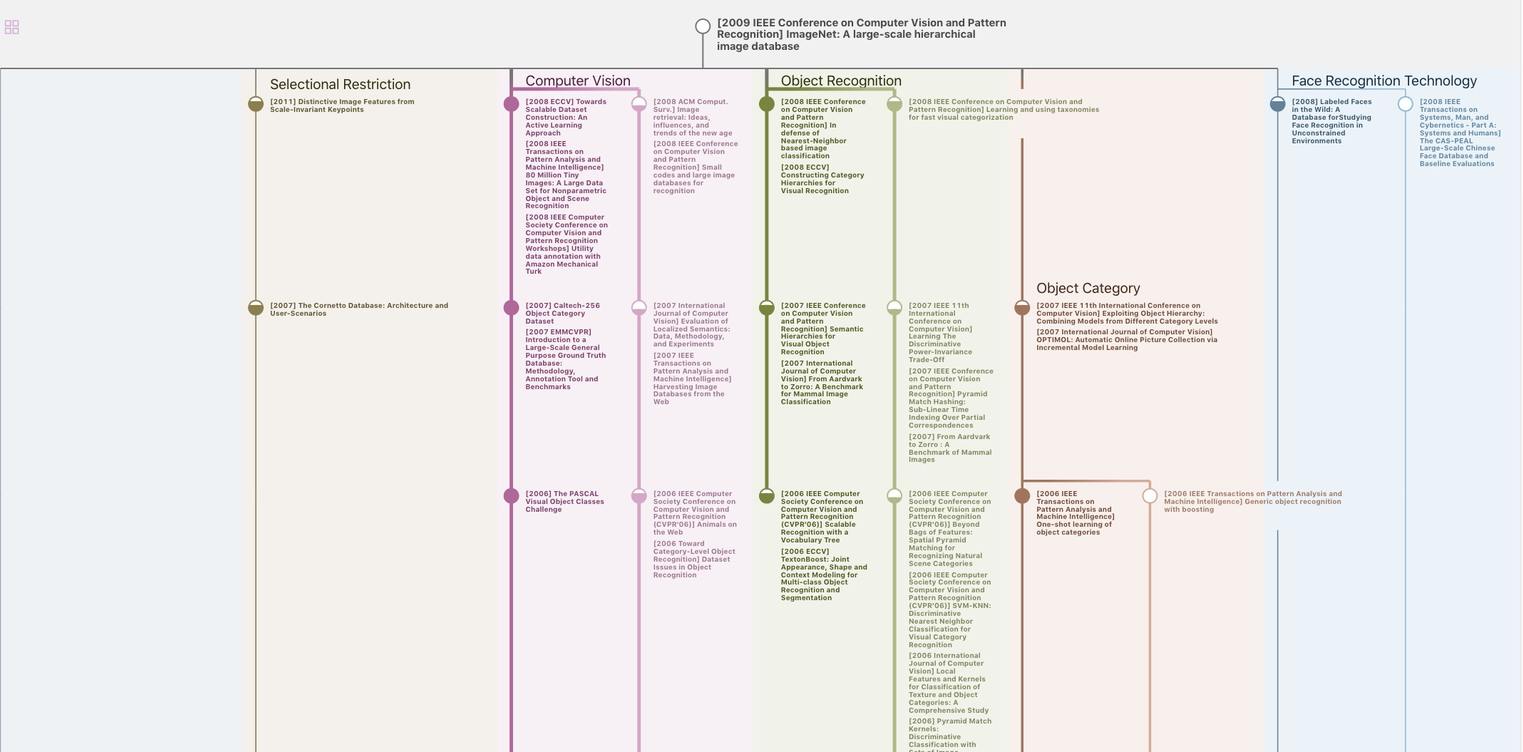
生成溯源树,研究论文发展脉络
Chat Paper
正在生成论文摘要