Precursor recommendation for inorganic synthesis by machine learning materials similarity from scientific literature
arxiv(2023)
摘要
Synthesis prediction is a key accelerator for the rapid design of advanced materials. However, determining synthesis variables such as the choice of precursor materials is challenging for inorganic materials because the sequence of reactions during heating is not well understood. In this work, we use a knowledge base of 29,900 solid-state synthesis recipes, text-mined from the scientific literature, to automatically learn which precursors to recommend for the synthesis of a novel target material. The data-driven approach learns chemical similarity of materials and refers the synthesis of a new target to precedent synthesis procedures of similar materials, mimicking human synthesis design. When proposing five precursor sets for each of 2654 unseen test target materials, the recommendation strategy achieves a success rate of at least 82%. Our approach captures decades of heuristic synthesis data in a mathematical form, making it accessible for use in recommendation engines and autonomous laboratories.
更多查看译文
关键词
machine learning materials similarity,inorganic synthesis,machine learning
AI 理解论文
溯源树
样例
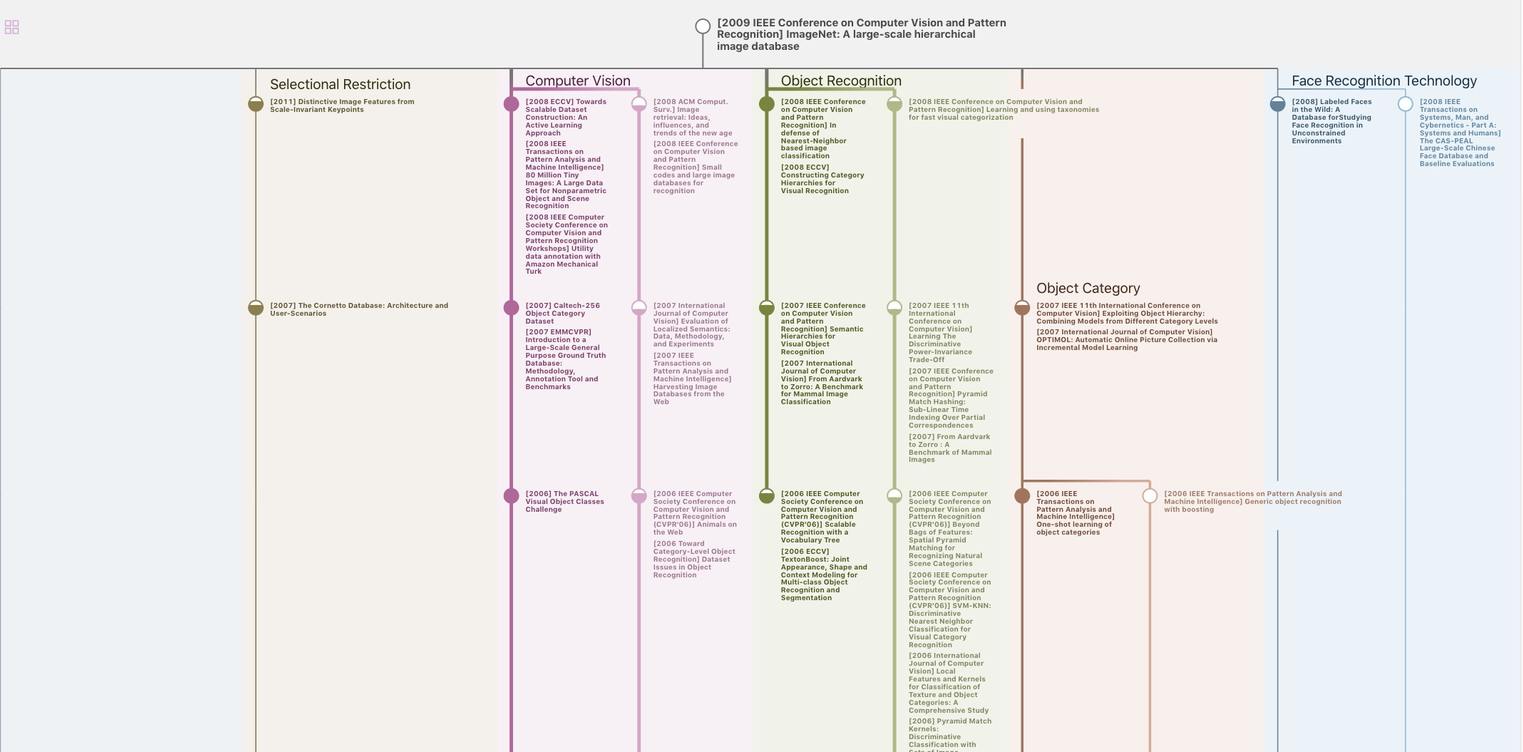
生成溯源树,研究论文发展脉络
Chat Paper
正在生成论文摘要