X-ReID: Cross-Instance Transformer for Identity-Level Person Re-Identification
arxiv(2023)
摘要
Currently, most existing person re-identification methods use Instance-Level features, which are extracted only from a single image. However, these Instance-Level features can easily ignore the discriminative information due to the appearance of each identity varies greatly in different images. Thus, it is necessary to exploit Identity-Level features, which can be shared across different images of each identity. In this paper, we propose to promote Instance-Level features to Identity-Level features by employing cross-attention to incorporate information from one image to another of the same identity, thus more unified and discriminative pedestrian information can be obtained. We propose a novel training framework named X-ReID. Specifically, a Cross Intra-Identity Instances module (IntraX) fuses different intra-identity instances to transfer Identity-Level knowledge and make Instance-Level features more compact. A Cross Inter-Identity Instances module (InterX) involves hard positive and hard negative instances to improve the attention response to the same identity instead of different identity, which minimizes intra-identity variation and maximizes inter-identity variation. Extensive experiments on benchmark datasets show the superiority of our method over existing works. Particularly, on the challenging MSMT17, our proposed method gains 1.1% mAP improvements when compared to the second place.
更多查看译文
AI 理解论文
溯源树
样例
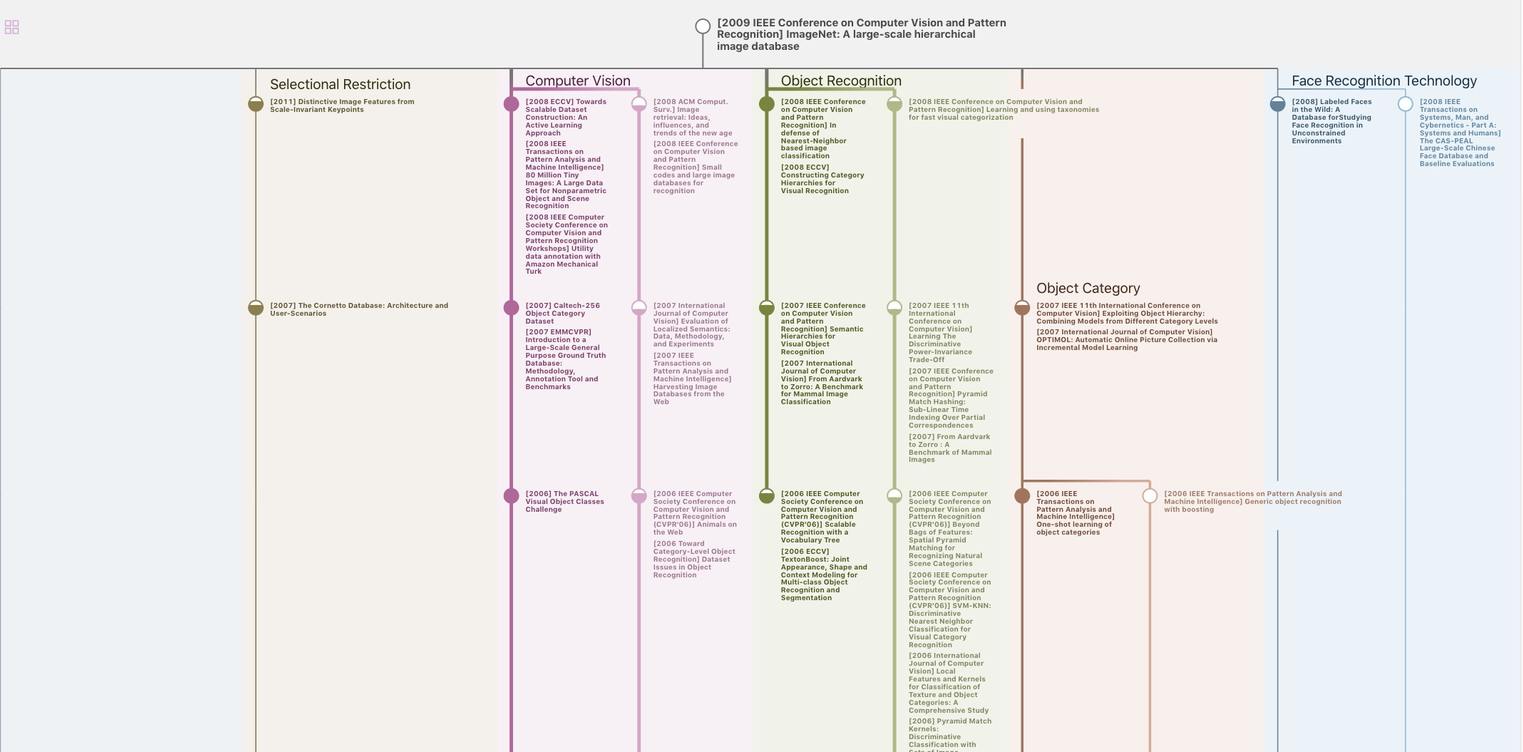
生成溯源树,研究论文发展脉络
Chat Paper
正在生成论文摘要