PINN Training using Biobjective Optimization: The Trade-off between Data Loss and Residual Loss
arxiv(2023)
摘要
Physics informed neural networks (PINNs) have proven to be an efficient tool to represent problems for which measured data are available and for which the dynamics in the data are expected to follow some physical laws. In this paper, we suggest a multiobjective perspective on the training of PINNs by treating the data loss and the residual loss as two individual objective functions in a truly biobjective optimization approach. As a showcase example, we consider COVID-19 predictions in Germany and built an extended susceptibles-infected-recovered (SIR) model with additionally considered leaky-vaccinated and hospitalized populations (SVIHR model) to model the transition rates and to predict future infections. SIR-type models are expressed by systems of ordinary differential equations (ODEs). We investigate the suitability of the generated PINN for COVID-19 predictions and compare the resulting predicted curves with those obtained by applying the method of non-standard finite differences to the system of ODEs and initial data. The approach is applicable to various systems of ODEs that define dynamical regimes. Those regimes do not need to be SIR-type models, and the corresponding underlying data sets do not have to be associated with COVID-19.
更多查看译文
关键词
pinn training,biobjective optimization,data loss,trade-off
AI 理解论文
溯源树
样例
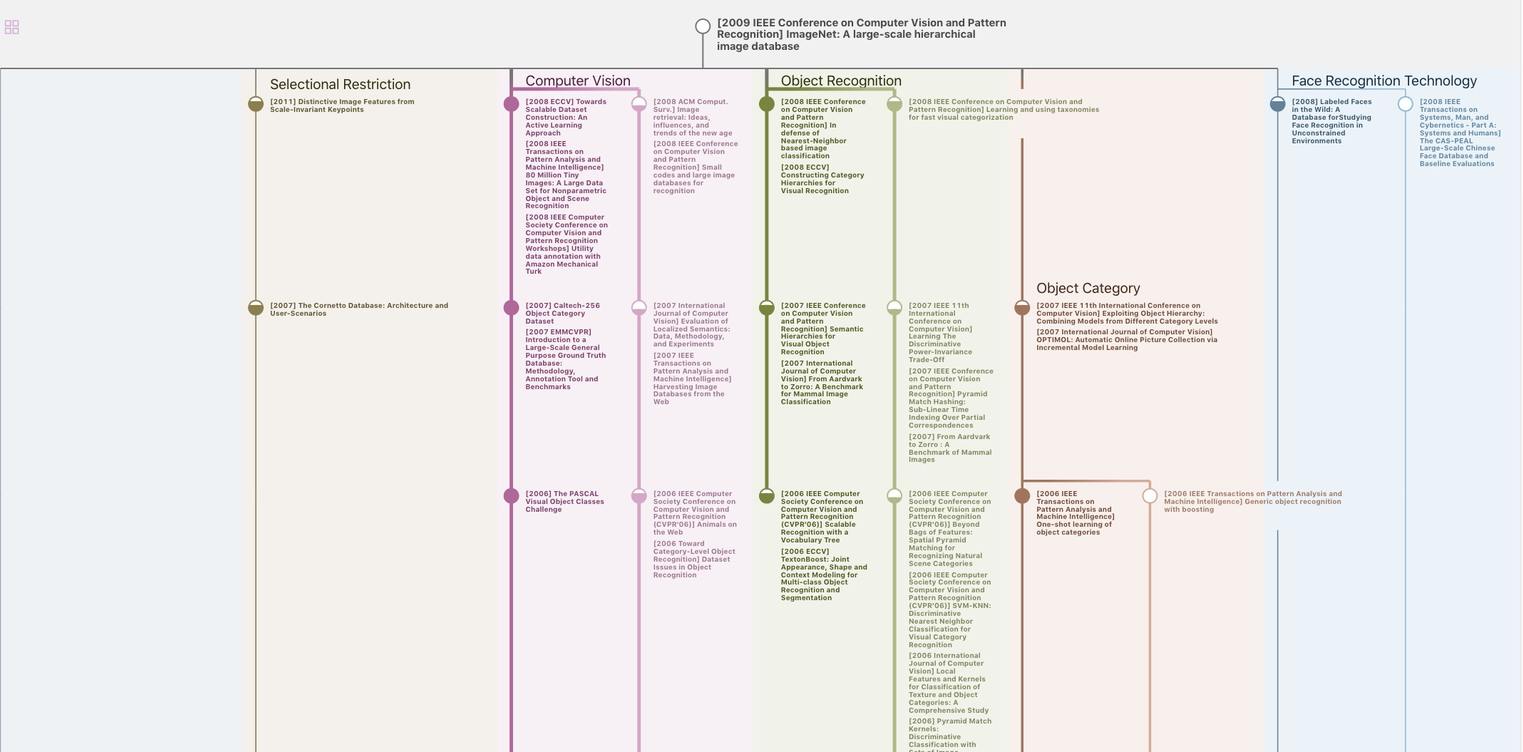
生成溯源树,研究论文发展脉络
Chat Paper
正在生成论文摘要