Learning to Decouple Complex Systems
CoRR(2023)
摘要
A complex system with cluttered observations may be a coupled mixture of multiple simple sub-systems corresponding to latent entities. Such sub-systems may hold distinct dynamics in the continuous-time domain; therein, complicated interactions between sub-systems also evolve over time. This setting is fairly common in the real world but has been less considered. In this paper, we propose a sequential learning approach under this setting by decoupling a complex system for handling irregularly sampled and cluttered sequential observations. Such decoupling brings about not only subsystems describing the dynamics of each latent entity but also a meta-system capturing the interaction between entities over time. Specifically, we argue that the meta-system evolving within a simplex is governed by projected differential equations (ProjDEs). We further analyze and provide neural-friendly projection operators in the context of Bregman divergence. Experimental results on synthetic and real-world datasets show the advantages of our approach when facing complex and cluttered sequential data compared to the state-of-the-art.
更多查看译文
关键词
neural differential equation,sequential learning,decoupling complex system
AI 理解论文
溯源树
样例
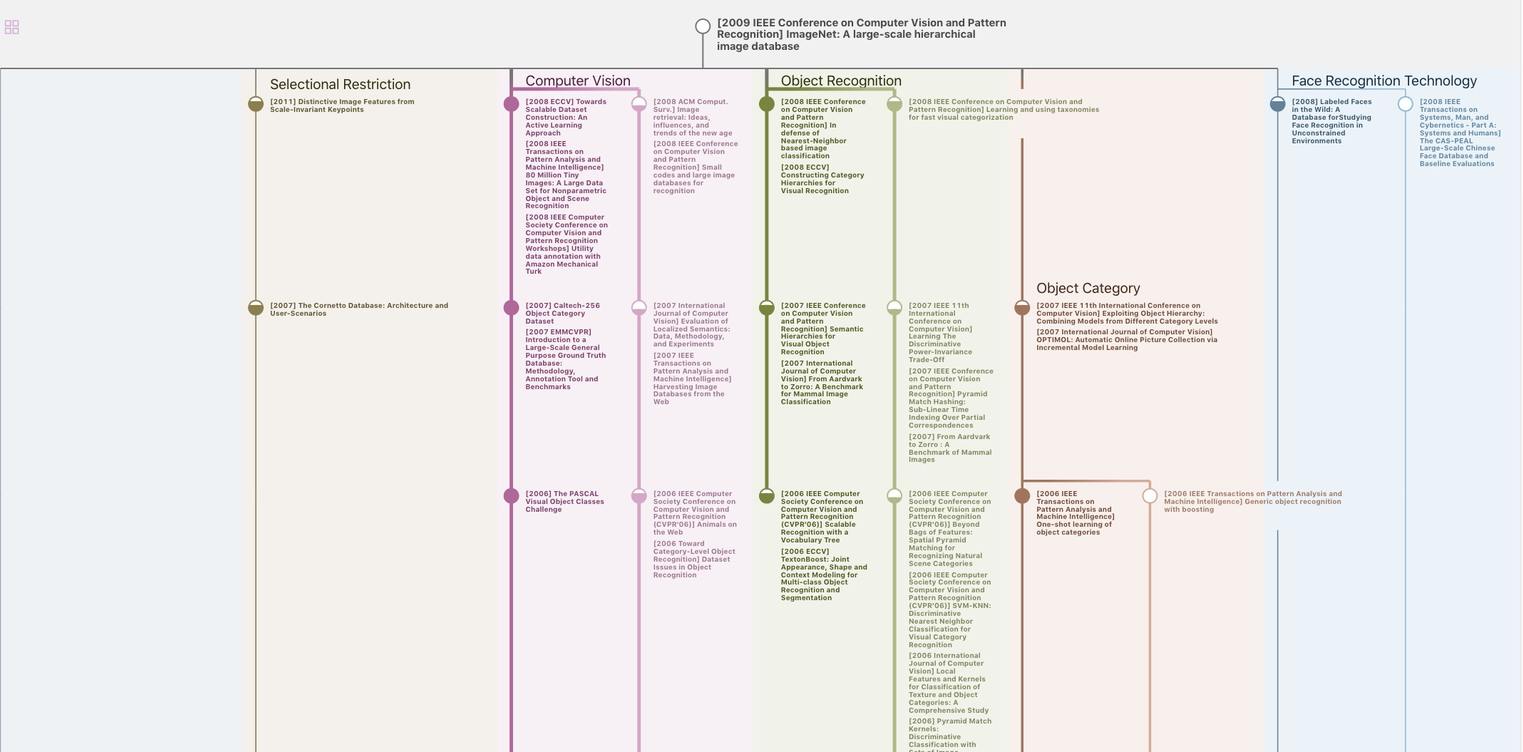
生成溯源树,研究论文发展脉络
Chat Paper
正在生成论文摘要