Between therapy effect and false-positive result in animal experimentation
BIOMEDICINE & PHARMACOTHERAPY(2023)
摘要
Despite the animal models' complexity, researchers tend to reduce the number of animals in experiments for expenses and ethical concerns. This tendency makes the risk of false-positive results, as statistical significance, the primary criterion to validate findings, often fails if testing small samples. This study aims to highlight such risks using an example from experimental regenerative therapy and propose a machine-learning solution to validate treatment effects. The example analysed was the pharmacological treatment of ear pinna punch wound healing in mice. Wound closure data analysed included eight groups treated with an epigenetic inhibitor, zebularine, and eight control groups receiving vehicle alone, of six mice each. We confirmed the zebularine healing effect for all 64 pairwise comparisons between treatment and control groups but also determined minor yet statistically significant differences between control groups in five of 28 possible comparisons. The occur-rences of significant differences between the control groups, regardless of standardised experimental conditions, indicate a risk of statistically significant effects in the case a compound lacking the desired biological activity is tested. Since the criterion of statistical significance itself can be confusing, we demonstrate a machine-learning algorithm trained on datasets representing treatment and control experiments as a helpful tool for validating treatment outcomes. We tested two machine-learning approaches, Naive Bayes and Support Vector Machine classifiers. In contrast to the Mann-Whitney U-test, indicating enhanced healing effects for some control groups receiving saline alone, both machine-learning algorithms faultlessly assigned all animal groups receiving saline to the controls.
更多查看译文
关键词
Animal experimentation, pharmacoregeneration,False-positive results,Machine-learning,Naïve Bayes classifier,Sample size,Statistical significance,Support Vector Machine classifier
AI 理解论文
溯源树
样例
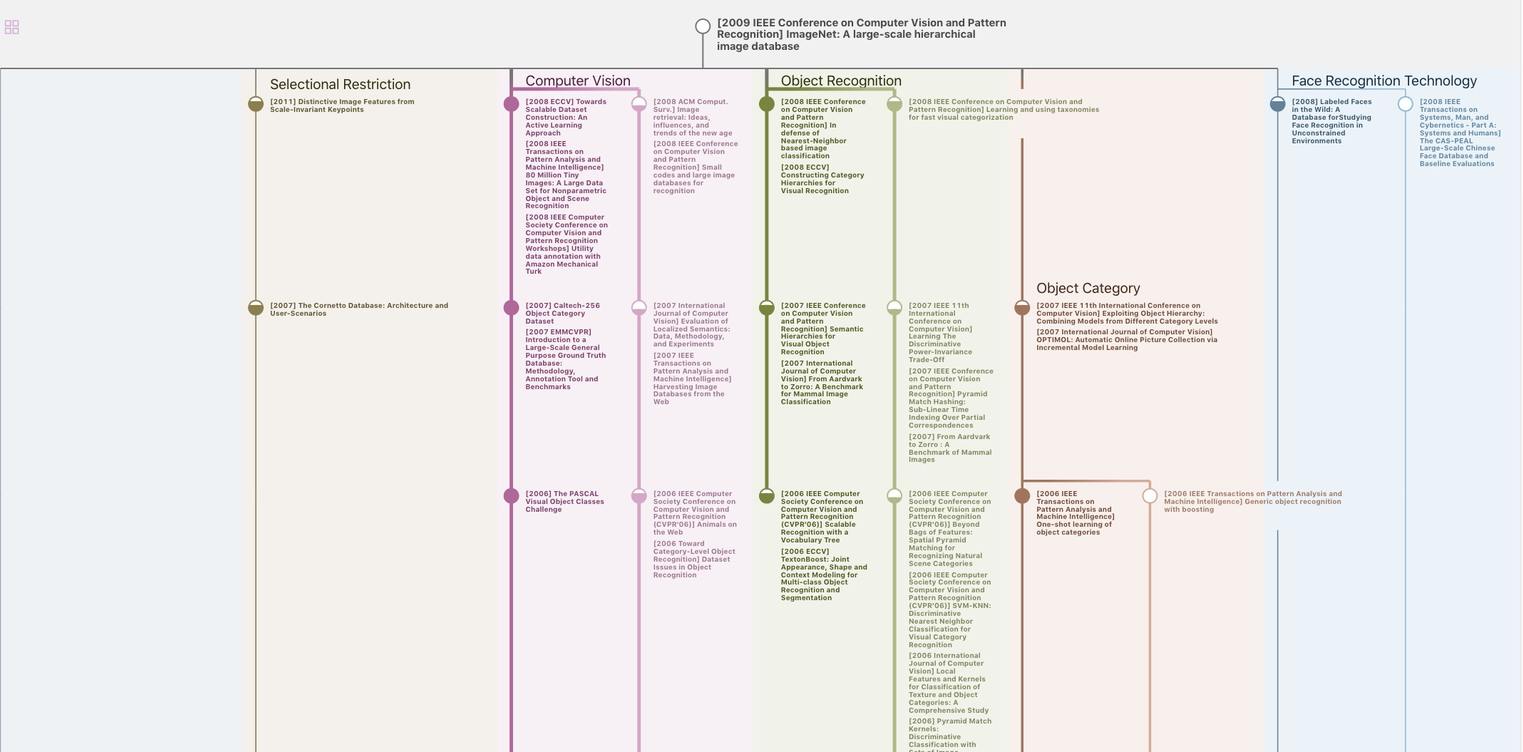
生成溯源树,研究论文发展脉络
Chat Paper
正在生成论文摘要