Machine learning-based prediction for outcomes of cardiac arrest in intensive care units: Model Development and Validation Study (Preprint)
crossref(2023)
Abstract
BACKGROUND Cardiac arrest (CA) is a global public health challenge. Accurate prediction of outcomes is a critical aspect of the management of patients after CA. This study will develop and validate an applicable machine learning (ML) model to predict in-hospital mortality of CA in intensive care units. This study will develop and validate an machine learning (ML) model to predict in-hospital mortality of CA in ICU. OBJECTIVE The study aims to develop and validate machine learning models to predict in-hospital mortality of CA in ICU. METHODS Patients with CA were extracted from the Medical Information Mart for Intensive Care (MIMIC)-IV database, and further divided into the training set (80%) and validation set (20%). The primary outcome was in-hospital mortality. The best model was selected from 11 ML algorithms and 3 time periods of 24 hours, 48 hours, and 72 hours. SHapley Additive exPlanations (SHAP) was applied to visualize the importance of features, while recursive feature elimination (RFE) was performed to figure out key features. The optimal compact model was developed based on selected key features, and its performance was proven based on the validation set. In addition, a Web-based tool was designed to applied this model to clinical practice. RESULTS 721 patients were included in this study, dividing into the training set (80%, n=576) and the validation set (20%, n=145). The 72-hour CatBoost model obtained the highest area under the receiver operating characteristic (AUROC) of 0.839. Thirteen variables were ultimately selected as key features, and the importance of each feature was visualized by SHAP. The compact model achieved the greatest AUROC of 0.862 in validation, which better than other models and SOFA (AUROC: 0.650). The Web-based tool was convenient for clinicians to use our model. CONCLUSIONS CatBoost model had great prediction performance of in-hospital mortality in CA patients.
MoreTranslated text
AI Read Science
Must-Reading Tree
Example
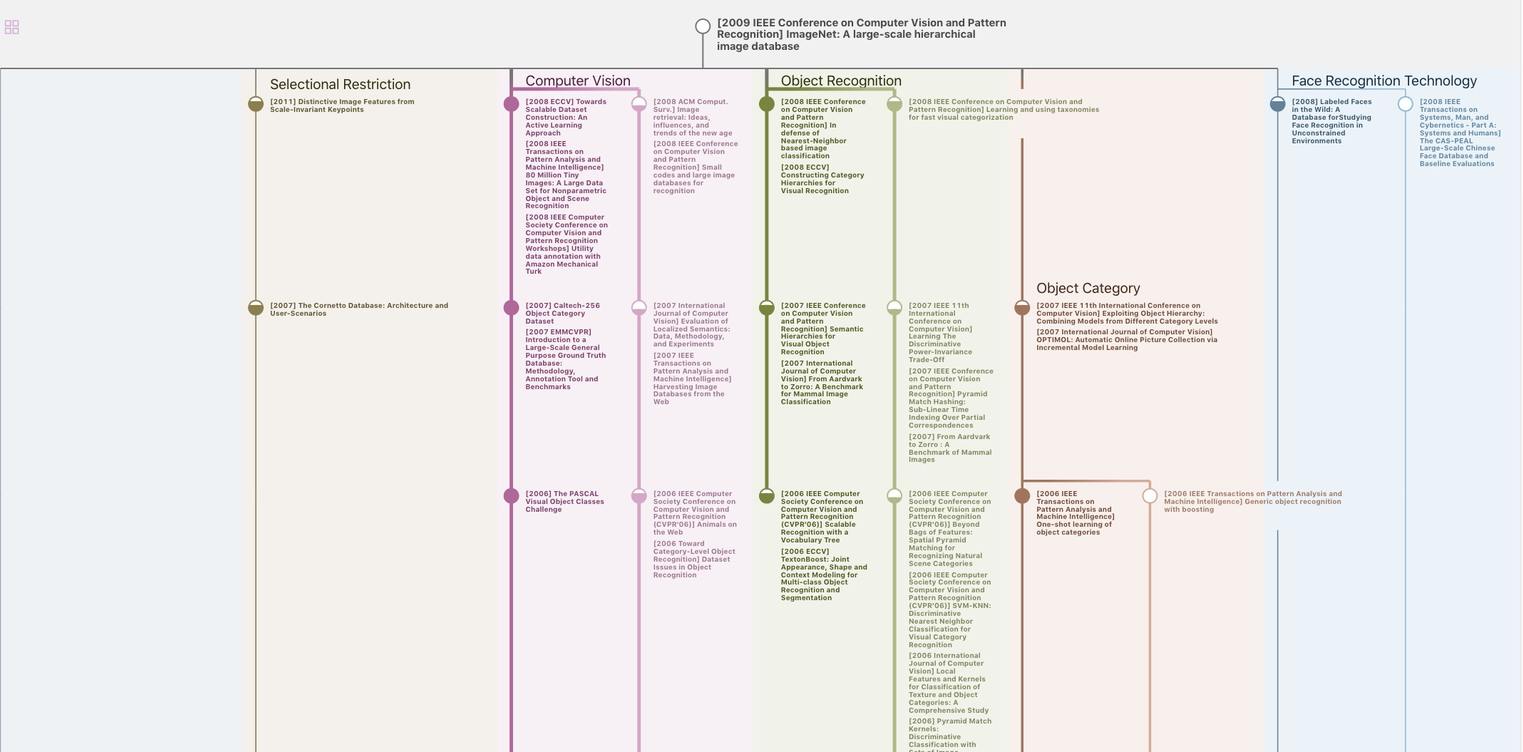
Generate MRT to find the research sequence of this paper
Chat Paper
Summary is being generated by the instructions you defined