Automatic Classification of C-SAM Voids for Root Cause Identification of Bonding Yield Degradation
2022 International Symposium on Semiconductor Manufacturing (ISSM)(2023)
摘要
Wafer-level direct bonding technology is a key process for the production of backside illuminated (BSI) CMOS image sensor (CIS). Usually, constant-depth mode scanning acoustic microscope (C-SAM) 300mm wafer images are acquired and defect size distribution is provided to monitor defects that degrade bonding yield. This acquisition technique is used as it is nondestructive and is able to capture the full wafer. Current solutions are not providing information detailed enough to identify the root cause of this degradation. A consequence is that most measurements and classification is currently done manually which take time and have reproducibility issues. In this paper, we propose a rule-based method for the classification of the defects and automatic segmentation of the defects to extract precise measurements depending on the type of defect. The classes of defects are precisely defined based on their origin in the process. All these information will allow to reduce the time to analyze the images and improve the precision and consistency of the analysis. These results will allow to identify more precisely the defects thus the causes of yield degradation during bonding process.
更多查看译文
关键词
defects,classification,segmentation,voids
AI 理解论文
溯源树
样例
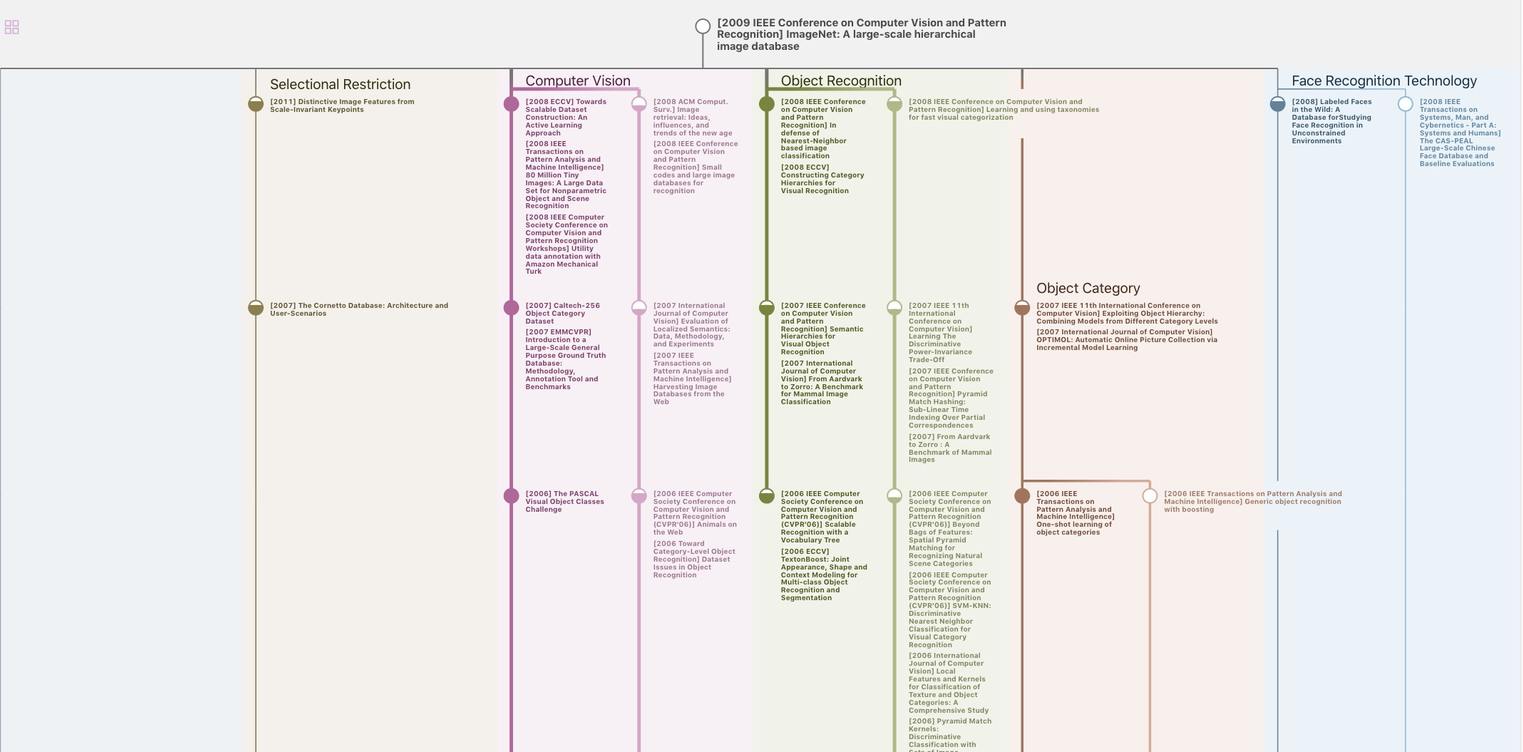
生成溯源树,研究论文发展脉络
Chat Paper
正在生成论文摘要