Schizo-Net: A novel Schizophrenia Diagnosis Framework Using Late Fusion Multimodal Deep Learning on Electroencephalogram-Based Brain Connectivity Indices
IEEE Transactions on Neural Systems and Rehabilitation Engineering(2023)
摘要
Schizophrenia (SCZ) is a serious mental condition that causes hallucinations, delusions, and disordered thinking. Traditionally, SCZ diagnosis involves the subject’s interview by a skilled psychiatrist. The process needs time and is bound to human errors and bias. Recently, brain connectivity indices have been used in a few pattern recognition methods to discriminate neuro-psychiatric patients from healthy subjects. The study presents
$\textit {Schizo-Net}$
, a novel, highly accurate, and reliable SCZ diagnosis model based on a late multimodal fusion of estimated brain connectivity indices from EEG activity. First, the raw EEG activity is pre-processed exhaustively to remove unwanted artifacts. Next, six brain connectivity indices are estimated from the windowed EEG activity, and six different deep learning architectures (with varying neurons and hidden layers) are trained. The present study is the
$\textit {first}$
which considers a large number of brain connectivity indices, especially for SCZ. A detailed study was also performed that identifies SCZ-related changes occurring in brain connectivity, and the vital significance of BCI is drawn in this regard to identify the biomarkers of the disease.
$\textit {Schizo-Net}$
surpasses current models and achieves 99.84% accuracy. An optimum deep learning architecture selection is also performed for improved classification. The study also establishes that Late fusion technique outperforms single architecture-based prediction in diagnosing SCZ.
更多查看译文
关键词
Schizophrenia,deep learning,brain connectivity features,feature fusion,classification
AI 理解论文
溯源树
样例
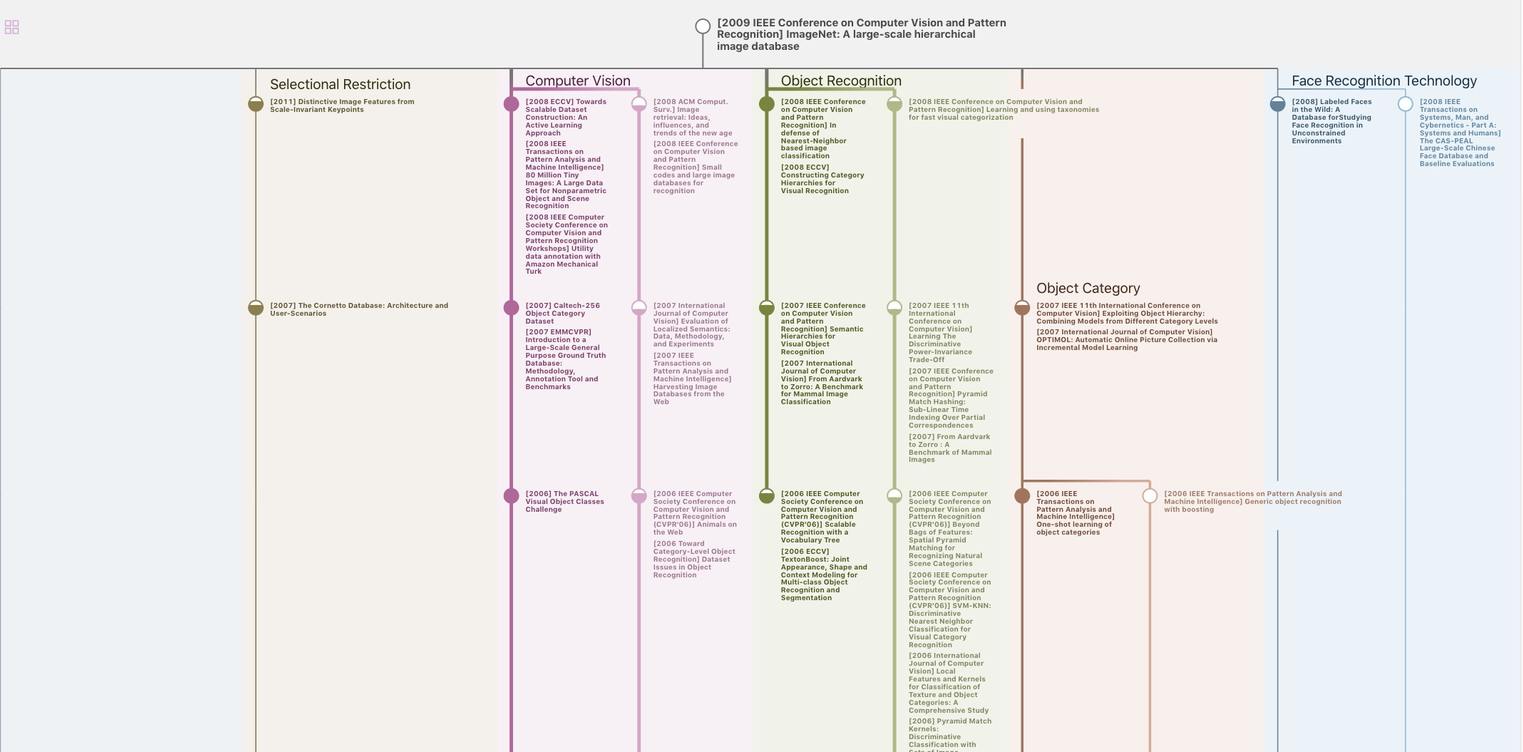
生成溯源树,研究论文发展脉络
Chat Paper
正在生成论文摘要