3D Neural Embedding Likelihood for Robust Sim-to-Real Transfer in Inverse Graphics
ICLR 2023(2023)
摘要
A central challenge in 3D scene perception via inverse graphics is robustly modeling the gap between 3D graphics and real-world data. We propose a novel 3D Neural Embedding Likelihood (3DNEL) over RGB-D images to address this gap. 3DNEL uses neural embeddings to predict 2D-3D correspondences from RGB and combines this with depth in a principled manner. 3DNEL is trained entirely from synthetic images and generalizes to real-world data. To showcase this capability, we develop a multi-stage inverse graphics pipeline that uses 3DNEL for 6D object pose estimation from real RGB-D images. Our method outperforms the previous state-of-the-art in sim-to-real pose estimation on the YCB-Video dataset, and improves robustness, with significantly fewer large-error predictions. Unlike existing bottom-up, discriminative approaches that are specialized for pose estimation, 3DNEL adopts a probabilistic generative formulation that jointly models multi-object scenes. This generative formulation enables easy extension of 3DNEL to additional tasks like object and camera tracking from video, using principled inference in the same probabilistic model without task specific retraining.
更多查看译文
关键词
3D inverse graphics,probabilistic inference,likelihood,RGB-D,neural embedding,object pose estimation
AI 理解论文
溯源树
样例
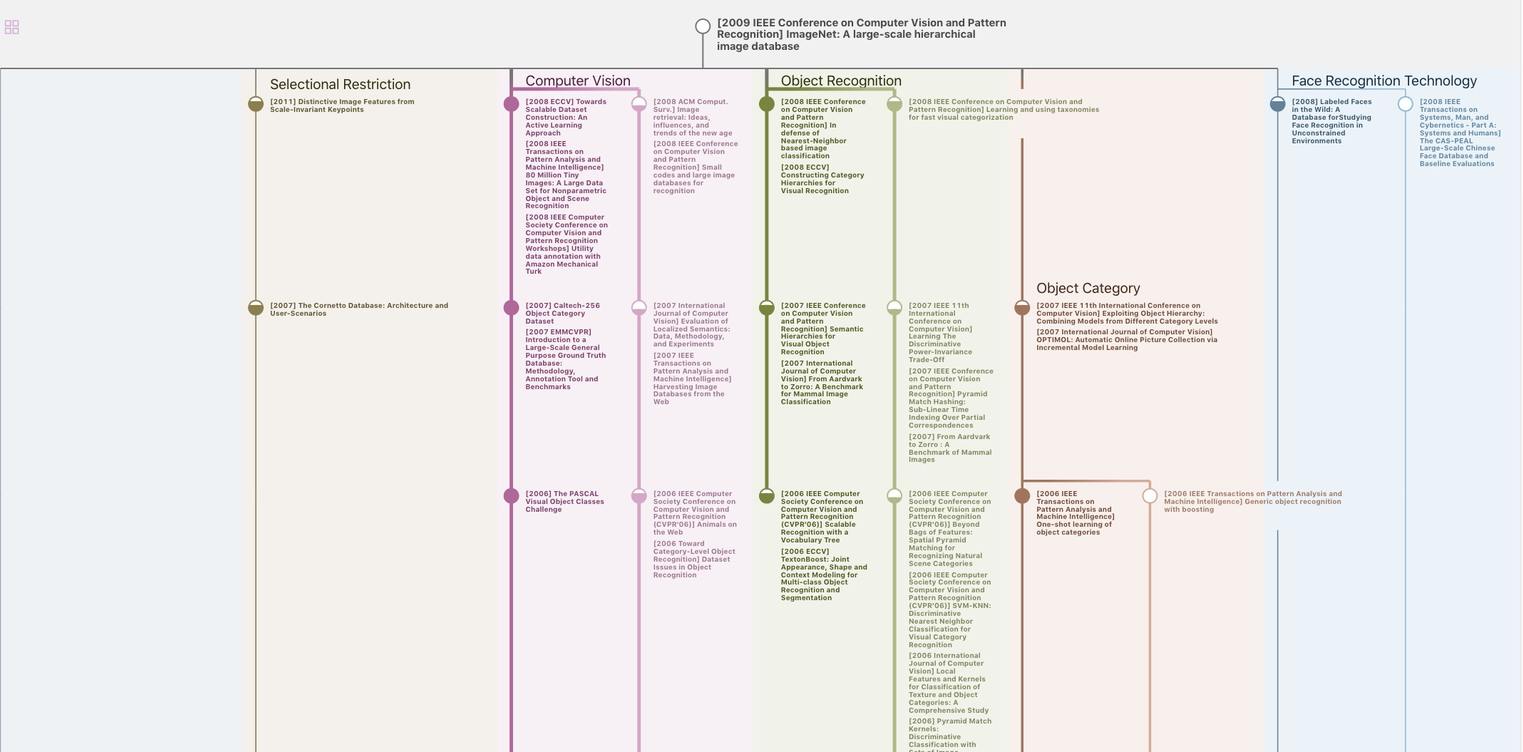
生成溯源树,研究论文发展脉络
Chat Paper
正在生成论文摘要