Masked Visual-Textual Prediction for Document Image Representation Pretraining
ICLR 2023(2023)
摘要
In this paper, we present Masked Visual-Textual Prediction for document image representation pretraining, called MaskDoc. It comprises of two self-supervised pretraining tasks: Masked Image Modeling and Masked Language Modeling, based on text region-level image masking. Our approach randomly masks some words or texts and accordingly the corresponding image regions, and the pretraining task is reconstructing the masked image regions as well as the corresponding words. In comparison to masked image modeling which usually predict the image patches or tokens, the encoder pretrained by our approach captures more textual semantics. Compared to the masked multi-modal modeling methods for document image understanding, e.g., LayoutLM and StrucTexT, that need both the image and text inputs, our approach is able to model image-only input, and potentially can deal with more application scenarios free from OCR pre-processing. We demonstrate the effectiveness of MaskDoc on several document image understanding tasks such as image classification, layout analysis, table structure recognition, document OCR, and end-to-end information extraction. Experimental results show that MaskDoc achieves state-of-the-art performance. Our code and models will be released soon.
更多查看译文
AI 理解论文
溯源树
样例
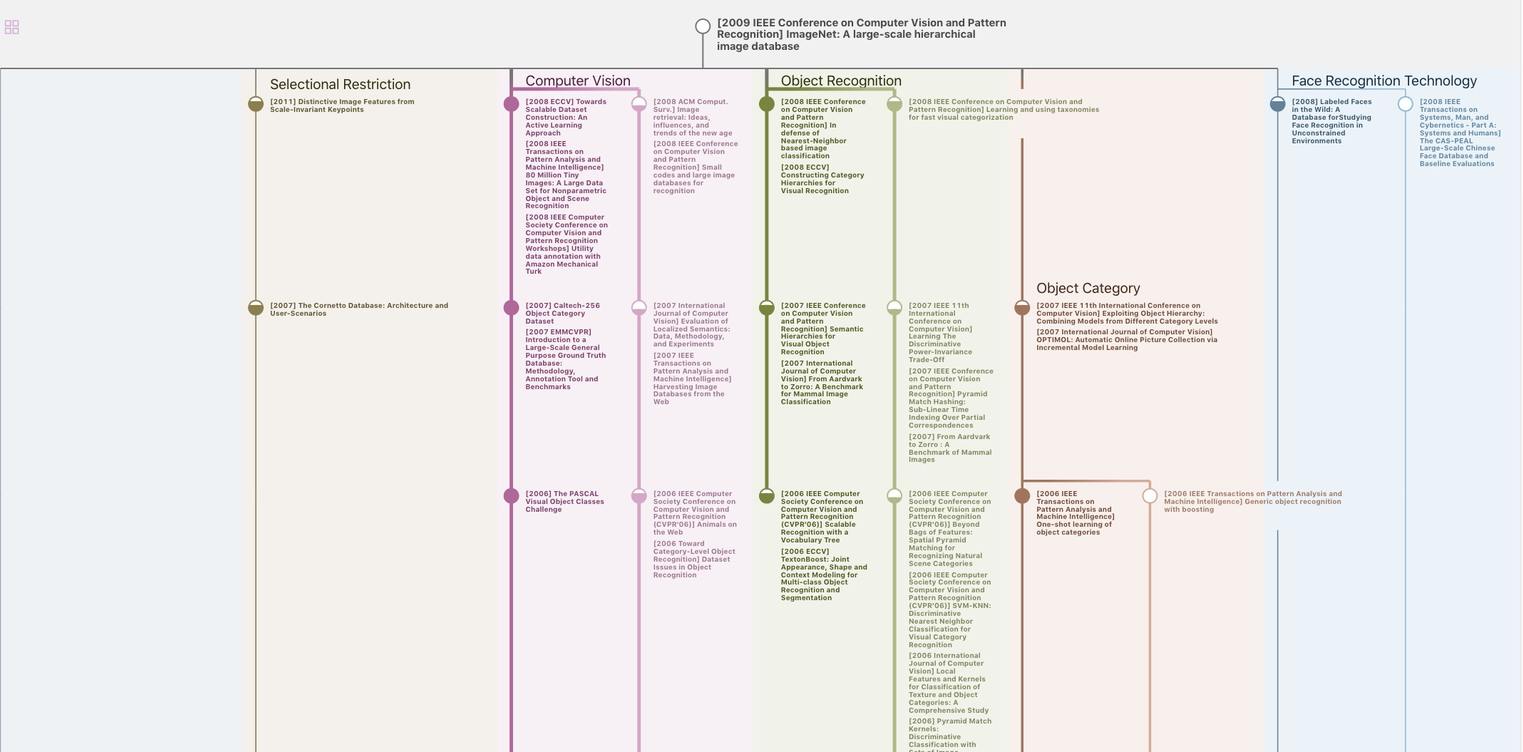
生成溯源树,研究论文发展脉络
Chat Paper
正在生成论文摘要