Causal Representation Learning for Instantaneous and Temporal Effects
ICLR 2023(2023)
摘要
Causal representation learning is the task of identifying the underlying causal variables and their relations from high-dimensional observations, such as images. Recent work has shown that one can reconstruct the causal variables from temporal sequences of observations under the assumption that there are no instantaneous causal relations between them. In practical applications, however, our measurement or frame rate might be slower than many of the causal effects. This effectively creates ``instantaneous'' effects and invalidates previous identifiability results. To address this issue, we propose iCITRIS, a causal representation learning method that allows for instantaneous effects in temporal sequences with known intervention targets. iCITRIS identifies the potentially multidimensional causal variables from temporal observations, while simultaneously using a differentiable causal discovery method to learn their causal graph. In experiments on three video datasets, iCITRIS accurately identifies the causal variables and their causal graph.
更多查看译文
关键词
Representation Learning,Causality,Causal Representation Learning,Causal Discovery,Disentanglement
AI 理解论文
溯源树
样例
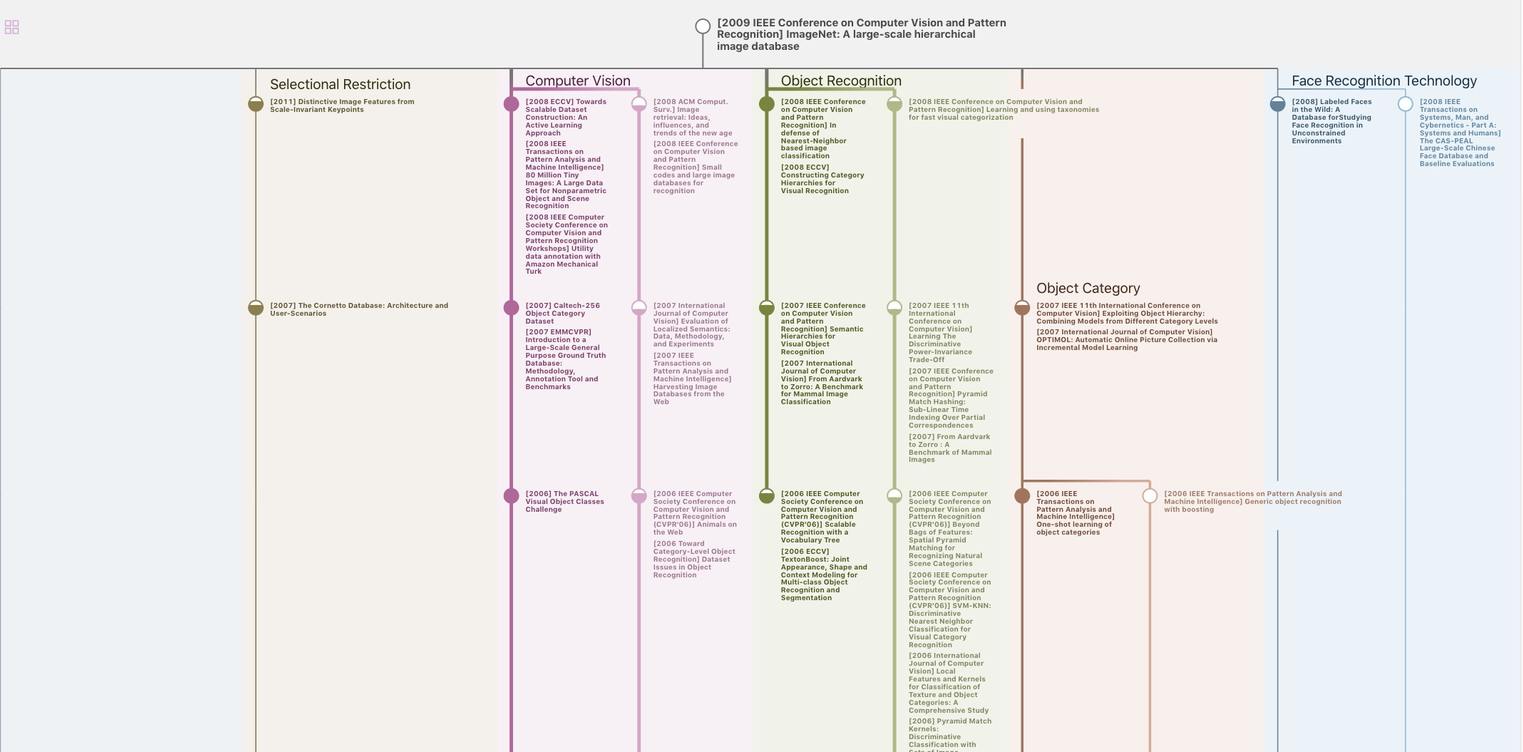
生成溯源树,研究论文发展脉络
Chat Paper
正在生成论文摘要