Eva: Practical Second-order Optimization with Kronecker-vectorized Approximation
ICLR 2023(2023)
Abstract
Second-order optimization algorithms exhibit excellent convergence properties for training deep learning models, but often incur significant computation and memory overheads. This can result in lower training efficiency than the first-order counterparts such as stochastic gradient descent (SGD). In this work, we present a memory- and time-efficient second-order algorithm named Eva with two novel techniques: 1) we construct the second-order information with the Kronecker factorization of small stochastic vectors over a mini-batch of training data to reduce memory consumption, and 2) we derive an efficient update formula without explicitly computing the inverse of matrices using the Sherman-Morrison formula. We further provide a theoretical interpretation of Eva from a trust-region optimization point of view to understand how it works. Extensive experimental results on different models and datasets show that Eva reduces the end-to-end training time up to $2.05\times$ and $2.42\times$ compared to first-order SGD and second-order algorithms (K-FAC and Shampoo), respectively.
MoreTranslated text
Key words
Deep learning,Second-order optimization,Approximation
AI Read Science
Must-Reading Tree
Example
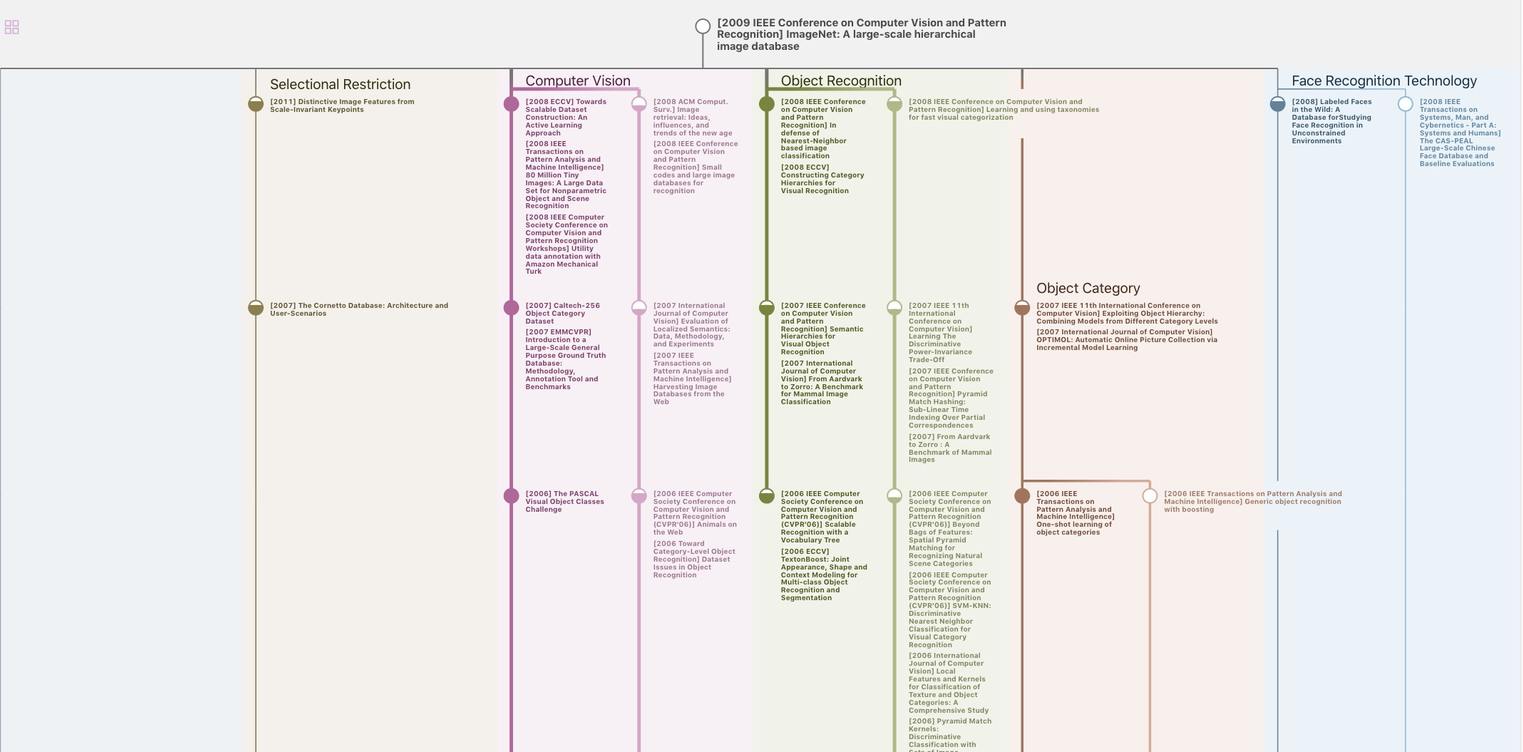
Generate MRT to find the research sequence of this paper
Chat Paper
Summary is being generated by the instructions you defined