Provable Memorization Capacity of Transformers
ICLR 2023(2023)
摘要
Quantifying memorization capacity is essential for understanding the expressiveness and generalizability of deep learning model architectures. However, the memorization capacity of the Transformer architecture has yet to be explored. In this work, we present the first study of the memorization capacity of the Transformer architecture. We prove that Transformers are capable of memorizing $N$ sequence-to-sequence mappings of length $n$ with $d$-dimensional input tokens using $\tilde{O}(d + n + \sqrt{nN})$ parameters. Our theory supports memorization both with and without permutation equivariance, utilizing positional encodings in the latter case. Building on our theory, we also analyze the memorization capacity of Transformers in the sequence classification task. To verify these theoretical findings, we conduct experiments analyzing the memorization capacity of Transformers in the natural language domain.
更多查看译文
关键词
Transformer,Expressivness,Memorization,Deep learning theory,contextual mapping,permutation equivariance
AI 理解论文
溯源树
样例
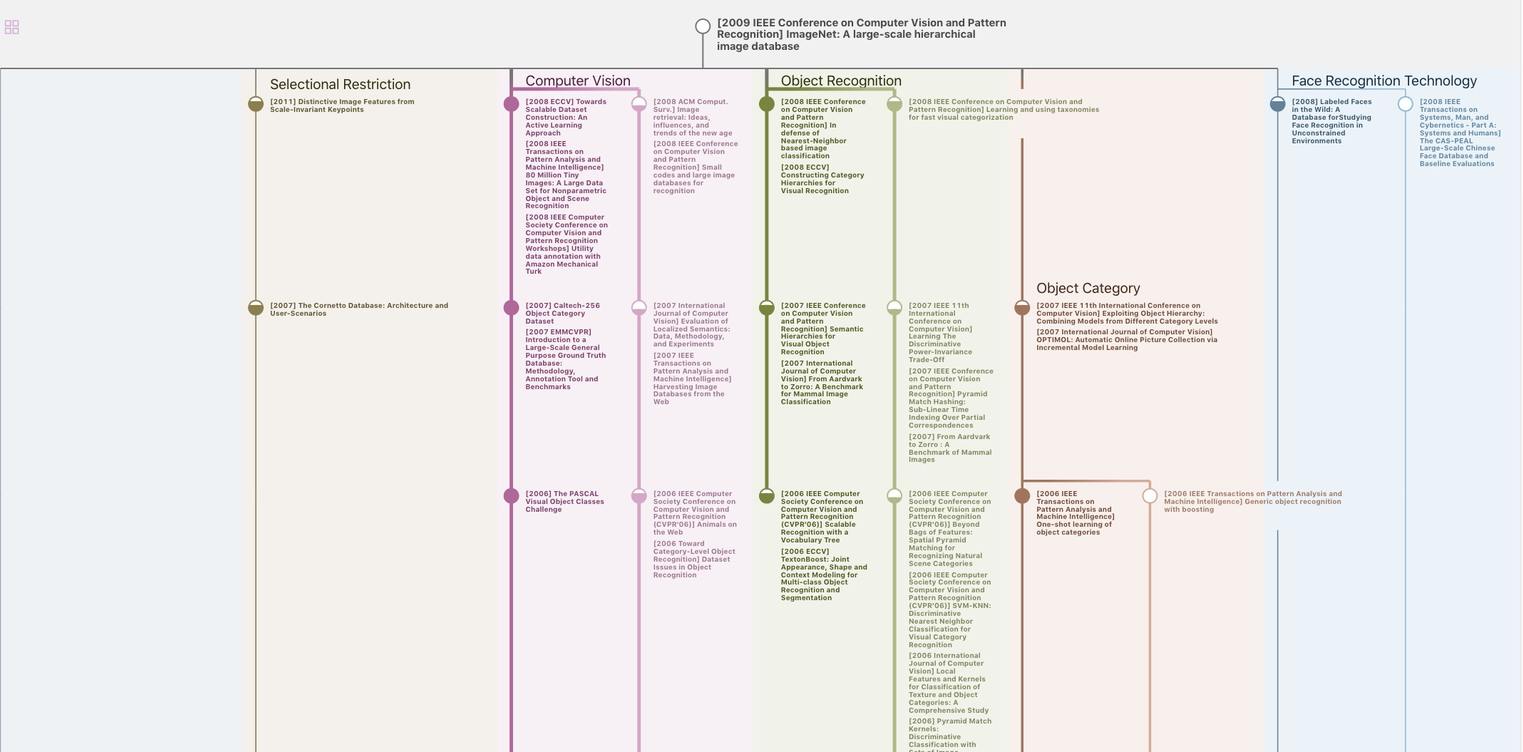
生成溯源树,研究论文发展脉络
Chat Paper
正在生成论文摘要