Budgeted Training for Vision Transformer
ICLR 2023(2023)
摘要
The superior performances of Vision Transformers often come with higher training costs. Compared to their CNN counterpart, Transformer models are hungry for large-scale data and their training schedules are usually prolonged. This sets great restrictions on training Transformers with limited resources, where a proper trade-off between training cost and model performance is longed. In this paper, we address the problem by proposing a framework that enables the training process under any training budget, while achieving competitive model performances. Specifically, based on the observation that Transformer exhibits different levels of model redundancies at different stages of training, we propose to dynamically control the activation rate of model parameters along the training process and meet the demand on the training budget by adjusting the duration on each level of model complexity. Extensive experiments demonstrate that our framework is applicable to various Vision Transformers, and achieves competitive performances on a wide range of training budgets.
更多查看译文
AI 理解论文
溯源树
样例
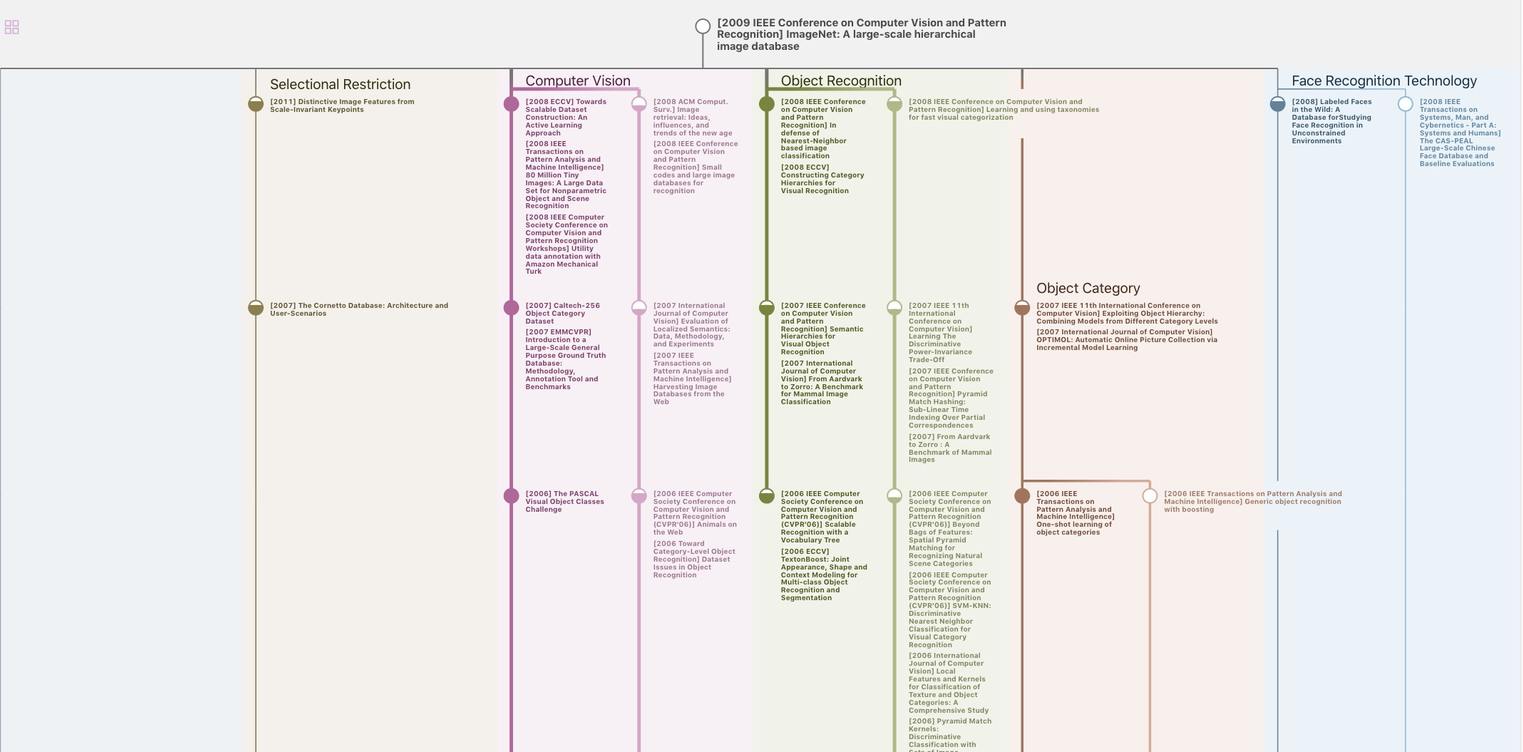
生成溯源树,研究论文发展脉络
Chat Paper
正在生成论文摘要