The Implicit Bias of Minima Stability in Multivariate Shallow ReLU Networks
ICLR 2023(2023)
摘要
We study the type of solutions to which stochastic gradient descent converges when used to train a single hidden-layer multivariate ReLU network with the quadratic loss. Our results are based on a dynamical stability analysis. In the univariate case, it was shown that linearly stable minima correspond to network functions (predictors) $f$, whose second derivative has a bounded weighted $L^1$ norm. The bound on the norm gets smaller as the step size increases, implying that training with a large step size leads to `smoother' predictors. Here we generalize this result to the multivariate case, showing that a similar result applies to the Laplacian of the predictor, $\Delta f$. We demonstrate the tightness of our bound on the MNIST dataset, and show that it accurately captures the behavior of the solutions as a function of the step size. Additionally, we prove a depth separation result on the approximation power of ReLU networks corresponding to stable minima of the loss. Specifically, although shallow ReLU networks are universal approximators, we prove that stable shallow networks are not. Namely, there is a function that cannot be well-approximated by stable single hidden-layer ReLU networks trained with a non-vanishing step size. In contrast, we show that the same function can be realized as a stable two hidden-layer ReLU network. Finally, we prove that if a function is sufficiently smooth (Sobolev) then it can be approximated arbitrarily well using single hidden-layer ReLU networks that correspond to stable solutions of gradient descent.
更多查看译文
关键词
Implicit bias,implicit regularization,stability,Hessian,dynamical systems,depth separation,approximation
AI 理解论文
溯源树
样例
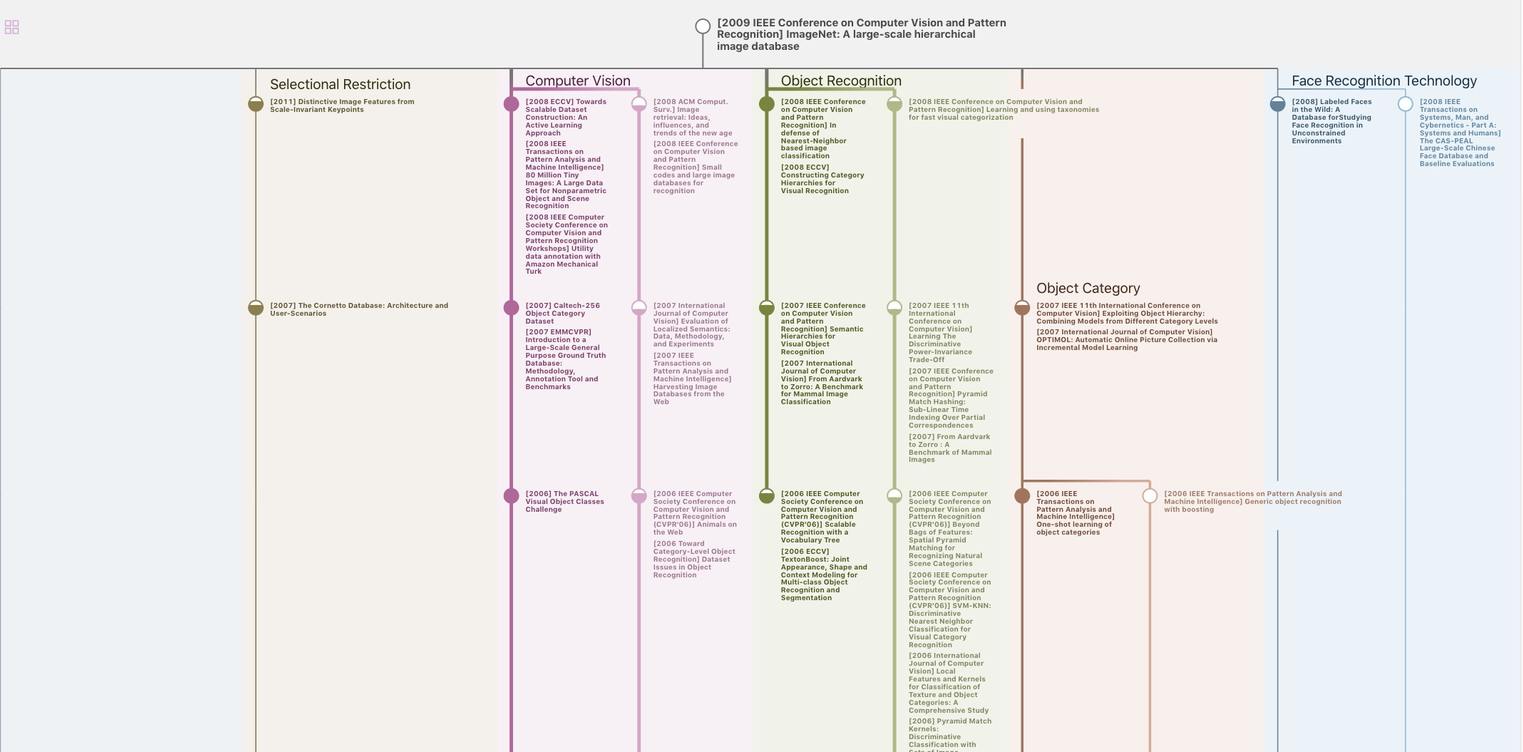
生成溯源树,研究论文发展脉络
Chat Paper
正在生成论文摘要