How gradient estimator variance and bias impact learning in neural networks
ICLR 2023(2023)
Abstract
There is growing interest in understanding how real brains may approximate gradients and how gradients can be used to train neuromorphic chips. However, neither real brains nor neuromorphic chips can perfectly follow the loss gradient, so parameter updates would necessarily use gradient estimators that have some variance and/or bias. Therefore, there is a need to understand better how variance and bias in gradient estimators impact learning dependent on network and task properties. Here, we show that variance and bias can impair learning on the training data, but some degree of variance and bias in a gradient estimator can be beneficial for generalization. We find that the ideal amount of variance and bias in a gradient estimator are dependent on several properties of the network and task: the size and sparsity of the network, the norm of the gradient, and the curvature of the loss landscape. As such, whether considering biologically-plausible learning algorithms or algorithms for training neuromorphic chips, researchers can analyze these properties to determine whether their approximation to gradient descent will be effective for learning given their network and task properties.
MoreTranslated text
Key words
Computational Neuroscience,learning and plasticity,Credit assignment,Imperfect gradient descent,Gradient approximation,Biologically-plausible learning,Neuromorphic computing,Neural networks
AI Read Science
Must-Reading Tree
Example
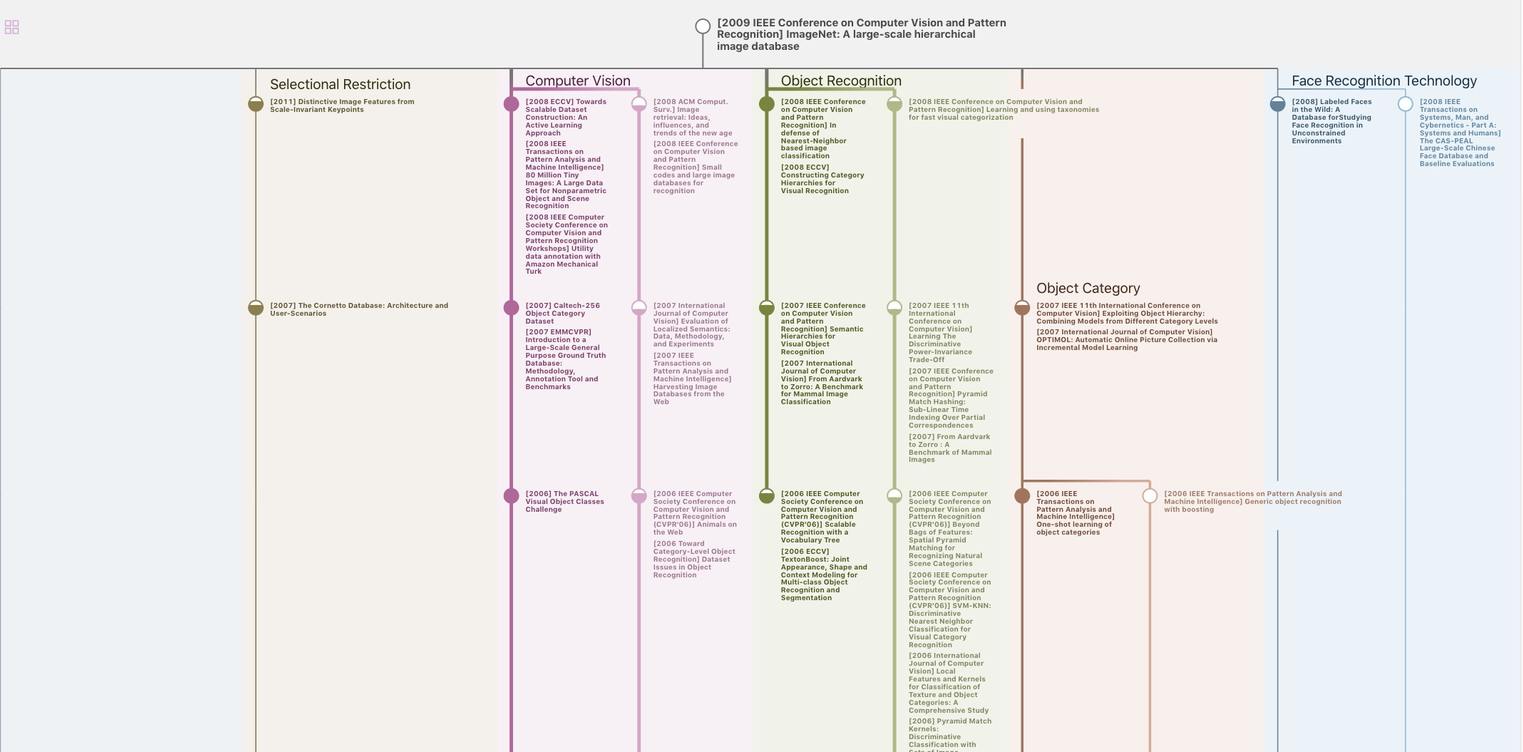
Generate MRT to find the research sequence of this paper
Chat Paper
Summary is being generated by the instructions you defined