On Achieving Optimal Adversarial Test Error
ICLR 2023(2023)
摘要
We first elucidate various fundamental properties of optimal adversarial predictors: the structure of optimal adversarial convex predictors in terms of optimal adversarial zero-one predictors, bounds relating the adversarial convex loss to the adversarial zero-one loss, and the fact that continuous predictors can get arbitrarily close to the optimal adversarial error for both convex and zero-one losses. Applying these results along with new Rademacher complexity bounds for adversarial training near initialization, we prove that for general data distributions and perturbation sets, adversarial training on shallow networks with early stopping and an idealized optimal adversary is able to achieve optimal adversarial test error. By contrast, prior theoretical work either considered specialized data distributions or only provided training error guarantees.
更多查看译文
关键词
test
AI 理解论文
溯源树
样例
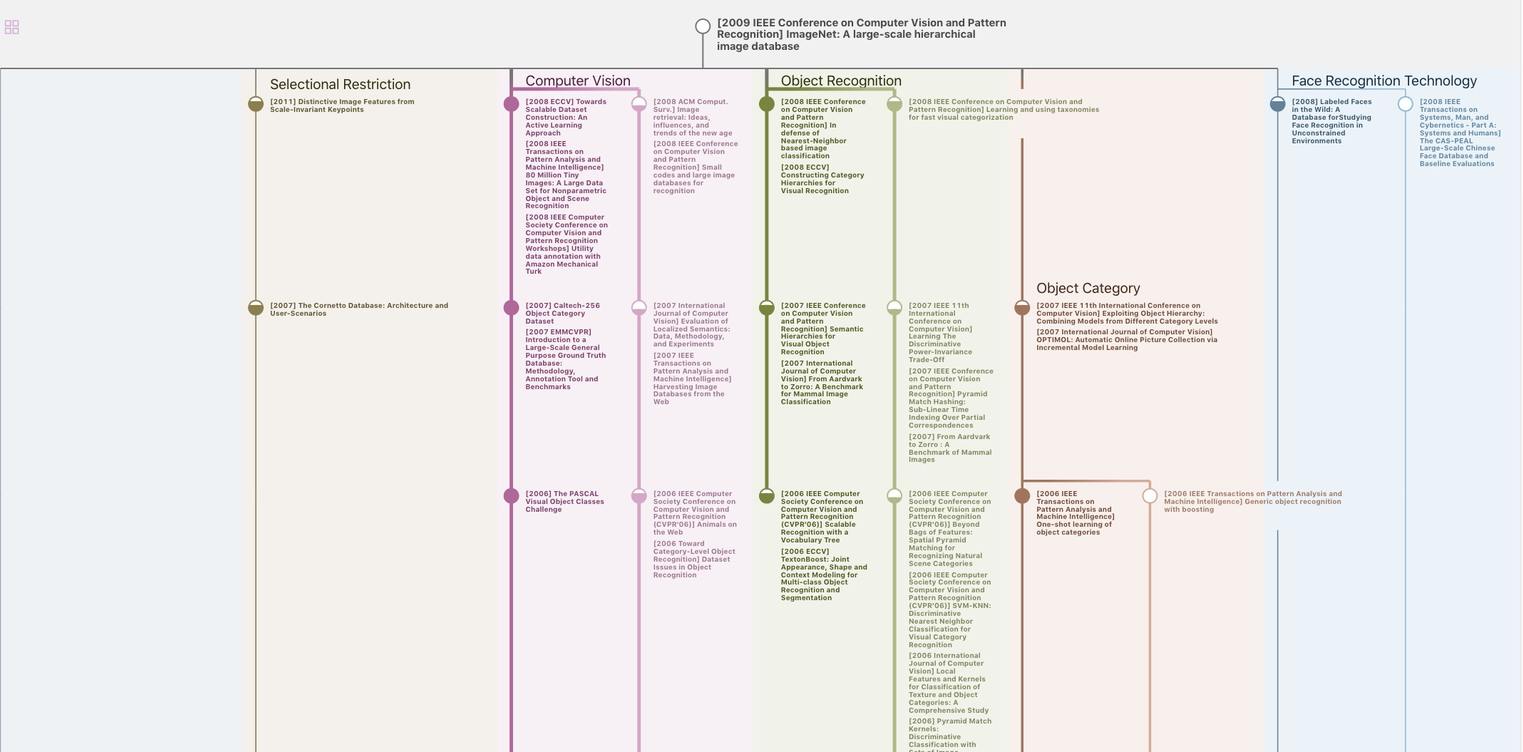
生成溯源树,研究论文发展脉络
Chat Paper
正在生成论文摘要