Learning Iterative Neural Optimizers for Image Steganography
ICLR 2023(2023)
摘要
Image steganography is the process of concealing secret information in images through imperceptible changes. Recent work has formulated this task as a classic constrained optimization problem. In this paper, we argue that image steganography is inherently performed on the (elusive) manifold of natural images, and propose an iterative neural network trained to perform the optimization steps. In contrast to classical optimization methods like L-BFGS or projected gradient descent, we train the neural network to also stay close to the manifold of natural images throughout the optimization. We show that our learned neural optimization is faster and more reliable than classical optimization approaches. In comparison to previous state-of-the-art encoder-decoder-based steganography methods, it reduces the recovery error rate by multiple orders of magnitude and achieves zero error up to 3 bits per pixel (bpp) without the need for error-correcting codes.
更多查看译文
关键词
iterative neural optimizers,image steganography,learning
AI 理解论文
溯源树
样例
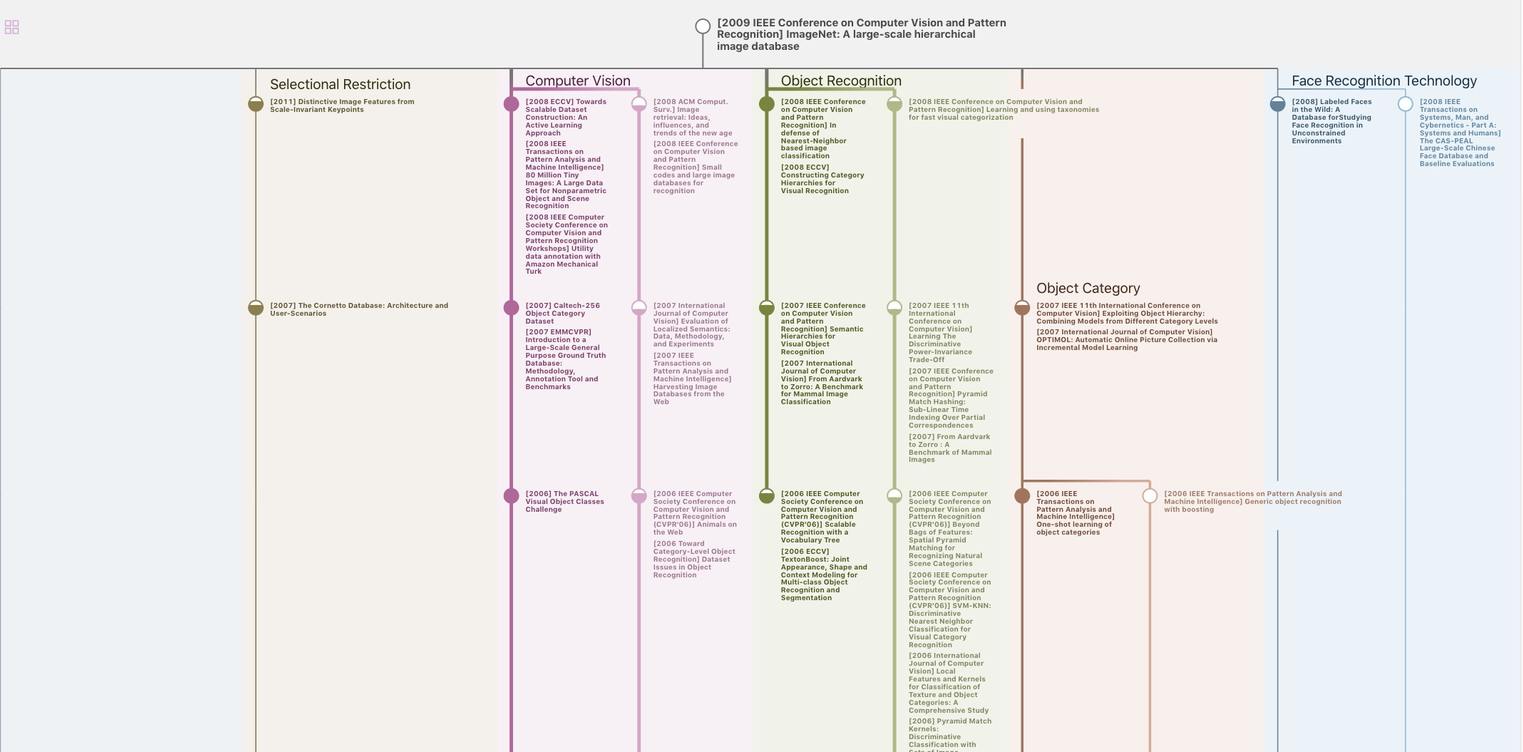
生成溯源树,研究论文发展脉络
Chat Paper
正在生成论文摘要