Information-Theoretic Diffusion
ICLR 2023(2023)
摘要
Denoising diffusion models have spurred significant gains in density modeling and image generation, precipitating an industrial revolution in text-guided AI art generation. Whether interpreted through the lens of variational models or differential equations, diffusion models require many steps of expensive computation to give accurate density estimates. We introduce a new mathematical foundation for diffusion models inspired by classic results in information theory that connects Information with Minimum Mean Square Error estimators, the so-called I-MMSE relations. We generalize the I-MMSE relations to \emph{exactly} relate the data distribution and optimal denoising, leading to an elegant refinement of existing diffusion bounds. This new insight improves density estimation for diffusion models and enables simultaneous modeling of both continuous and discrete probabilities with no additional cost.
更多查看译文
关键词
diffusion,density models,information theory
AI 理解论文
溯源树
样例
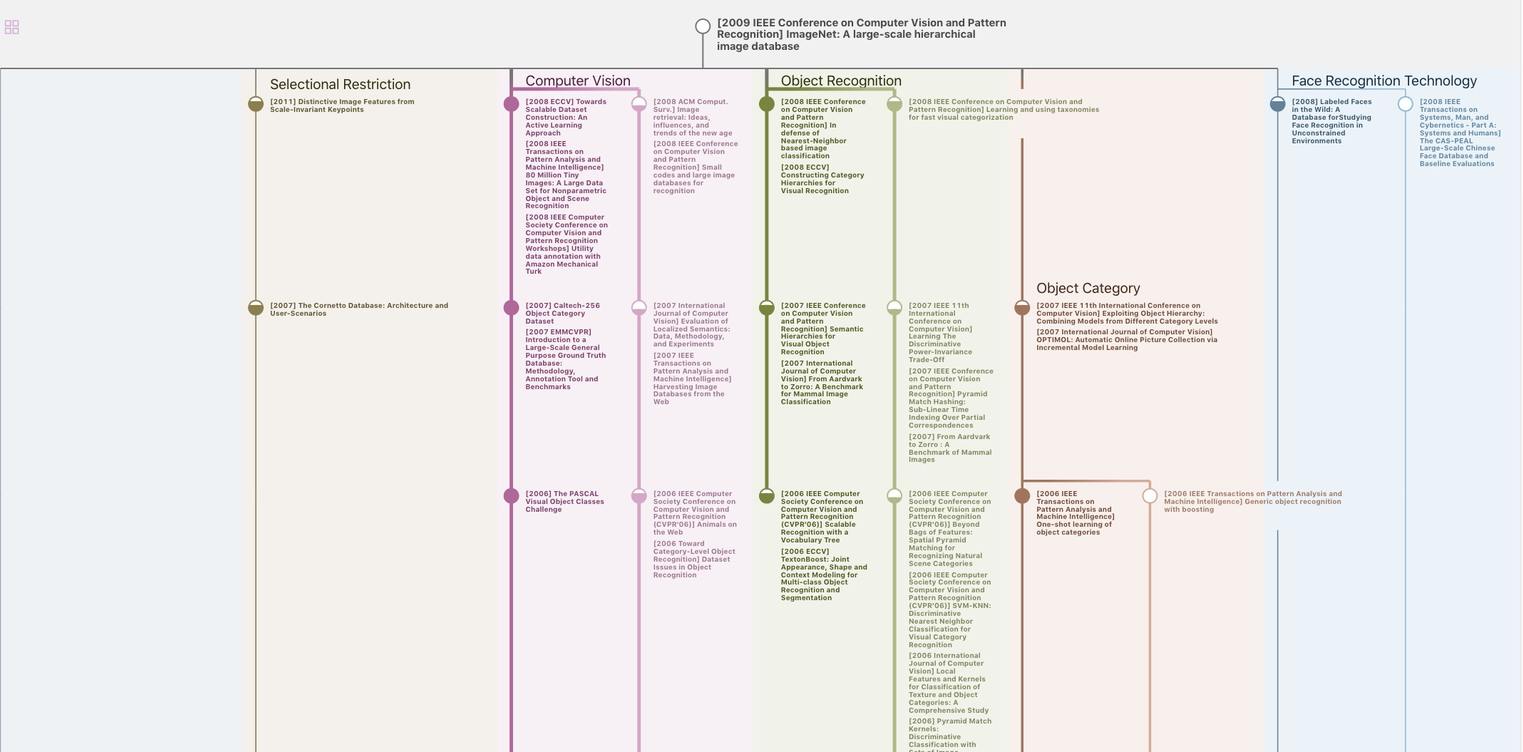
生成溯源树,研究论文发展脉络
Chat Paper
正在生成论文摘要