Can Agents Run Relay Race with Strangers? Generalization of RL to Out-of-Distribution Trajectories
ICLR 2023(2023)
摘要
In this paper, we evaluate and improve the generalization performance for rein-
forcement learning (RL) agents on the set of “controllable” states, where good
policies exist in these states to achieve high rewards. An RL agent that generally
masters a task should reach its goal starting from any controllable state of the
environment, without memorizing actions specialized for a small set of states. To
practically evaluate generalization performance in these states, we propose relay-
evaluation, involving starting the test agent from the middle of trajectories of other
independently trained, high-reward stranger agents. With extensive experimental
evaluation, we show the prevalence of generalization failure on controllable states
from stranger agents. For example, in the Humanoid environment, we observed that
a well-trained Proximal Policy Optimization (PPO) agent, with only 3.9% failure
rate during regular testing, failed on 81.6% of the states generated by well-trained
stranger PPO agents. To improve generalization, we propose a novel method called
Self-Trajectory Augmentation (STA), which does not rely on training multiple
agents and does not noticeably increase training costs. After applying STA to the
Soft Actor Critic’s (SAC) training procedure, we reduced the failure rate of SAC
under relay-evaluation by more than three times in most settings without impacting
agent performance and increasing the needed number of environment interactions.
更多查看译文
关键词
Genralization,Reinforcement Learning
AI 理解论文
溯源树
样例
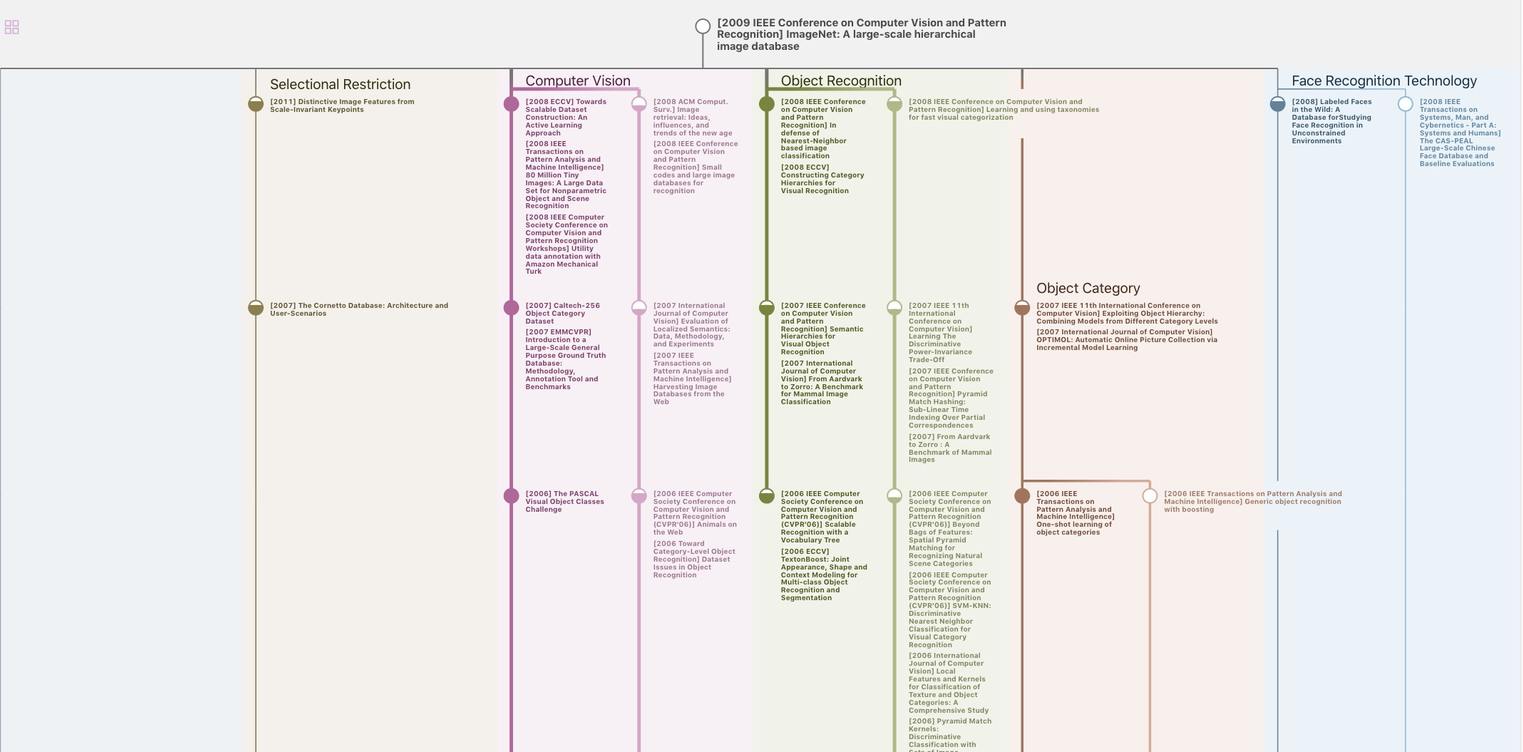
生成溯源树,研究论文发展脉络
Chat Paper
正在生成论文摘要