Mid-Vision Feedback for Convolutional Neural Networks
ICLR 2023(2023)
Abstract
Feedback plays a prominent role in biological vision, where perception is modulated based on agents' continuous interactions with the world, and evolving expectations and world model. We introduce a novel mechanism which modulates perception in Convolutional Neural Networks (CNNs) based on high level categorical expectations: Mid-Vision Feedback (MVF). MVF associates high level contexts with linear transformations. When a context is "expected" its associated linear transformation is applied over feature vectors in a mid level of a CNN. The result is that mid-level network representations are biased towards conformance with high level expectations, improving overall accuracy and contextual consistency. Additionally, during training mid-level feature vectors are biased through introduction of a loss term which increases the distance between feature vectors associated with different contexts. MVF is agnostic as to the source of contextual expectations, and can serve as a mechanism for top down integration of symbolic systems with deep vision architectures - applications range from image and video understanding to explainable AI and robotics. We show the superior performance of MVF to post-hoc filtering for incorporation of contextual knowledge, and show superior performance of configurations using predicted context (when no context is known a priori) over configurations with no context awareness.
MoreTranslated text
AI Read Science
Must-Reading Tree
Example
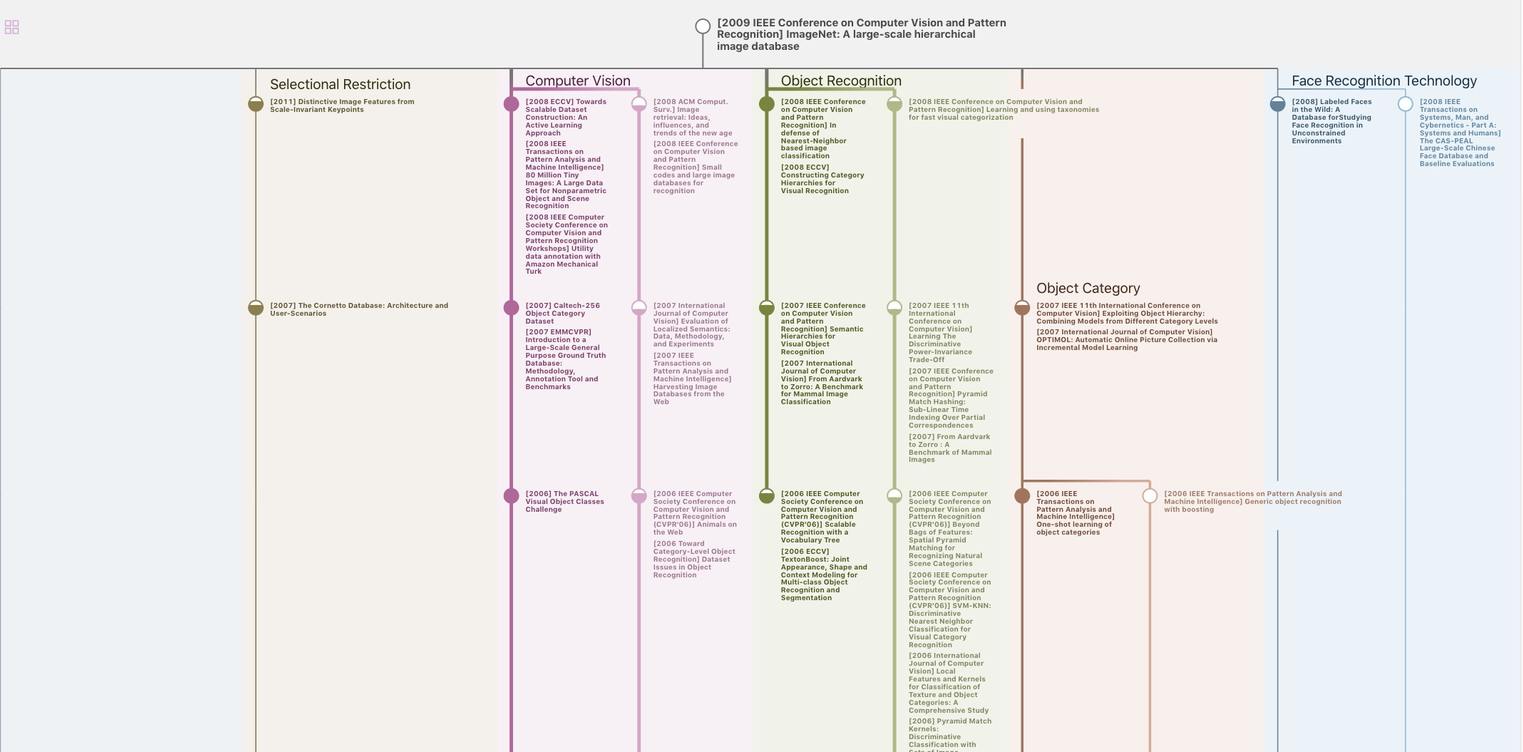
Generate MRT to find the research sequence of this paper
Chat Paper
Summary is being generated by the instructions you defined