Cross-Layer Retrospective Retrieving via Layer Attention
ICLR 2023(2023)
摘要
More and more evidence has shown that strengthening layer interactions can enhance the representation power of a deep neural network, while self-attention excels at learning interdependencies by retrieving query-activated information. Motivated by this, we devise a cross-layer attention mechanism, called multi-head recurrent layer attention (MRLA), that sends a query representation of the current layer to all previous layers to retrieve query-related information from different levels of receptive fields. A light-weighted version of MRLA is also proposed to reduce the quadratic computation cost. The proposed layer attention mechanism can enrich the representation power of many state-of-the-art vision networks, including CNNs and vision transformers. Its effectiveness has been extensively evaluated in image classification, object detection and instance segmentation tasks, where improvements can be consistently observed. For example, our MRLA can improve 1.6% Top-1 accuracy on ResNet-50, while only introducing 0.16M parameters and 0.07B FLOPs. Surprisingly, it can boost the performances by a large margin of 3-4% box AP and mask AP in dense prediction tasks.
更多查看译文
关键词
Layer Attention,Recurrent Layer Attention,Layer Interaction,CNNs,Vision Transformers,Vision Networks
AI 理解论文
溯源树
样例
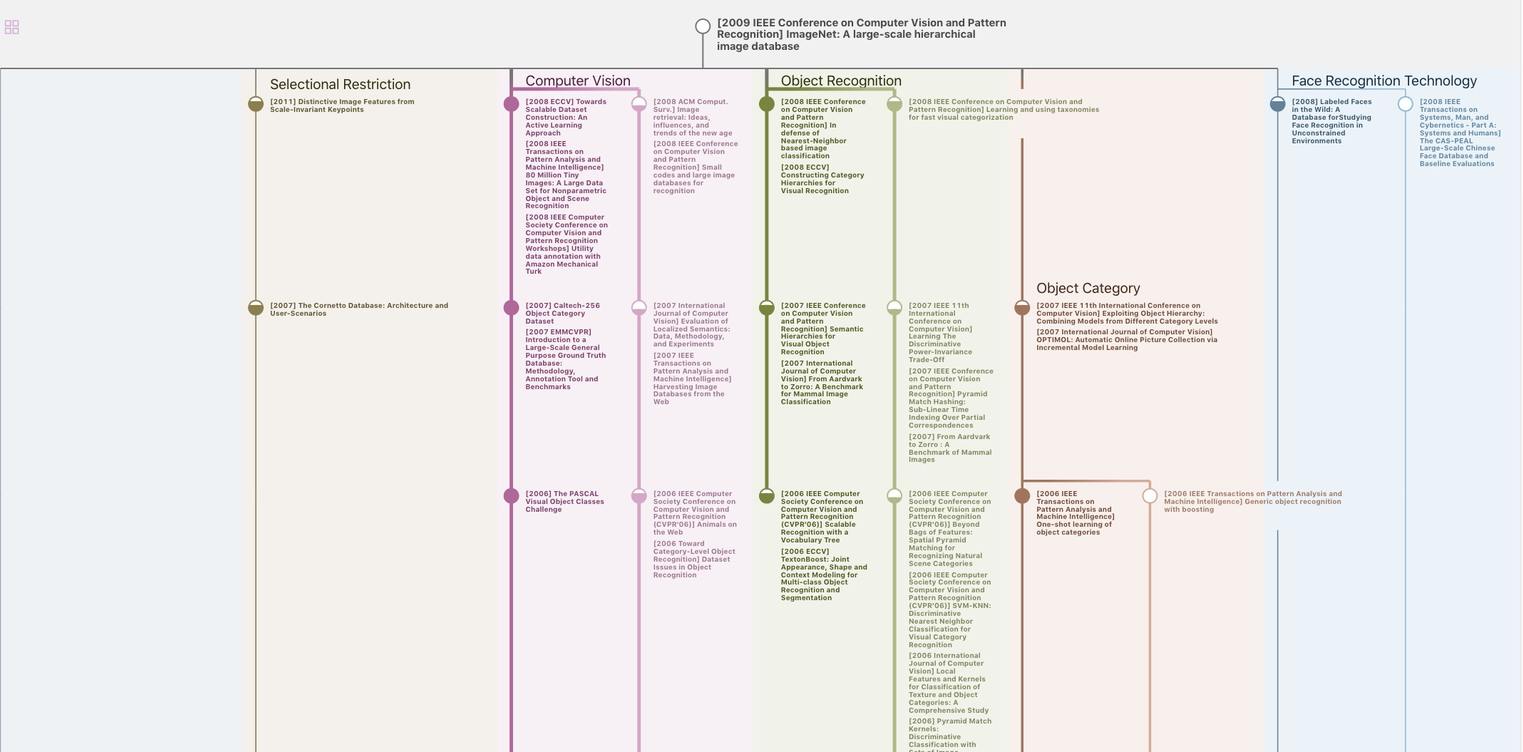
生成溯源树,研究论文发展脉络
Chat Paper
正在生成论文摘要