Softened Symbol Grounding for Neuro-symbolic Systems
ICLR 2023(2024)
摘要
Neuro-symbolic learning generally consists of two separated worlds, i.e.,
neural network training and symbolic constraint solving, whose success hinges
on symbol grounding, a fundamental problem in AI. This paper presents a novel,
softened symbol grounding process, bridging the gap between the two worlds, and
resulting in an effective and efficient neuro-symbolic learning framework.
Technically, the framework features (1) modeling of symbol solution states as a
Boltzmann distribution, which avoids expensive state searching and facilitates
mutually beneficial interactions between network training and symbolic
reasoning;(2) a new MCMC technique leveraging projection and SMT solvers, which
efficiently samples from disconnected symbol solution spaces; (3) an annealing
mechanism that can escape from
groundings. Experiments with three representative neuro symbolic learning tasks
demonstrate that, owining to its superior symbol grounding capability, our
framework successfully solves problems well beyond the frontier of the existing
proposals.
更多查看译文
关键词
neuro-symbolic learning,symbol grounding problem,projection-based sampling
AI 理解论文
溯源树
样例
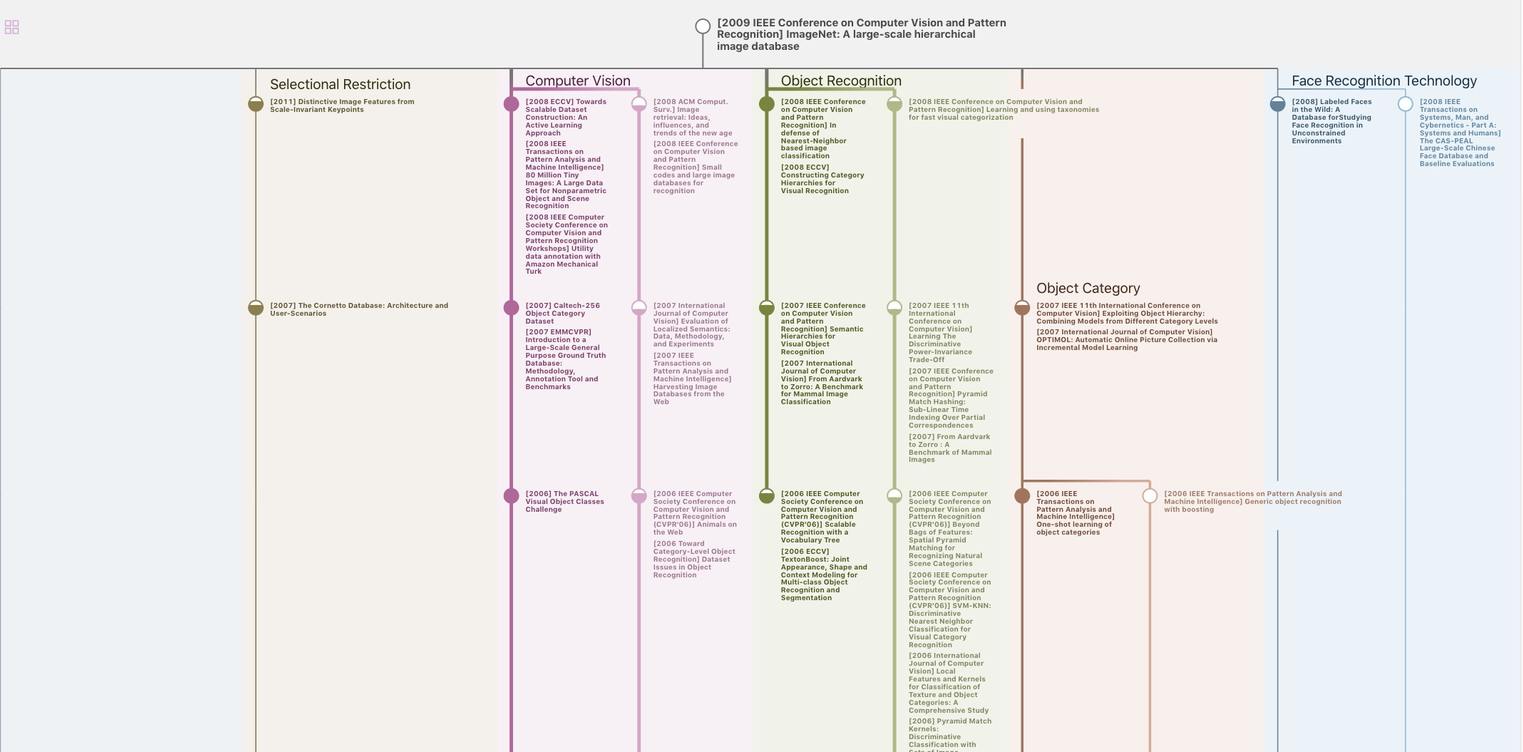
生成溯源树,研究论文发展脉络
Chat Paper
正在生成论文摘要